What is human pose estimation?
It is easy for a human to tell whether a person is squatting down or waving a hand. For a machine? Not so simple. When shown a photo or a video frame, a computer “sees” a collection of pixels.
Human pose estimation technology enables a computer to model human bodies and recognize body postures in images and videos, including real-time footage.
How does human pose estimation work?
Traditionally, human pose estimation relied on machine learning algorithms, specifically random forests. The essence of the ML-based approach boiled down to presenting a human body as a collection of parts arranged in a deformable structure, such as the one below.
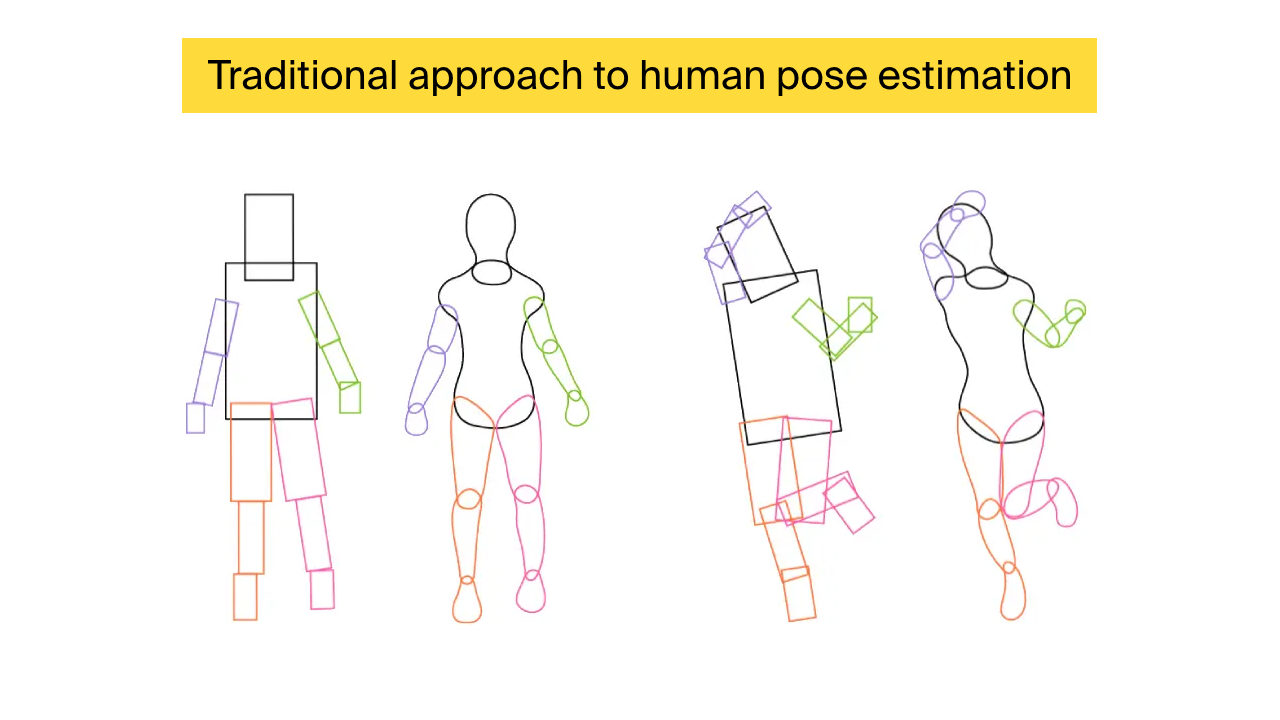
But the classical, ML-based method struggled to identify hidden body parts and failed at multi-person pose estimation. That has sparked the transition to deep learning that is used for human pose estimation today.
There are many ways to interpret a human posture with deep learning (hence, the many tools and libraries, which we explore in the subsequent section). Here’s how a typical deep learning model for human pose estimation runs.
A standard deep learning model for human pose estimation has a convolutional neural network (CNN) at its base.
The CNN detects keypoints in an input image, then maps the pre-estimated keypoints to the heatmap, and outputs those with the highest probability rate. It then groups the associated keypoints into a skeleton-like body structure.
