Exploring the four fundamental types of AI: from reactive to self-aware systems
Artificial intelligence is an umbrella term that defines smart algorithms capable of applying human-like reasoning to make decisions autonomously and perform miscellaneous activities.
The most rational way to classify artificial intelligence would be to compare algorithms’ cognitive abilities to those of a person.
This approach allows us to differentiate between four types of AI:
-
Reactive machines
This type of AI is intended to perform specific tasks, such as playing chess or converting handwritten messages into editable text. Such algorithms neither possess memory nor predict the future; instead, they merely respond to a current situation. An excellent example of a reactive machine is the IBM Deep Blue supercomputer that narrowly beat Garry Kasparov, a reigning world chess champion, back in 1997. It reportedly took IBM scientists 12 years to create algorithms that could explore up to 200 million chess positions per second. However, Deep Blue lacked the ability to learn from previous games and perform well outside of chess.
-
Limited memory machines
When making decisions, limited memory AI algorithms consider both the information they’re gathering from the outside world in real time and the data they’ve been trained on. That’s how autonomous vehicles maneuver around objects and obey speed limits. Foundation models like GPT and DALL·E that power generative AI solutions belong to the limited memory machines category, too. Even though Gen AI use cases range from responding to customer emails to identifying trends in unstructured data, the technology shares limitations with other types of artificial intelligence from the limited memory machine category, such as being constrained by training data and lacking self-awareness.
-
Theory of Mind (ToM)
Scientists working on Theory of Mind artificial intelligence hope to develop algorithms with cognitive and social skills that can detect people’s emotions from video data, voice recordings, or text messages. Unlike the first two types of artificial intelligence discussed in this article, ToM solutions in their traditional sense do not yet exist. DeepMind’s ToMnet, a computer program that uses three neural networks to anticipate other agents’ needs, is perhaps the closest we’ve got to Theory of Mind so far. Yet again, such systems largely use statistical shortcuts to solve problems instead of acting on their own accord.
-
Self-aware AI
Such algorithms acknowledge their existence. Aside from interpreting others’ feelings, they can form their own beliefs and desires, including a sense of self-preservation, which could lead to a Skynet scenario in the not-too-distant future. Luckily for us, self-aware artificial intelligence remains a sci-fi concept.
It should be noted that this classification is neither rigid nor exhaustive, as the field of AI is constantly evolving. Many AI systems may not neatly fit into one of these categories or may exhibit traits from multiple categories. As technology advances, new classifications of AI types may emerge to better describe the current state of artificial intelligence capabilities.
For example, some experts tend to divide AI systems into two categories: narrow artificial intelligence (ANI) and general artificial intelligence (AGI).
Let’s see how these types of artificial intelligence stack up against each other:
-
The former category includes applications that outperform humans in certain narrowly defined tasks, such as detecting malicious tumors in mammograms. The amount and quality of data fed into ANI solutions determine their cognitive abilities. When faced with an unfamiliar task or a new dataset, these systems may deliver inaccurate results.
-
AGI solutions, on the other hand, try to mimic human intelligence by adapting their abilities to different contexts. Theory of Mind and self-aware AI systems belong to this type of artificial intelligence.
In-depth analysis of AI subsets: machine learning, neural networks, and beyond
As we explained in the previous section, artificial intelligence is a broad term that refers to machines’ ability to perform tasks previously executed solely by humans.
This term is often used interchangeably with other buzzwords like machine learning, reinforced learning, and deep learning, particularly in the business context.
Let us investigate what is wrong with this approach.
