What is big data in healthcare, and how is it used?
Big data has several accepted definitions. Here are two popular ones:
-
Douglas Laney’s definition. Laney is a former Chief Data Officer at Gartner. He states that big data is characterized by 3 Vs: volume, velocity, and variety. Volume stands for large amounts of data. Velocity refers to the speed of collecting data and making it accessible, while variety indicates the different types of data, such as text, video, logs, audio, etc.
-
McKinsey’s definition. The renowned consulting firm defines big data as datasets whose size is beyond the ability of typical database software tools to capture, store, manage, and analyze.
According to an IDC report, the volume of big data is expected to reach 175 Zettabytes by 2025. To put it in perspective, it will take 1.8 billion years to download this amount of data with the average internet speed available nowadays.
Big data in healthcare: sources and benefits
Healthcare big data refers to the massive amounts of health-related data coming from various sources, such as electronic health records (EHRs), genomic sequencing, medical research, wearables, and medical imaging, to mention a few. This data is enormous in its volume and diverse in its format, making it difficult to store in conventional databases, and is too complex for traditional data processing technologies.
There is an emergent discussion that “big” is no longer the defining parameter. For the healthcare sector, it is more important how “smart” the data is and which type of insights it provides.
Benefits of big data in healthcare
The healthcare sector is lagging in big data adoption due to the sensitivity of healthcare information. Despite big data problems in healthcare, hospitals are eager to deploy innovative technology to unlock the benefits of big data in medicine. These benefits include:
-
Reducing costs
-
Improving patient outcomes
-
Advancing competitive position
-
Understanding an organization’s performance
-
Detecting illnesses at early stages
-
Enhancing personnel management
Two main trends are currently pushing big data adoption in the medical field:
-
The movement towards a value-based care model that rewards clinics for their patient population health.
-
The need for evidence-based information to understand the best practices related to diseases and injuries management.
Big data analytics for healthcare
To extract meaningful insights from big data, healthcare organizations resort to analytics. Big data analytics is the process of uncovering patterns in large amounts of data with the help of different techniques originating in mathematics, statistics, computer science, and economics. McKinsey’s big data report enumerates the most prominent ones:
-
Machine learning:
it is a subset of AI. This technique trains algorithms on labeled data.
-
Data mining:
uses statistics and machine learning to extract patterns from datasets.
-
Natural language processing:
this technique is a subdomain of AI and linguistics. It uses algorithms to analyze human language.
-
A/B testing:
compares test groups with a control group to identify which changes have impact on the examined variable.
-
Data fusion and data integration:
techniques that integrate data from diverse sources and analyze it.
-
Statistics:
collect, classify, and interpret data from experiments and surveys.
Types of big data analytics in healthcare
The role of big data analytics for healthcare is to separate information from the noise and extract insights that will benefit all stockholders, from doctors to patients to hospital managers to medical tech startups. There are four main types of healthcare big data analytics:
-
Discovery analytics:
analyzes raw, incoming data to identify patterns and outliers. If the incoming data is incomplete and full of noise, the results will be questionable.
-
Descriptive analytics:
uses historical data to analyze trends and help understand what happened in the past. It doesn’t predict future events.
-
Predictive analytics:
depends on modeling techniques to forecast what will happen in the future, given that all conditions will remain constant. If circumstances change, the prediction loses its validity.
-
Prescriptive analytics:
employs machine learning to analyze data from multiple sources and suggest a course of action. These techniques are still limited in their capabilities.
7 use cases of big data in the healthcare industry
-
Enabling real-time alerts
-
Improving population health
-
Facilitating medical research
-
Contributing to cancer treatment
-
Preventing cyberattacks and fraud
-
Managing mental health conditions
-
Mitigating hospitalization risks
1. Enabling real-time alerts
Clinical decision support (CDS) software and other healthcare software solutions analyze data in real time, offering timely advice to medical professionals while diagnosing patients or composing treatment plans.