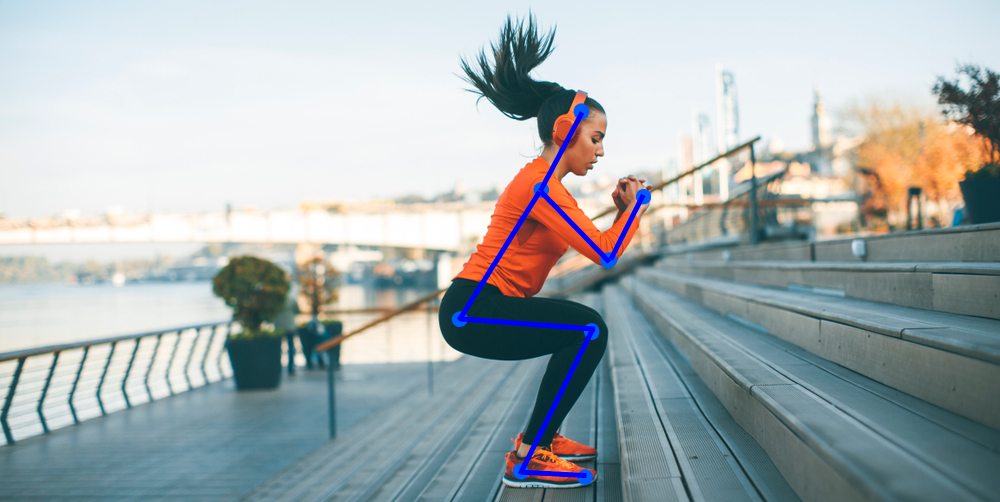
For multi-person estimation, when there are several high-probability areas for each keypoint, say, two left elbows, an additional post-processing layer may be added to ensure each keypoint belongs to the right person.
The method described above follows a bottom-up pipeline. It means that a CNN locates every instance of a particular keypoint first and then groups the associated ones into a body structure.
A model may follow a top-down approach, too. In this case, a CNN first locates all the bodies in an image, putting them into bounding boxes, and then detects the keypoints within each bounding box.
A rundown of essential human pose estimation models
Before we highlight the most popular deep learning-based human pose detection tools, it’s important to mention that not all pose detection models are designed for multi-person pose estimation. When choosing a pose estimation model, it’s essential to consider whether your application needs multi-person functionality. For instance, virtual try-ons and fitness tracking frequently only require single-person estimation, whereas crowd analysis and surveillance call for multi-person capability.
The most notable and widely used human pose estimation models
Multi-person pose recognition models
OpenPose
With its high accuracy and flexibility in a variety of settings and applications, OpenPose provides a reliable solution for multi-person real-time human pose estimation.
Architecture and technical features of OpenPose
-
Multi-stage CNN with probability maps and part affinity fields (PAFs)
OpenPose employs a two-part framework. First, it creates probability maps for each keypoint found in an image. Then it computes part affinity fields, which describe the direction and association between key points. These PAFs are vector fields that aid OpenPose in precisely associating keypoints to create a logical skeleton.
-
Hierarchical detection for body, hands, and face
Modular components of OpenPose are capable of separately detecting main body key points, hand gestures, and facial expressions. Such hierarchical detection structure enables a comprehensive analysis of pose, which is particularly useful for applications that need detailed tracking beyond simple body points.
-
Multi-person human pose estimation with cross-person key point association
OpenPose can accurately estimate and predict the posture of multiple individuals simultaneously. It ensures accuracy and consistency in complex, multi-person scenarios by using its affinity fields to individually associate each detected location with the appropriate person in crowded scenes.
-
Scalable and open-source architecture
Because OpenPose is extremely customizable and open-source, researchers and developers can modify the model to suit various tasks. It can be tailored for varied computational resources and is compatible with platforms like PyTorch and TensorFlow, which makes it adaptable to a range of deployment requirements.
Model applicability
OpenPose works well for activities that call for a high level of precision and flexibility in a variety of recording situations. These include applications requiring real-time precise tracking of hand, facial, and body posture; complicated and multi-person situations; and research and customizable applications where open-source, scalable solutions are required.
