Top 5 AI challenges & tips to navigate them
As a technology company that jumped on the AI bandwagon before it became mainstream, we’ve seen our share of challenged AI projects.
More often than not, artificial intelligence problems stem from a misunderstanding of what AI is, what it is capable of, and whether its implementation makes sense in particular situations.
For example, ever since ChatGPT and other foundation AI models came into prominence, enterprises have been willing to explore generative AI services. Guess how many companies lack an infrastructure for integrating the technology into their processes — and quality data for AI model training.
Essentially, we could boil AI challenges down to five critical issues:
-
Encountering technology-related problems in the development process
-
Failing to reproduce lab results in the real world
-
Struggling to scale AI systems across use cases
-
Making erroneous assumptions about AI capabilities
-
Solving the ethical challenges of AI adoption
AI challenge #1: Hitting technology roadblocks
While AI has been around since the mid-50s, AI-powered chatbots, face swap apps, and robot dogs only became a viable reality a couple of years ago.
As of now, neither businesses nor their technology partners have a tried-and-true formula for developing and deploying AI systems company wide.
Some of the common AI pitfalls include:
-
Poor architecture choices. Making accurate predictions is not the only thing you should expect from an AI solution. In multi-tenant AI as a service (AIaaS) applications serving thousands of users, performance, scalability, and effortless management are equally important. You cannot expect your vendor to simply write a Flask service, package it in a Docker container, and deploy your ML model. When the system reaches its maximum capacity, you’ll be left with an app that is too big and complex to manage effectively.
-
Inaccurate or insufficient training data. AI systems’ performance depends on the quality of the data they have been trained on. In some cases, companies struggle to provide quality data (and a substantial volume thereof!) to train AI algorithms. The situation is not uncommon in healthcare, where patient data like X-ray images and CT scans is hard to obtain due to privacy reasons. To better identify and understand recurring patterns in input data, it is also crucial to manually label training datasets using annotation tools like Supervise.ly. According to Gartner, data-related AI problems were the #1 reason for 85% of artificial intelligence projects delivering erroneous results through 2022.
-
Lack of AI explainability. Explainable artificial intelligence (XAI) is a concept that revolves around providing enough data to clarify how AI systems come to their decisions. Powered by white-box algorithms, XAI-compliant solutions deliver results that can be interpreted by both developers and subject matter experts. Ensuring AI explainability is critical across a variety of industries where smart systems are used. For example, a person operating injection molding machines at a plastic factory should be able to comprehend why the novel predictive maintenance system recommends running the machine in a certain way — and reverse bad decisions. Compared to black-box models like neural networks and complicated ensembles, however, white-box AI models may lack accuracy and predictive capacity, which somewhat undermines the whole notion of artificial intelligence.
Solution
To avoid technology-related artificial intelligence challenges, we recommend that you start your artificial intelligence project with a discovery phase and create an AI proof of concept.
This would allow you to map the solution requirements against your business needs, eliminate technology barriers, and plan the system architecture with the anticipated number of users in mind.
It is also essential to select a technology partner who knows how to overcome the data-related challenges of artificial intelligence — for instance, by reusing existing algorithms or deliberately expanding the size of a training dataset.
When it comes to the accuracy vs. explainability trade-off, the vendor of your choice should have hands-on experience working with LIME and surrogate models, which represent the decision-making process of sophisticated AI systems.
AI challenge #2: Replicating lab results in real-life situations
An AI-based breast cancer scanning system created by Google Health and Imperial College London reportedly delivers fewer false-positive results than two certified radiologists.
In 2016, Oxford and Google DeepMind scientists developed a deep neural network that reads people’s lips with 93% accuracy (compared to just 52% scored by humans).
And now there’s evidence that machine learning models can accurately detect COVID-19 in asymptomatic patients based on a cellphone-recorded cough!
When fueled by powerful hardware and a wealth of training data, AI algorithms can perform a wide range of tasks on a par with humans — and even outmatch them. The problem with AI is, most companies fail to replicate the results achieved by Google, Microsoft, and MIT — or the accuracy displayed by their own AI prototypes — outside the laboratory walls.
Solution
The solution to this daunting AI challenge partially lies in tech giants’ willingness to share complete research findings and source code with fellow scientists and AI developers.
On a company level, there are several things you could do to replicate the results delivered by AI solutions in a controlled environment:
-
Understand the constraints, variations, and unpredictability of the real world that can affect the performance of the AI system
-
Ensure that the training data used for the AI system is diverse and representative of the real-world scenarios it will encounter
-
Use data augmentation techniques like adding noise, introducing variations, or simulating different scenarios to artificially increase the diversity of your training data
-
Utilize transfer learning by initially pre-training your AI model on a vast, comprehensive dataset, and subsequently refine it by fine-tuning with domain-specific data
-
Configure your AI systems to update and retrain themselves with new real-world data to stay relevant and accurate
-
Establish feedback loops with real-world users or operators of the AI system, who will collect feedback on its performance and use the insights to adjust the model and its predictions
-
Implement monitoring and debugging tools to identify and address issues that arise in real-world settings, such as model drift, AI bias, and gradual performance degradation
If your in-house IT team lacks the necessary skills and expertise to perform these activities, you can enlist the help of experienced ML consultants.