This example of big data in healthcare is particularly relevant when speaking of wearable medical devices. Wearables constantly collect patient data, which is then analyzed and presented to doctors, enabling them to react immediately if the results are alarming. For example, if a patient’s blood pressure suddenly increases, their doctor will receive an alert on the spot and administer measures to help the patient.
Furthermore, real-time alerts can be useful to monitor hospital staff and ensure compliance. One example comes from the collaboration between ITRex and Connecticut-based Hygenix Inc., a patient safety technology company. Hygenix wanted to monitor healthcare employees’ handwashing compliance and give timely notifications if the hygiene standards were breached. ITRex built an IoT ecosystem, which included a smart wristband, software application for data management, data analytics and visualization tool.
The results of deploying this solution in hospitals were astonishing. The clinics witnessed a 70% increase in hand hygiene compliance throughout the first week, the duration of handwashing has doubled, while sanitizer use has tripled.
2. Improving population health
Population health research helps medical clinics understand the vulnerabilities of specific cohorts of patients and address the issues before they escalate. One example of this big data application in healthcare is developing models that predict the risk of falling for seniors in the age group of 75 to 85 years old. For an accurate prediction, one needs to combine data from diverse sources, such as EHRs, to understand their medical history, and social factors to see their living conditions. Demographics do not restrict population cohorts. They can also be formed based on shared medical conditions, lifestyle, risks, etc.
Connecticut-based SCIO Health provides analytics solutions on a global scale. Their technology uses big data to identify care gaps that result in increased costs and bad patient outcomes. After seeing these gaps, doctors can preemptively target at-risk patients and avoid hospitalization.
Another example comes from Massachusetts-based Linguamatics, which uses predictive analytics and natural language processing to interpret unstructured health data and identify lifestyle factors that can lead to health complications in the future.
Moreover, medical researchers can use predictive modeling to analyze disease outbreaks. This gives an opportunity to develop targeted vaccines faster and prevent a health crisis. At the moment, big data has the potential to predict COVID-19 hotspots as algorithms can analyze medical history and trace contacts of infected patients.

3. Facilitating medical research
Another impact of big data in healthcare is contributing to drug production. Drug discovery, creation, and acceptance is a tedious process regulated by strict protocols. It involves multiple rounds of testing, and the time to market can be rather lengthy. With big data and machine learning, scientists apply data models to predict potential drug effects instead of carrying out actual lab experiments, which are time-consuming.
Moreover, conventional pre-clinical drug testing is performed on animals and is not fully representative of human outcomes. Artificial intelligence and big data in healthcare allow simulating drug effects on virtual humans. When the time comes, big data repositories help researchers locate and recruit suitable patients for advanced stages of drug testing.
Identifying drug molecules
Another use case of big data in medical research is finding the right drug molecules. After scientists identify a biological target, they start researching molecules that can interact with it and produce the desirable effect. A health tech startup, Atomwise, employs deep learning neural networks for medicine discovery. With their software, the company managed to go through 8.2 million compounds in a few days to find a potential cure for multiple sclerosis.
Organizing medical knowledge
Big data in healthcare analytics also contributes to organizing the vast medical knowledge base. For instance, New Jersey-based Innoplexus developed a discovery tool that arranges medical dissertations, articles, clinical trial documentations, etc. and makes these materials searchable for pharmaceutical companies developing new drugs.
4. Contributing to cancer treatment
Big data analytics benefits cancer research as scientists need to go through vast amounts of data to unveil remedies with the highest success rate. They examine tumor samples from biobanks and link them to patients’ medical records to discover how cancer proteins interact with different treatments. This big data examination can yield unexpected results. One such study discovered that Desipramine, an antidepressant, can aid in curing certain types of lung cancer.
However, this type of big data analytics requires a comprehensive cancer database that would integrate information from hospitals, medical universities, and other relevant organizations. New York-based Flatiron Health developed a solution that connects different players in this field, from oncologists to academics, and allows them to learn from each other. The company offers access to billions of data points from different cancer patients.