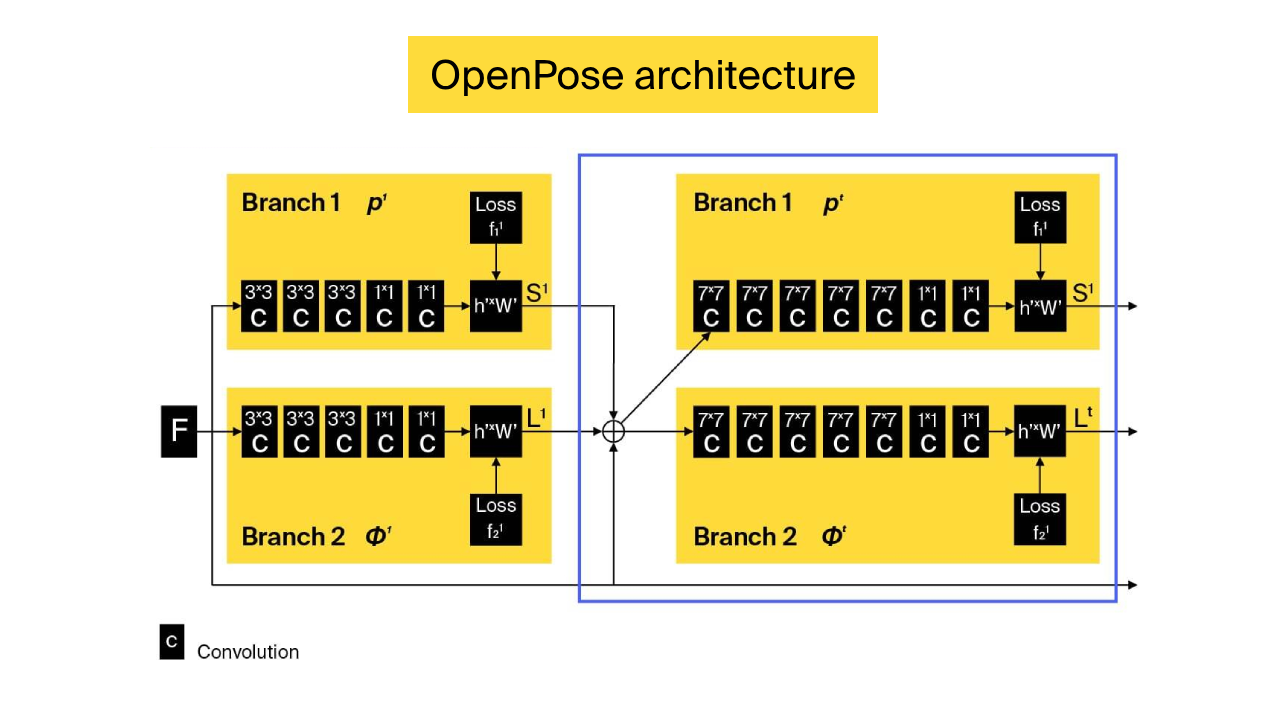
YOLOPose
Based on the YOLO (You Only Look Once) object detection framework, the model adapts YOLO’s single-pass approach to recognize human keypoints. This makes it fast and capable of pose recognition with little delay, which is particularly important for environments where real-time performance and minimal delay are priorities.
Architecture and features of YOLOPose
-
Single-pass detection
YOLOPose reduces latency and swiftly identifies keypoints by processing the full image in a single run. This allows the model to conduct human pose estimation in real-time.
-
Efficient keypoint localization
Rather than just identifying bounding boxes around people, YOLOPose employs YOLO’s feature extraction to locate specific body parts by assigning probability maps to each key point.
-
Optimized for low-resource devices
The lightweight architecture of YOLOPose is tailored to function well on devices with limited computational resources, such as embedded and mobile systems.
-
Cross-person keypoint association
Even in crowded surroundings, YOLOPose manages to associate keypoints with specific individuals, which makes it reliable for tracking multiple people without confusing detected poses.
Model applicability
YOLOPose works well in situations where simplicity and speed are key considerations, including scenarios that require quick system response with minimal delay. These include applications in gaming, surveillance, interactive media, and fitness tracking.
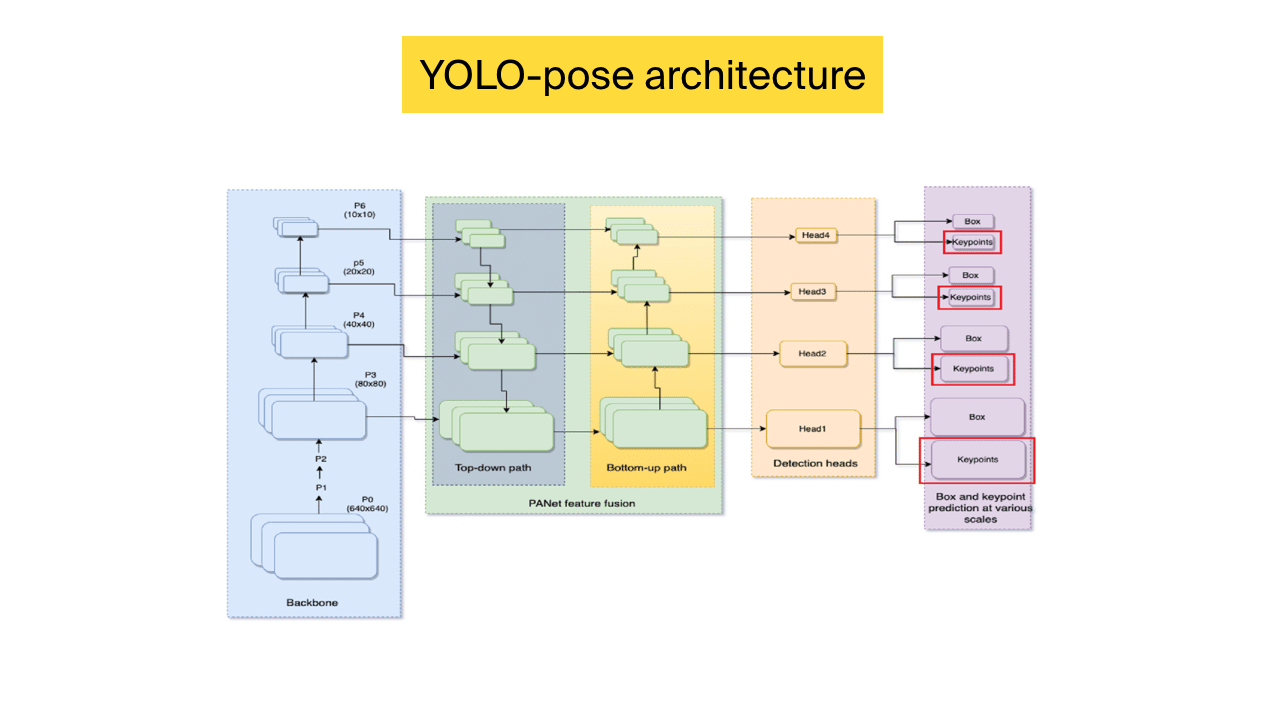
AlphaPose
AlphaPose is a popular model for high-precision human pose estimation designed to produce more accurate and robust pose estimations in complex, multi-person real-world conditions such as crowded environments and occlusions, where traditional methods may struggle to differentiate between individuals.