AI challenge #3: Scaling artificial intelligence
Software scalability issues haunt various IT projects regardless of their technology stack, and AI solutions are no exception,
According to Gartner, just 53% of AI projects successfully transition from prototypes to production. This statistic indicates a lack of technical expertise, competencies, and resources needed to deploy intelligent systems at a large scale.
Other factors behind AI scalability challenges include:
-
The size of data sets for algorithm training and the quality of their data
-
The utilization of significant computing resources for AI model training and deployment
-
The increasing complexity of present-day AI models
-
The need to expand the underlying cloud infrastructure horizontally or vertically to accommodate for the AI model evolution and implementation across multiple use cases
-
The necessity to integrate AI models with other systems within a company’s IT infrastructure
Solution
Continuous knowledge transfer might be a viable solution to the AI scalability problems.
While most companies currently rely on third-party vendors to build smart systems and put them to work, forward-thinking CIOs and IT leaders must ensure their pilot projects help transfer knowledge from external DevOps, MLOps, and DataOps specialists.
Equipped with this knowledge, you can take several steps to address AI scalability challenges:
-
Assess your AI infrastructure to identify any potential bottlenecks and establish clear objectives for scaling the system
-
Implement efficient data management practices, including data cleaning, pre-processing, and storage optimization, and maintain sufficient data quality as your datasets expand
-
Invest in model optimization techniques to reduce their size and complexity and improve their resource efficiency
-
Adopt distributed computing frameworks like Apache Spark or TensorFlow for distributed training and inference
-
Utilize cloud computing platforms that offer scalability and elasticity
-
Explore containerization and orchestration tools like Kubernetes for effective resource management
-
Enhance your cloud deployments with fog and edge computing capabilities to reduce latency and server load
-
Tap into anomaly detection techniques to detect AI model performance issues
-
Implement specialized hardware accelerators like GPUs, TPUs, or FPGA to speed up AI workloads
AI challenge #4: Overestimating AI’s power
Back in 2020, MIT Sloan Management Review and Boston Consulting Group released a report that provided insights into why certain companies reap the benefits of AI while others do not.
DHL, a postal and logistics company that delivers 1.5 billion parcels a year, is among the AI winners.
The company uses a computer vision system to determine whether shipping pallets can be stacked together and optimize space in cargo planes.
Gina Chung, VP of innovation at DHL, says the cyber-physical system performed poorly in its early days. Once it started learning from human experts who had years of experience detecting non-stackable pallets, the results improved dramatically.
In business settings, such a balanced approach to AI implementation is rather an exception than a rule.
In reality, many companies are influenced by the media hype around AI and begin ambitious projects without adequately assessing their needs, IT capabilities, AI development costs, and the legal and ethical implications of the technology.
Solution
If complete automation and reduction in your company’s headcount lie at the heart of your AI strategy, you are likely to fail.
For one thing, algorithms need human knowledge to eventually make accurate predictions.
And for another, your employees will feel more enthusiastic about teaching algorithms if you make it clear smart machines won’t replace the human workforce in the foreseeable future.
To prevent overestimation of AI’s capabilities, wrap your head around potential AI benefits and current constraints — and here’s where our eBook may come in handy!

AI challenge #5: Dealing with AI ethical issues
Greater adoption of smart applications comes along with several AI ethical challenges, including:
-
Bias in algorithmic decision making, which stems from flawed training data prepared by human engineers and bears the mark of social and historical inequities. A facial recognition system deployed by US law enforcement agencies, for instance, is more likely to identify a non-white person as a criminal.
-
Moral implications, which mainly revolve around some companies’ intent to replace human workers with highly productive, always-on robots. Even though two-thirds of business executives believe AI will eventually create more jobs than it’s going to kill, 69% of organizations might need different skills to strive in the digital era.
-
Limited transparency and explainability, which is typical of advanced black-box AI solutions. Not only do deep learning networks fail to explain the reasoning behind their decisions, but it’s also challenging to determine accountability for AI recommendations in case of system errors and inflicted harm.
Solution
Your company can solve most ethical artificial intelligence problems by creating balanced training datasets that include images of people representing different ethnic, gender, and age groups.
In fact, artificial intelligence can help us eliminate racial, gender, age, and sexual orientation bias in the long run. For example, AI-powered HR management software can scan more resumes than human specialists and identify potential candidates based solely on their education and working experience.