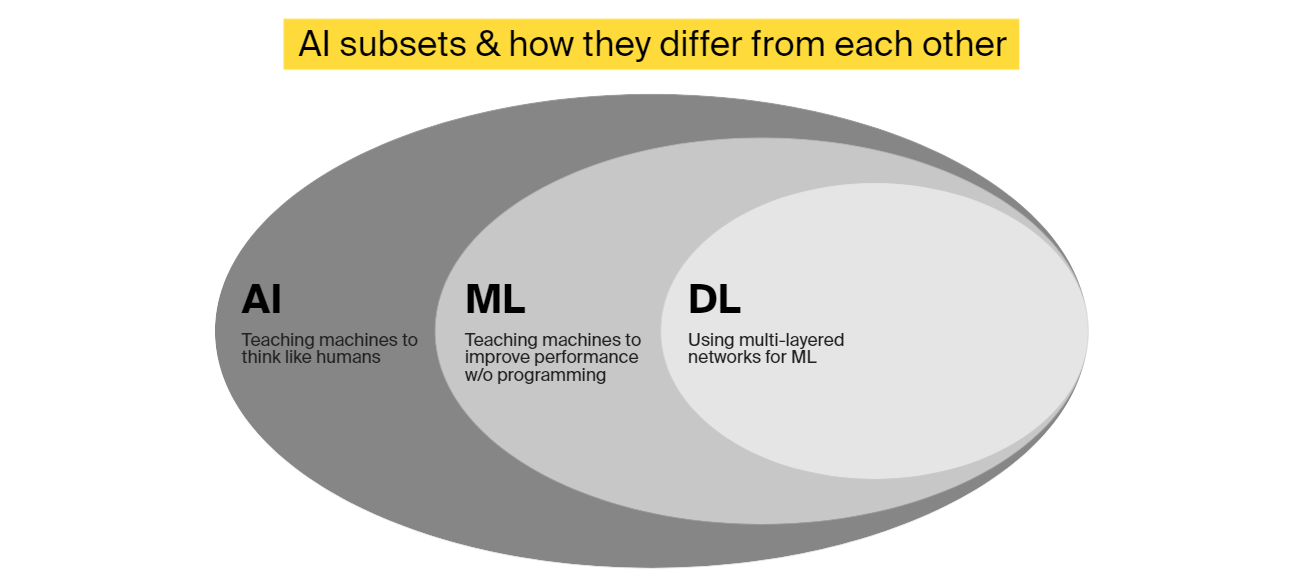
AI vs. machine learning vs. deep learning
What is artificial intelligence?
Unlike humans and animals, computers and software cannot behave intelligently unless they’ve been deliberately programmed to do so.
Artificial intelligence is a multidisciplinary field of science and engineering. Its goal is to create smart machines that emulate and ultimately exceed the full range of human cognition. Being able to mimic human reasoning and continuously learn from new data, AI-powered hardware and computer programs can simulate intelligent behaviour, such as autonomous decision making, visual perception, and speech recognition.
For this, different subsets of artificial intelligence, including machine learning, natural language processing, and computer vision, are used.
What is machine learning?
Machine learning is a subset of artificial intelligence. The technique involves training algorithms on labeled data—for instance, images of puppies and bagels.