Architecture and technical features of AlphaPose
-
Two-stage detection and refinement process
AlphaPose uses a multi-stage pipeline. In the initial stage, it identifies distinct areas containing individuals, and then it fine-tunes the locations of keypoints inside each identified area. By concentrating on particular areas and decreasing false positives, this two-stage method improves accuracy and precision of keypoints.
-
Pose-guided proposals and keypoint matching
After using pose-guided proposals to aid in isolating keypoint locations, the model leverages a matching technique to link key points inside detected regions as efficiently as possible.
-
Integration with HRNet for enhanced accuracy
In order to maintain high spatial resolution in feature maps, AlphaPose frequently integrates with HRNet layers. Due to this integration, AlphaPose is able to record fine details, particularly in intricate poses, improving resilience to occlusion and spatial precision.
-
Robust multi-person tracking in dense scenes
The model is integrated with features that help identify and track people even when they are close to one another or partially concealed, which makes it capable of handling multi-person scenarios in densely crowded settings.
Model applicability
Its modules for handling occlusions, pose refinement, and real-time processing make this human pose detection model particularly useful in such applications as sports analysis, fitness tracking, and surveillance where robustness and precision are critical.
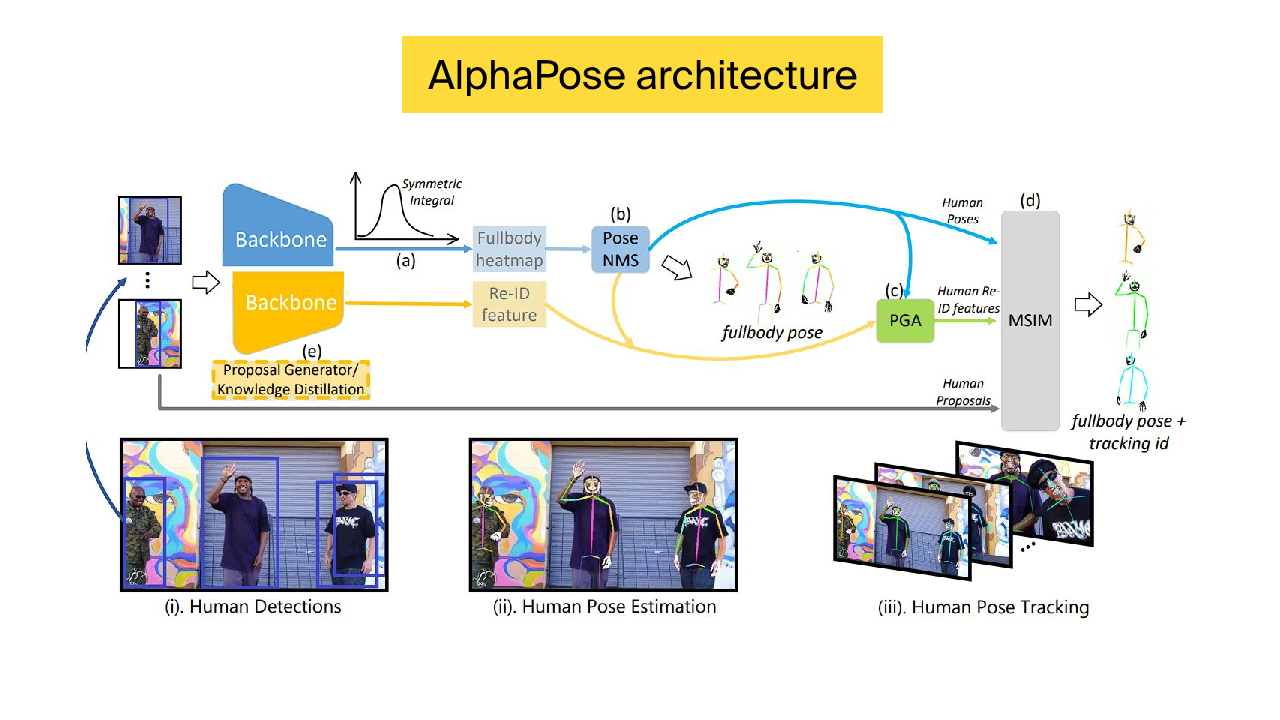
PoseNet
PoseNet is a lightweight architecture-based real-time human pose estimation system primarily designed for mobile applications. Its ability to estimate keypoints directly from images or video streams while balancing speed and accuracy makes it ideal for applications with limited computational resources.