Big data analytics and breast cancer
Big data contributes to breast cancer detection. The traditional breast cancer prediction models consider only a limited set of factors, such as family history, past biopsies, and breast density. Big data analytics can accommodate a higher level of detail from mammograms and patient records.
Recently, researchers at Massachusetts General Hospital developed machine learning algorithms that can evaluate the risk of breast cancer by identifying imaging biomarkers on mammograms. Here is what Leslie Lamb, Division Chief of Breast Imaging at the hospital, said about this big data tool: “Why should we limit ourselves to only breast density when there is such rich digital data embedded in every woman’s mammogram? Every woman’s mammogram is unique to her just like her thumbprint. It contains imaging biomarkers that are highly predictive of future cancer risk, but until we had the tools of deep learning, we were not able to extract this information to improve patient care.”
5. Preventing cyberattacks and fraud
Healthcare providers are among the most targeted organizations when it comes to cybersecurity breaches. This sector accounts for nearly four out of five industrial breaches. The costs of these security violations amounted to $4 billion in 2019. This is not surprising, considering the value of personal health data. A healthcare record can be sold for $250 on the black market, compared to only $5.4 for payment card information.
Healthcare organizations are opting for big data analytics in healthcare to block security threats by monitoring changes in network traffic or any other relevant behavior.
Predictive analytics in healthcare using big data also help prevent insurance claims fraud as they use a combination of rules, data and text mining, and database searches.
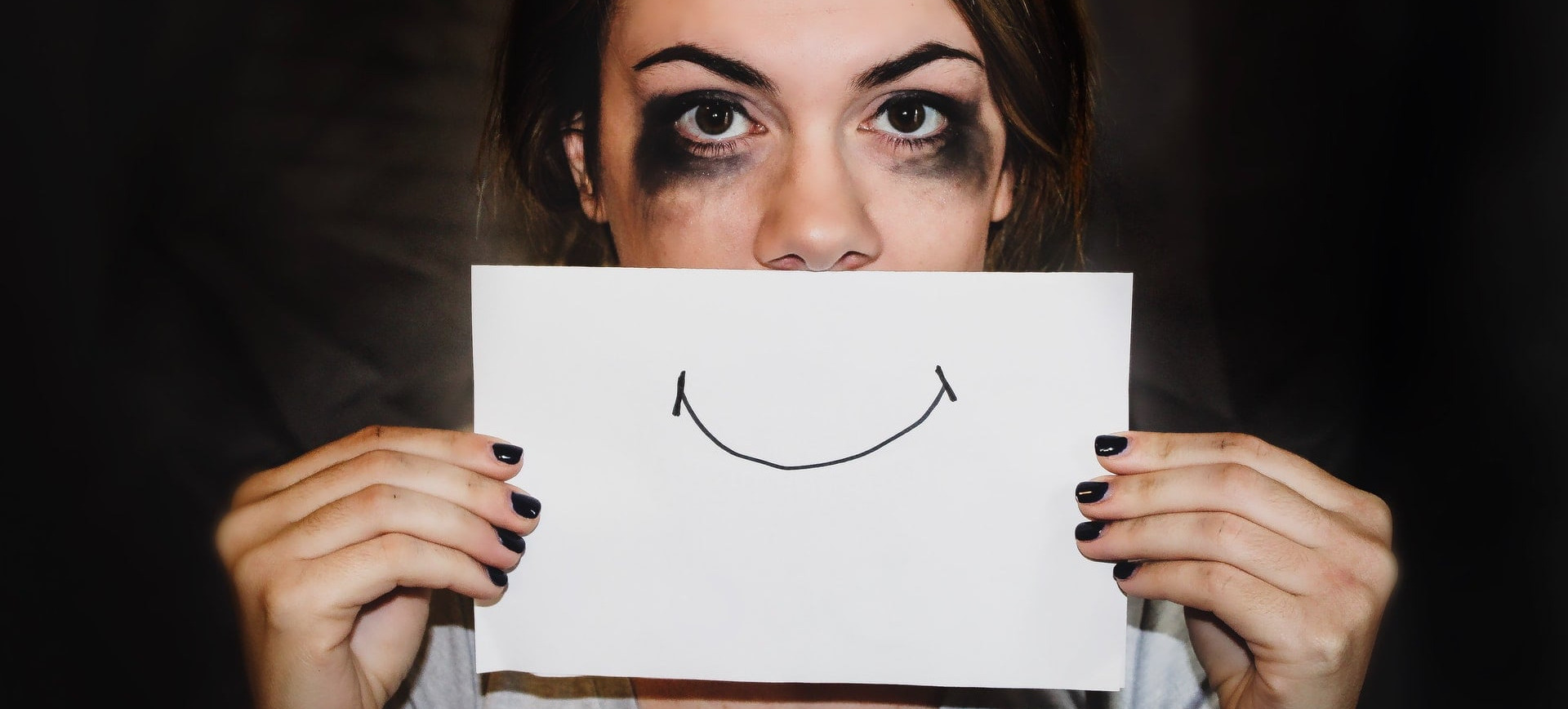
6. Managing mental health conditions
This is one of the most relevant big data examples in healthcare to COVID-19.
According to the National Institute of Mental Health, one in five adults in the US lives with mental illness. Big data and AI can advance mental health education and understanding. The powerful duo enables therapists to analyze data from social media, wearable devices, internet activities, and other sources using natural language processing tools to identify psychological markers associated with particular mental conditions.