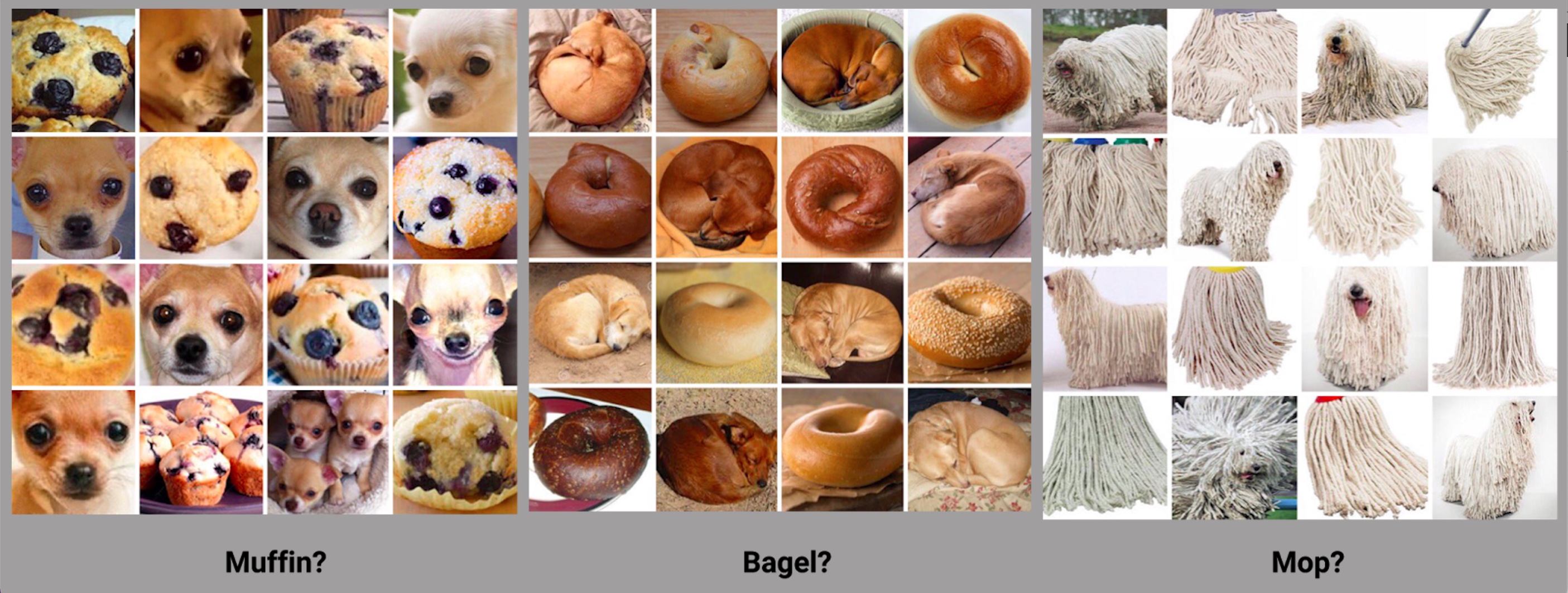
While basic AI systems often rely on if-then rules defined by human engineers, ML solutions alter themselves when faced with new information and improve their performance with time. To boost the accuracy of machine learning algorithms, developers calculate the bias and variance of a given model and intentionally program it to minimize errors.
Types of machine learning algorithms
-
Supervised algorithms lean on labeled data to find patterns and make intelligent predictions. Human engineers know in advance what the model’s output is likely to be. Supervised learning is best for classification tasks—for example, predicting real estate prices based on the square footage of a house, number of bedrooms, availability of a garden, etc.
-
Unsupervised models are exposed to unlabelled data, meaning engineers leave it up to smart algorithms to structure information and spot trends. Unsupervised machine learning is used in clustering tasks like identifying customer segments in CRM data.
-
In reinforcement learning, algorithms do not simply guess outputs based on the received data. Instead, they interact with the environment to find the best way to solve a problem, learn from mistakes, and get rewards when delivering accurate results. Some practical examples of deep learning include stock trading bots and computer applications that summarize long texts. OpenAI’s ChatGPT has also been created using reinforcement learning—specifically, the reinforcement learning from human feedback (RLHF) approach, which involves communication between the model and human trainers. In this approach, human specialists interact with AI in a conversational manner and rank its responses or provide better alternatives to tweak it.
What is deep learning?
Deep learning, a subset of artificial intelligence (or, more specifically, machine learning), uses neural networks with multiple hidden layers to extract additional insights from input data and make better predictions.
In contrast to linear algorithms, neural networks use artificial neurons modeled after the human brain. When neurons receive input data, they assign weights to it; the combined weight is then compared to a predetermined threshold, which represents the probability or improbability of an event. A neural network must have at least three layers to qualify as “deep.” While deep neural networks may use labeled data to guide their algorithms, they can also learn on their own.