Architecture and technical features of PoseNet
-
Lightweight convolutional architecture
Due to its lightweight convolutional architecture optimized for low latency, PoseNet can identify keypoints at a reduced computational cost, which makes it well-suited for mobile and web environments.
-
Keypoint detection via heatmaps
PoseNet operates by creating heatmaps for each key body point. The algorithm can precisely locate keypoints while adjusting to movement and body change in dynamic scenarios thanks to these heatmaps.
-
Single and multi-person support
Through the identification of unique heatmaps for each person in the frame, PoseNet can estimate poses for both single and multiple individuals.
-
Mobile and web-optimized with TensorFlow.js and TensorFlow Lite
PoseNet works with frameworks like TensorFlow.js and TensorFlow Lite, which are meant to facilitate real-time inference on web browsers and mobile devices. This allows PoseNet to execute pose estimation jobs on a variety of systems with minimal delay.
Model applicability
While not as accurate as other human pose detection models, PoseNet is highly useful in applications where speed and efficiency are more critical than precise keypoint localization.
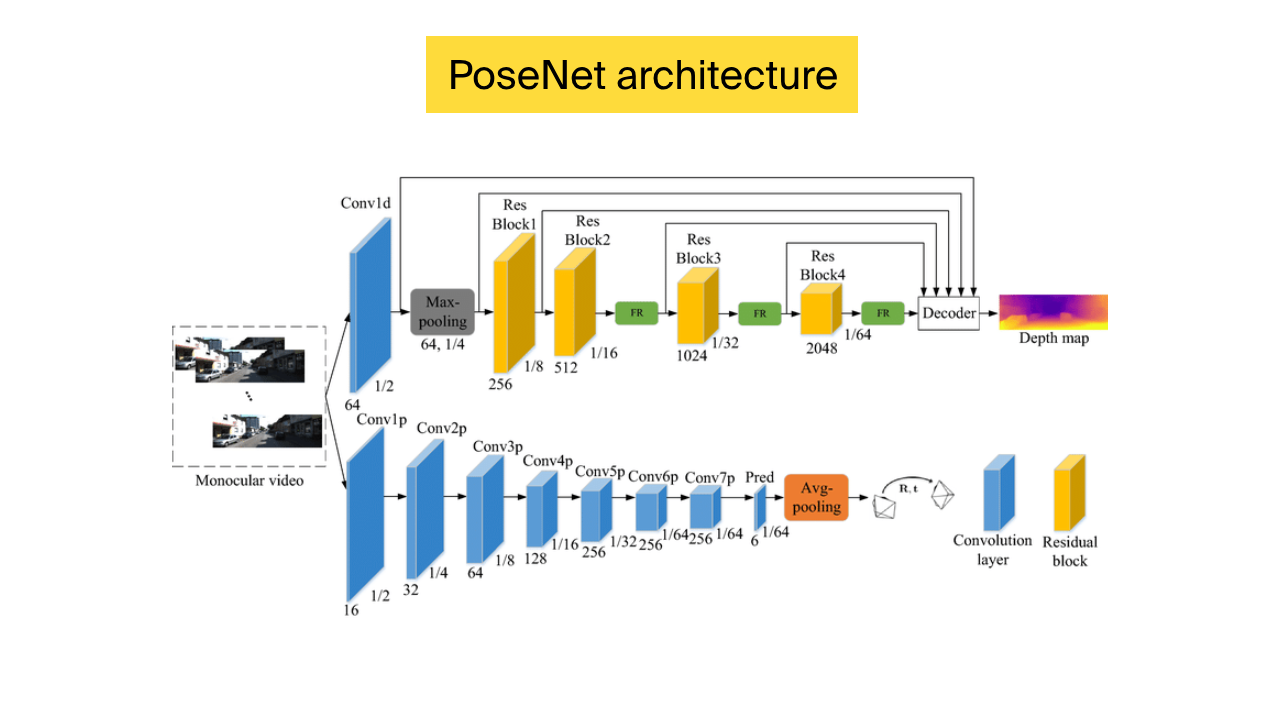
CenterNet
A powerful and effective model for object detection, CenterNet can also be modified for human pose estimation. By utilizing center-based detection and offset calculations, CenterNet provides a simplified approach to body pose estimation, enabling precise and effective pose tracking for a variety of real-time applications.