The World Psychiatry journal published a study showing that speech elements can hint at mental disorders. For instance, the frequency of using possessive nouns is an indicator of whether someone at risk of psychosis will actually become psychotic. The tool presented in the journal had 83% accuracy.
Suicide prevention
How big data helps healthcare in suicide prevention? Crisis Text Line provides crisis counseling via text to English-speaking countries. It uses big data and machine learning models to prioritize people who need help. As the demand often exceeds the staff capacity, not everyone can be helped on time. The algorithms analyze unstructured text to spot patients who need support urgently.
The Crisis Text Line tool presented some unexpected findings. For example, the words “Ibuprofen” and “Advil” are 14 times more predictive of suicide than “die” and “cut”. Also, the crying-face emoji is 11 times more predictive of suicide than the word “suicide” itself. If you don’t know this in advance, you can give priority to the wrong people and fail to offer support to the ones who really need it.
7. Mitigating hospitalization risks
Big data and healthcare analytics can help track hospitalization risks for patients with chronic diseases. By analyzing various criteria, such as symptom severity, medication intake, and the frequency of doctor visits, clinics can provide targeted preventive care to reduce admissions. Simultaneously, by predicting who might be admitted, hospitals can allocate space and resources to prospective patients.
During the pandemic, doctors are using big data in healthcare to gather data from all over the country to identify the spots that are at the greatest risk. According to Sampson David, an Emergency Medicine Physician, inspirational speaker, and a co-founder of The Three Doctors Foundation, “So now, we have to think about how we gather and share data across the country to pinpoint where the need is the greatest. And by knowing where the need is the greatest, we can then say that if we have a vaccine or treatment, we can start there to see what type of impact this treatment may have.”