Voice assistants like Alexa and Siri are examples of deep learning systems, as they capture human voice, compare audio waves to phonemes in a target language, and figure out what people say.
Deep learning has recently gained traction in advanced natural language processing and computer vision systems.
The former are distinguished by their ability to comprehend written or spoken language and provide appropriate responses.
The latter seek to understand the content of digital images; this is how Google Images, Face ID, and intelligent video surveillance systems operate.
How different AI types and subsets are used in business
Now that we know the difference between various artificial intelligence types and subsets, it’s time to explore how we could use these technologies to supercharge enterprise software solutions, changing the way we’ve been working for decades.
On a broader level, we could single out the following use cases:
-
Uncovering insights in operational data
A couple of years ago, Google turned to deep neural networks to manage a cooling plant at one of its data centers. The moonshot project began as a recommendation engine that would come up with random suggestions to help human engineers optimize energy use. As the system absorbed new data, its performance improved significantly. Google let the smart algorithms make the tweaks on their own and registered a whopping 40% reduction in the facility’s energy use. It should be noted that deep learning is just one of the AI subsets that can be used for data analytics tasks. For structured or semi-structured data, supervised machine learning algorithms could be just what the doctor ordered. Collaboration with skilled technology consultants is therefore essential for selecting the appropriate type of AI and implementation approach.
-
Automating digital and physical tasks
While today’s reactive and limited memory machines display poor results without human supervision, AI-powered systems confidently take over some repetitive and time-consuming jobs on the factory floor, in the office, and at call centers. Since 2019, Amazon has been using packaging robots that box up 700 orders per hour. Humana, a leading US healthcare insurance company, automates 60% of its call center activities using voice assistants and AI-driven intelligent process automation (IPA) technology. A global retail company created an AI platform to get a 360-degree view of all the data and documents accumulated in its technology systems and automatically update or delete inaccurate information. Shimizu Corporation is currently testing the OpenSpace artificial intelligence platform to analyze photographs taken at construction sites and track progress. When generative AI begins to have an impact on enterprise IT budgets in 2025, we can expect to see even more exciting applications of various artificial intelligence types for business process automation.
-
Improving user experience
Aside from answering customer questions at the help desk and assisting users with online catalog navigation, AI systems can delve deeper into social and purchase history data to generate relevant product recommendations. 80% of movies and TV shows people stream on Netflix, for instance, are discovered through the company’s smart recommendation engine. Another example comes from Walmart, which uses AI cameras to monitor inventory levels and promptly restock items that customers buy the most. And with voice assistants, thriving consumer electronics and automotive brands are elevating user experience to new heights—just ask Alexa to book you a flight with Ryanair or dial your friend’s number without taking your hands off the steering wheel to see the difference!
-
Enhancing security
While we saw two years’ worth of digital transformation in the first two months of the COVID-19 pandemic, cyberattacks targeting businesses of all shapes and sizes grew by 273% during the same period. As more companies shift apps and data to the cloud and adopt hybrid work policies, it is essential to look beyond antivirus software and hardware-enforced protection of OT and IT networks—and here’s where different AI types and subsets come in useful. As an alternative to passwords and PINs, enterprises may restrict access to corporate devices and applications using biometric authentication systems. Facial and voice recognition features steadily infiltrate corporate messengers, including WhatsApp for Business. And machine learning-powered platforms like Robust Intelligence could help companies monitor network traffic and detect over 100 types of cyberattacks.
Over the next decade, greater adoption of AI can result in 1.5% annual productivity growth across the key economic sectors. While we are still years away from human-like AI that can fully automate knowledge-intensive processes, even “basic” data classification and clustering systems offer significant time and cost savings to businesses.
Finally, our guide to different types of artificial intelligence would be incomplete without an assessment of AI implementation costs. For more information on the topic, refer to these blog posts.