Architecture and features of CenterNet
-
Center-based keypoint detection
CenterNet determines the body center of each human and then utilizes it as the anchor for other keypoints that represent other body parts. This narrows the search space to the area around the center of each body, allowing for effective pose recognition.
-
Offset-based keypoint association
CenterNet identifies individual keypoints on the body and assigns them to specific body parts by calculating the offset from the central keypoint. By associating each keypoint with the appropriate object, this offset technique ensures accurate multi-person pose estimation.
-
Single-stage network
Functioning as a single-stage network, CenterNet processes images in a single forward pass.
-
Multi-person and occlusion handling
Because each detected center serves as an independent anchor for keypoint identification, CenterNet can handle occlusions and complex scenes and properly recognize multiple people’s poses at once.
Model applicability
CenterNet is especially well-suited for tasks that need real-time, accurate, and efficient pose estimation. These include high-performance applications that require low latency and efficient processing, such as video analytics and live monitoring; dynamic and multi-person scenarios; and challenging environments with partial occlusions.
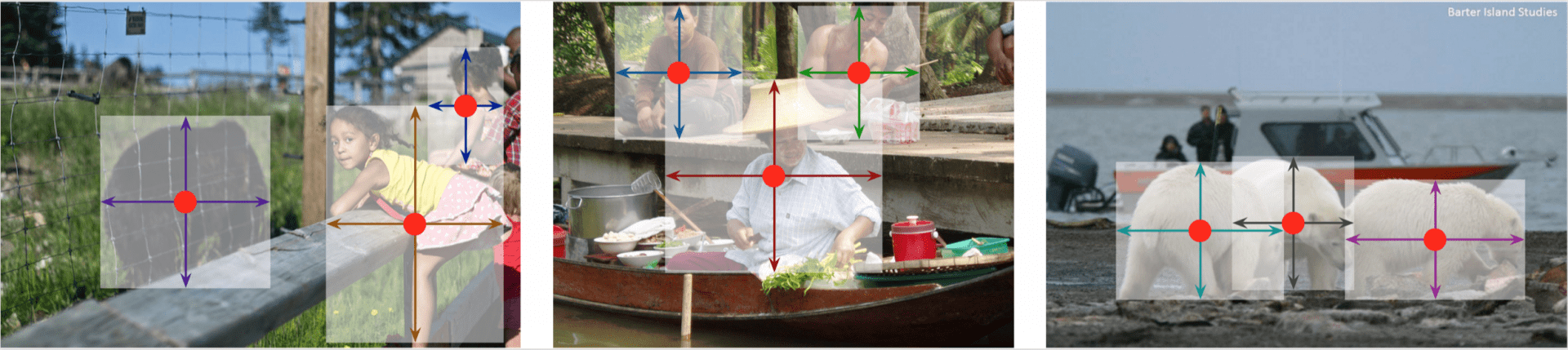
Single-person pose recognition models
BlazePoze
BlazePose is a high-performance body pose estimation model tailored for mobile real-time applications. It enables quick and precise human pose estimation by using a lightweight convolutional neural network architecture to identify 33 key body points from a single picture or video frame.
Key features of BlazePose
-
Two-stage detector-tracker pipeline
BlazePose employs a two-stage procedure in which an initial detector identifies the area of interest where the individual is located, and a second tracker forecasts the exact positions of keypoints within this area.
-
3D pose estimation capability
BlazePose can infer 3D coordinates in addition to 2D key point recognition, offering a thorough analysis of body movements in three dimensions.
-
Mobile device optimization
BlazePose was created with mobile platforms in mind and offers excellent performance without consuming a lot of energy.
Model applicability
BlazePose is particularly beneficial for tasks requiring immediate pose recognition, such as fitness tracking and augmented reality applications. Besides, it works well for applications requiring spatial analysis of poses.