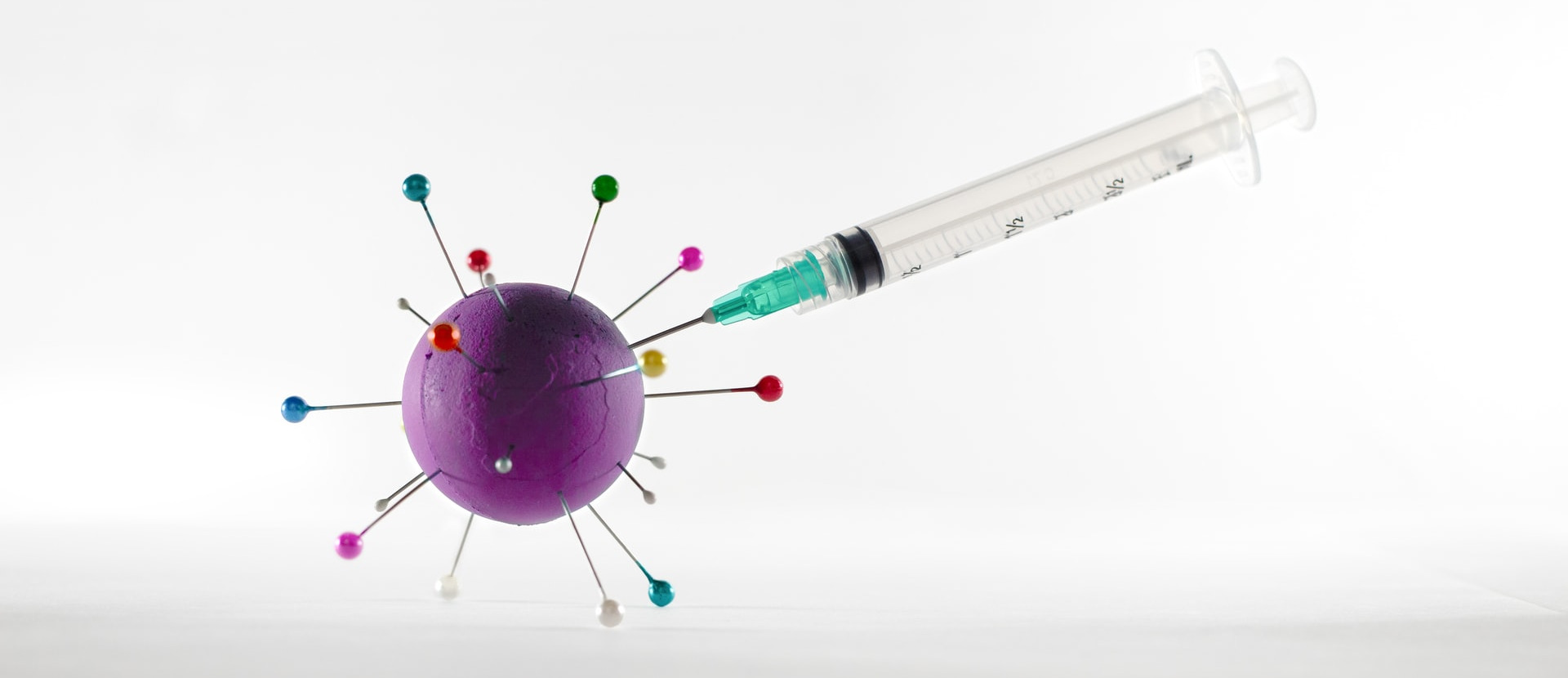
Challenges of big data in healthcare
The importance of big data in healthcare is undeniable, but many challenges surround its implementation. Deloitte recently conducted the State of AI survey to evaluate how different organizations worldwide employ/plan on using AI. This survey revealed that AI costs are still among the most prominent obstacles.