Other steps you could take to navigate AI challenges include developing a set of ethical guidelines and principles. These guidelines should reflect your company’s commitment to fairness, transparency, privacy, and accountability.
As part of this strategy, you should also conduct regular ethical audits of AI systems to identify and address potential ethical issues.
Lastly, consider offering training programs for employees involved in AI development and deployment to raise awareness of ethical issues and best practices.
How to overcome AI challenges: take-home message
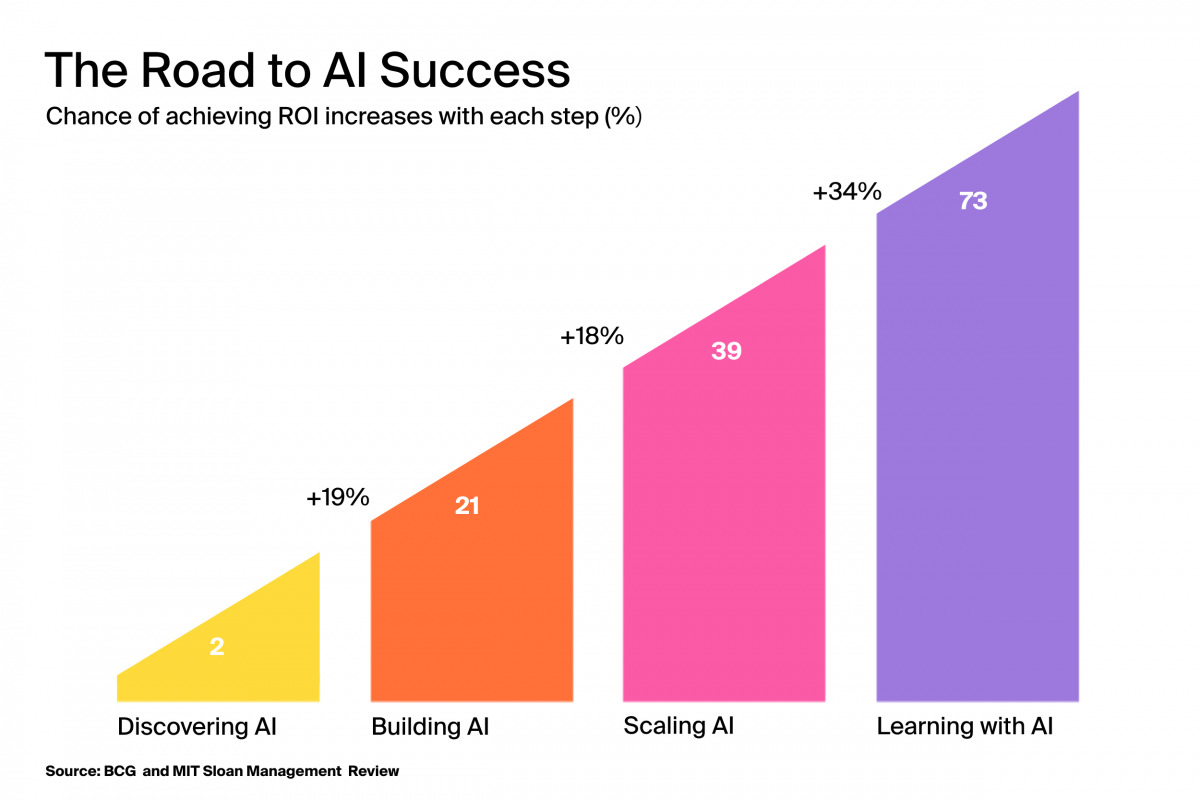
Going back to the BCG and the MIT Sloan Management Review report we referenced earlier, it’s worth noting that your chances of solving AI challenges successfully increase with every step in your journey.
Below you will find a high-level plan for ideating, validating, developing, implementing, and scaling AI systems in a risk-free manner:
-
Address an AI vendor with the relevant portfolio and expertise
-
Work with a skilled business analyst to determine which of your processes and IT systems could benefit from AI
-
Consider how ethical issues might prevent you from using AI to the fullest
-
Create a proof of concept to test the solution’s feasibility and work around technology-related AI pitfalls
-
Devise a detailed AI project implementation map covering solution development, integration, and scaling, as well as employee onboarding
-
Together with your vendor, start building your system while ensuring continuous knowledge sharing
-
Do not raise your hopes high: it takes time, patience, and lots of data to build AI solutions capable of enhancing or taking over critical tasks
-
Appoint subject matter experts to fine-tune AI algorithms
-
Educate your employees about the importance of data-driven decision making and optimization opportunities offered by artificial intelligence
Last but not least, continue experimenting with AI — even if your pilot project does not deliver on its promise! 73% of companies that overhaul their processes based on the lessons learned from failures eventually see a sizable ROI on their artificial intelligence investments.