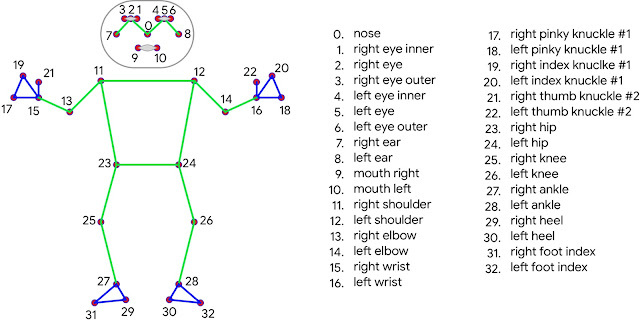
MoveNet
MoveNet is a highly efficient human pose estimation model especially designed for real-time applications on mobile and edge devices. A precise and low-latency tracking of human positions is made possible by MoveNet’s simplified architecture, which can identify 17 keypoints on the human body.
Architecture and features of MoveNet
-
Single-pass detection
MoveNet is a single-stage, single-pass model designed to identify body keypoints in real-time.
-
High-precision key point localization
The major joints and extremities, including the shoulders, elbows, wrists, hips, knees, and ankles, are among the 17 essential keypoints on the human body that MoveNet can detect.
-
Two variants: MoveNet Lightning and MoveNet Thunder
MoveNet Lightning is developed for high-speed inference on mobile and low-power devices, delivering rapid posture estimation with minimal resource utilization. MoveNet Thunder is appropriate for more complicated environments since it offers greater accuracy and is tailored for applications that value accuracy over speed.
-
Robustness in fast-moving and dynamic scenes
MoveNet shows a high level of accuracy and stability even in difficult settings with fast-moving individuals or a changing background.
Model applicability
MoveNet is perfect for applications that need quick and precise human pose estimation in a variety of conditions. These include real-time applications on mobile and embedded devices, scenarios with high-speed movements, and fitness, sports, and activity monitoring applications.
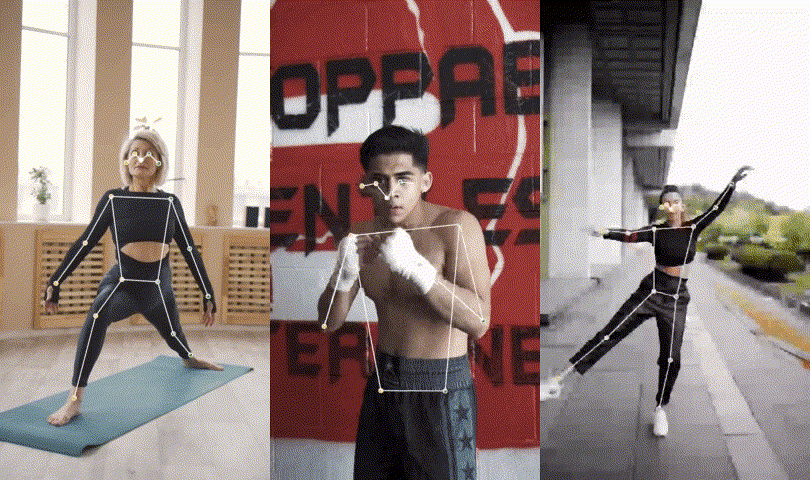
Where is human pose estimation applied?
Recognizing human posture and movements has long been in focus for major industries, including sports, retail, and entertainment. Here’s a run-through of sectors where human posture estimation is in use.
Fitness
The pandemic has pushed more people to practice physical activities at home, making the demand for fitness apps with human pose estimation grow rapidly. HPE-powered fitness applications provide detailed live feedback on whether a user performs an exercise correctly. For that, a human pose estimation component compares a model extracted from camera footage with a benchmark, thus providing for a safer home workout routine. Fitness apps featuring human pose estimation cater to various activities, from yoga to dancing to weight lifting.
If you are developing fitness apps, there are available solutions that you can integrate into your application. For example, MotionMind offers powerful pose estimation capabilities, which can be used in fitness, public safety, healthcare, and many other industries.
Professional sports
Human pose estimation technology can help athletes improve their performance and assist judges in rating athletes unbiasedly. HPE-powered applications are applied for various tasks—from assessing the quality of figure skating elements to helping soccer players strike perfect kicks to allowing high jumpers to polish up their techniques.
Gaming and filmmaking
Character animation has long been an exhaustive and complex process. Today, it is facilitated with the help of human pose estimation. Graphics, textures, and other enhancements can now be easily applied to a tracked body, so the graphics render naturally even if the body actively moves.
In interactive video gaming, human pose estimation is used to capture players’ motions and render them into the actions of virtual characters as well.
Retail
Whether trying to curb the effect of the pandemic or realize their vision of a supermarket of the future, retailers have started turning to AR and real-time virtual effects. Human pose estimation backs up those aspirations, enabling such experiences as virtual try-on and real-time marketing. An HPE-powered app, whether running on a customer’s mobile phone or integrated into a fitting room’s mirror, allows scanning a person’s body and imposing 3D virtual elements on the estimated posture. And that works for trying out everything from clothes to shoes to jewelry.