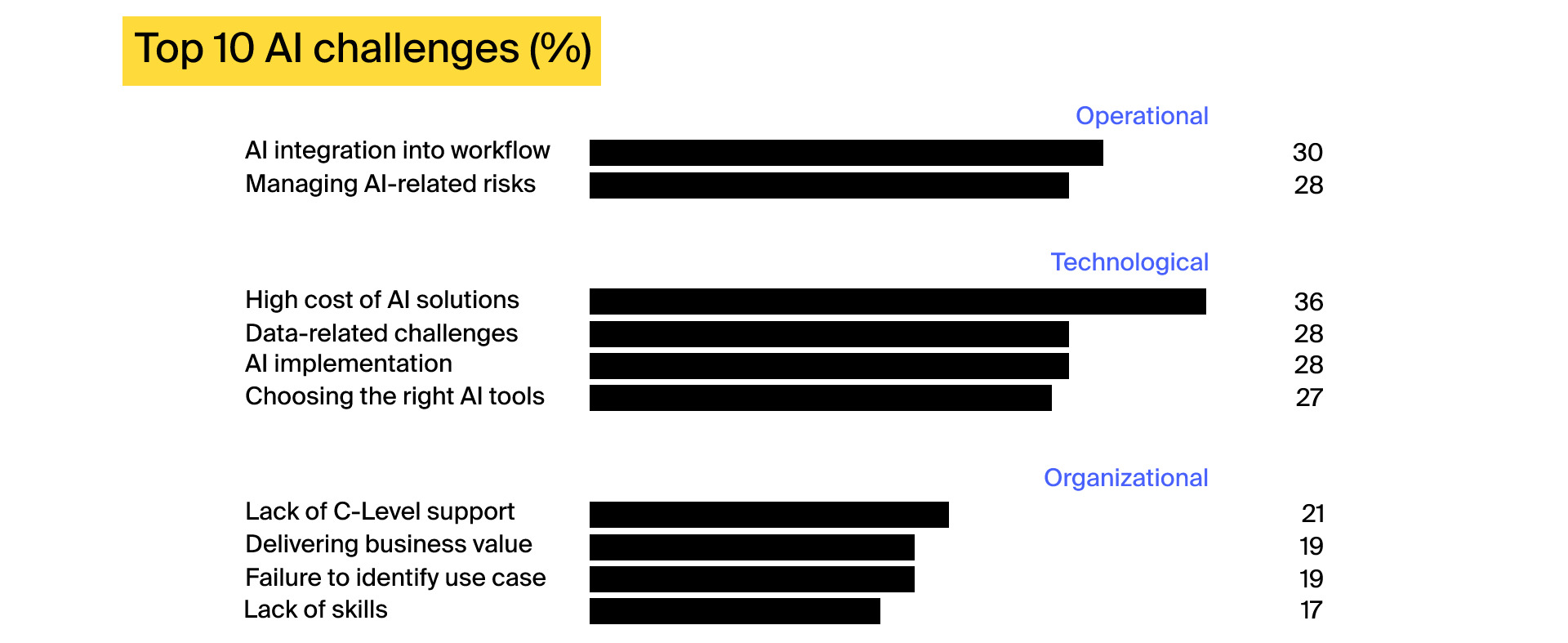
Here are six challenges of big data relevant to the healthcare industry:
-
Implementation costs
-
Data aggregation and cleaning
-
Security
-
Communication gap
-
Management-related issues
-
Interoperability
1. Implementation costs
Costs still present a considerable challenge to adopting big data in healthcare. Medical clinics will need to purchase technology, acquire computational tools and software to manage the data, and purchase/develop custom applications to benefit from big data analytics.
For example, building a custom patient engagement solution that works with big data can cost at least $120k.
Another item on the expenditure list is salary payments. Organizations will have to hire data scientists and teach their staff how to work with data, which is also paid time that employees spend on learning instead of doing productive work.
There is no way to bypass initial investment, but if hospitals consider all the possible expenses (even the indirect ones) beforehand, they will avoid unpleasant surprises in the future.
2. Data aggregation and cleaning
Patient data is spread across multiple sources, including payors and other medical organizations where patients received care. Pulling the data together will require collaboration between different parties and agreeing on a particular data format. Also, big data is heterogeneous and unstructured. Organizations will need to apply classification techniques to make this data suitable for analysis.
Moreover, you will need to clean the aggregated data to ensure its consistency, accuracy, and correctness. The cleaning process can be either manual or automated based on logic rules. You will also need to determine how to deal with the new data coming in real time.