Robot training
Traditionally, industrial robots were trained with the help of 2D vision systems that called for time- and effort-intensive calibration. Today, human pose estimation allows for faster, more responsive, and more accurate robot training. Instead of programming robots to follow the set trajectories, one may teach a robot to recognize the pose and the motions of a human. Having estimated the posture of the demonstrator, a robot then devises the way it should move its articulators to perform the same motion.
Security and surveillance
Human pose estimation may be applied to analyze the footage from security cameras to prevent potentially alarming situations. Identifying a human posture and estimating its anomaly score, HPE-powered security software may predict suspicious actions or identify people who have fallen down or, say, potentially feel sick.
Implementing human pose recognition: the peculiarities to keep in mind
ITRex Group has recently helped a fitness tech startup create a fitness mirror powered by artificial intelligence and human pose estimation. We sat down to talk to Kirill Stashevsky, the ITRex CTO, to discuss the specifics of implementing human pose estimation technology that contribute a lot to the project’s success but are often overlooked.
— How does one embark on the HPE implementation journey to ensure they produce a top-notch solution? What should one beware of during project planning to secure that further development efforts are headed in the right direction?
Kirill: The decisions you make at the start of the project will have a significant impact on whether or not you create a successful human pose estimation product. One such decision is selecting the optimum implementation strategy—i.e., developing a HPE solution from scratch or using one of the many human pose estimation libraries.
To choose the best-fitting approach, you need to clearly understand, among other issues, what exactly you aim to achieve with your future product, which platforms it will run on, and how much time you have until releasing your product to the market. Once you’ve clarified the vision, weigh it against the available strategies.
Consider going the custom route if the task you are solving is narrow and non-trivial and requires the ultimate accuracy of human pose estimation. Keep in mind, however, that the development process is likely to be time- and effort-intensive.
In turn, if you are developing a product with a mass-market appeal or a product that caters to a typical use case, going for library-based development would help build a quality prototype faster and with less effort. Still, in many cases, you would have to adjust the given model to your specific use case by further training it on the data that best represents real-world scenarios.
— Suppose I decide to go for library-based development; what factors should I consider to choose the right one?
Kirill: You may go for a proprietary or an open-source library. Proprietary libraries could ensure more accurate pose estimation and require less customization. But you have to prepare a backup plan in case the vendor, say, discontinues the library support.
Open-source libraries, in turn, often require more effort to configure. But with an experienced team, it may be an optimum option balancing the quality of recognition, moderate development costs, and fair time-to-market.
Pay attention to the number of keypoints a library is able to recognize, too. A solution for dancers or yogis, for instance, may require identifying additional keypoints for hands and feet so that BlazePose might look like a more reasonable option. If latency is critical, select the library that runs at an FPS rate of 30 and higher, for example, MoveNet.
— Why aren’t the standard datasets lying at the base of most models enough for an accurately performing solution? What data should I then use to further train and test the model?
Kirill: A well-performing human pose estimation model should be trained on the data that is authentic and representative of reality. The truth is that even the most expansive datasets often lack diversity and fail to yield reliable outcomes in real-life settings. That’s what we faced when developing the human pose estimation component for our client’s fitness mirror.
To mitigate the issue, we had to retrain the model on additional video footage filmed specifically to reflect the surroundings the mirror will be used in. So, we compiled a custom dataset of videos featuring people of different heights, body types, and skin colors exercising in various settings—from poorly lit rooms to spacious fitness studios—filmed at a particular angle. That helped us significantly increase the accuracy of the model.
— Are there any other easy-to-overlook issues that still influence the accuracy of human pose estimation?
Kirill: Focusing on the innards of deep learning, development teams may fail to pay due attention to the cameras. So, make sure that the features of a camera (including its positioning, frame size, a frame rate, a shooting angle, as well as whether the camera is shooting statically or dynamically) used for filming training data match those of cameras real users might employ.
Let's recap
So, you are considering implementing a solution with human pose estimation features. Here are vital things to keep in mind to develop a winning application:
-
Make sure that the strategy you choose to go by supports your vision. Go custom if you’re developing a solution for a specific, non-trivial task. Alternatively, consider implementing your future solution based on a readily available library — either open-source or proprietary
-
When choosing an optimum human pose estimation library, pay particular attention to the number of keypoints the offered model can recognize and the processing speed, especially if your future application is latency-critical
-
Whether you decide to develop a human pose estimation application from scratch or opt for library-based development, you will have to train a model further to guarantee it performs accurately
-
When training the model, use data that is as diverse and representative of reality as possible
-
At the very start of the project, make sure you have enough data to test the model