Medical imaging big data requires special attention when it comes to storage and processing. Image mishandling can cause the introduction of noise, which, for example, can lead to the delineation of anatomical structures. As a result, the image will no longer correspond to reality.
3. Security
As mentioned above, data breaches are common in the healthcare sector, and they are costly in terms of finances and reputation. It is important to choose well-trusted vendors for big data in healthcare technology and implement encryption and other security measures. It is also essential to secure all the connected devices on the hospital network. When necessary, restrict access to some applications to predefined devices only, and limit the authorized personnel.
Hospitals need to educate their staff members on data usage rules. Studies reveal that human error accounts for 31% of data breaches in the healthcare sector. Hence, it is crucial to explain how to access data safely and secure personal mobile devices (if used for work).
Last but not least, ensure your antivirus software and firewall are up to date. Also, promptly update all data management programs as soon as the new version is available.
4. Communication gap
Miscommunication between data scientists and data users presents another common challenge of big data in healthcare. Doctors may not be able to adequately explain which data they need and how they prefer to store and access it. As a result, they will not understand the formats generated by data scientists and will avoid using this data and analytics to the full ability.
5. Organizational issues
Big data is changing the healthcare sector, and becoming a data-oriented organization requires alterations in the company’s culture and the way it makes decisions and conducts business. Recruiting new talent will be unavoidable. Existing employees will also need training and an adjustment period. Some organizations might be forced to replace their IT infrastructure, which means additional expenses and training hours. When using big data in decision-support activities, staff members might not be immediately eager to trust the recommendations delivered by data-based solutions.
6. Interoperability
This challenge will arise if a healthcare organization wants to cooperate with other parties within its ecosystem. Many clinics are still following the old-school approach, resorting to pen and paper. So, if you digitalized your data, do not assume that others have done the same. Even if your selected partner is fully digital, you can face semantic challenges as their applications are likely to use different terminology to describe the same thing. All this needs to be discussed before using big data in healthcare.
Moreover, if your cooperation is on an international scale, you might encounter discrepancies in privacy laws.
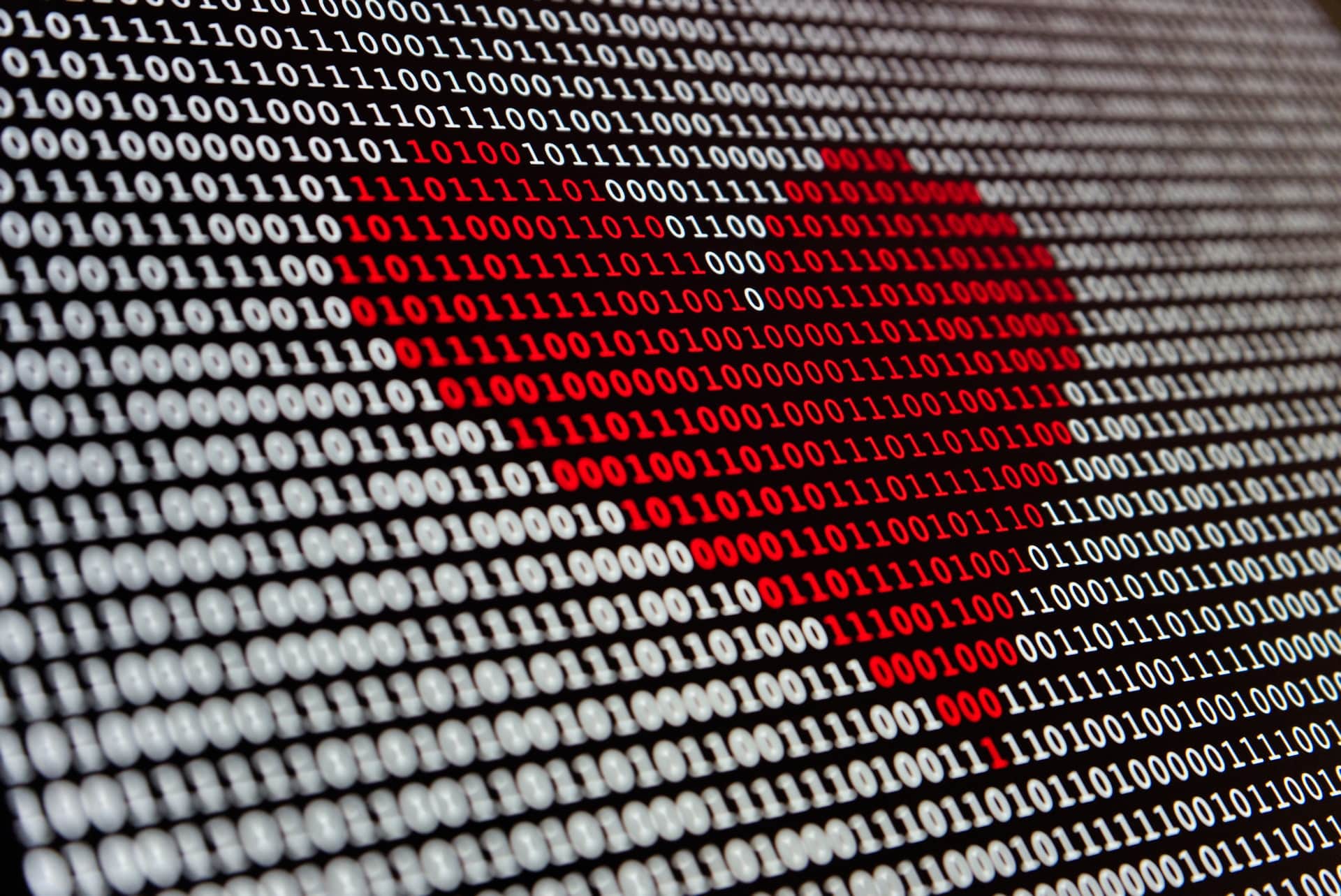
How to move forward with big data in healthcare
Multiple problems are surrounding big data in healthcare, but if implemented correctly, it will pay off. You can set yourself up for success by following these steps:
On the managerial level:
-
Commit to a vision of becoming a data-driven organization. Identify the current processes that will need adjustment and be ready to implement the change. Communicate your ideas to the employees.
-
Ensure that you have the key stakeholders’ support to avoid misunderstandings at later stages of the project.
-
Prepare to recruit new talent, including data scientists and developers, or collaborate with a trusted tech partner.
-
Commit to training your existing employees. Allocate time and budget for this purpose.
On the data level:
-
Decide on data storage options, whether it’s in the cloud or on-premises. The on-premises approach gives you control over access and security. But the cloud is cheaper, offers recovery plans, and facilitates expansion. It has been the preferred option for most healthcare organizations. Specially that cloud technologies are gaining in security and reliability. If you opt for the cloud, search for a cloud partner who understands healthcare-specific compliance and security requirements.
-
Make sure your infrastructure is scalable and can follow along with the unavoidable growth of data volumes.
-
Set up strong data governance practices, define the desired level of data quality and accuracy, and determine how the incoming data will be filtered.
-
Define data ownership and appoint owners of different datasets. These people will be accountable for data quality, completeness, etc.
In the end, big data in healthcare is purely a means to reach your strategic goals. There are several factors that you need to consider in the process.
As Robert Millette, Executive Director of Population Health at Lee Health, said during his interview with HealthITAnalytics: “Number one, you need to have a strategy. You need to know how you’re going to differentiate yourself in whatever area you’re looking to support with that tool. You should also remember that the unified data platform is simply a tool – it’s a means to an end. It is by far not the only thing. You have to have network infrastructures, and you have to have the right contracts with payers to move and perform in value. There are a number of different levers you need to have, and they’re all important.”