Key principles of a data management strategy
A data management strategy outlines a high-level course of action to enable data management.
The Data Management Body of Knowledge(DAMA-DMBOK2), written by over 120 data management practitioners, defines data management as the development, execution, and supervision of plans, policies, programs, and practices that deliver, control, protect, and enhance the value of data and information assets throughout their lifecycles.
In layman’s terms, data management is basically about:
-
Empowering insightful data analytics and data science with accurate, complete, consistent, reliable, and current data
-
Ensuring data privacy and security in accordance with regulatory requirements
-
Managing data infrastructure, from databases to data warehouses, in a cost-efficient manner
-
Building up data literacy across the organization
Data management is not a one-time initiative but an ongoing program that is complex.
Depending on the industry and business needs, data strategies can vary, but all of them cover 10 knowledge areas, with one area — data governance — at the center. Below is a table that provides a summary of each knowledge area and why it is critical.
10 components of a data management strategy
Data Management Component | What it is | Why it is needed |
---|---|---|
Data Governance |
A program that exercises control over the management of the organization’s data assets and includes:
|
|
Data Architecture |
A structure of the company’s current data assets and data management resources that maps how data flows through systems |
|
Data Modelling |
A process to discover how the company’s data fits together and communicate the data structures and relationships in:
|
|
Data Storage & Operation |
The design, implementation, and support of stored data to maximize its value. Key activities include:
|
|
Data Security & Privacy |
Security policies and procedures aimed at ensuring proper authentication, authorization, access, and auditing of data assets |
|
Data Integration & Operability |
The movement and consolidation of data within and between data stores and applications, with:
|
|
Document Management |
Controlling the capture, storage, access, and use of data stored outside relational databases |
|
Master Data Management |
Controlling master data (key business data about customers, products, suppliers, locations, etc.) to:
|
|
Data Warehousing and BI |
Operational extract, cleansing, transformation, control, and load processes that maintain the data in a data warehouse consisting of:
|
|
Metadata Management |
Control activities to enable access to high quality, integrated metadata that describes:
|
|
Who owns the data management strategy:
As a rule, the data management strategy is owned by the Chief Data Officer (CDO) and implemented through a data governance team with support from a Data Governance Council. It is the CDO who drafts an initial data management strategy and gets executives’ buy-in for establishing data governance and data stewardship to monitor data practices.
How an initial data management strategy can look like:
-
A compelling statement on data management vision
-
Critical business cases based on the organization’s data priorities
-
Guiding principles and management perspectives
-
A mission statement and long-term data management goals
-
Measures of data management success
-
Short-term objectives of the data management program that are SMART (specific, measurable, actionable, realistic, time-bound)
-
Descriptions of data management roles, responsibilities, and decision powers
-
A draft implementation roadmap with high-priority projects and action items
How to set the stage for data management
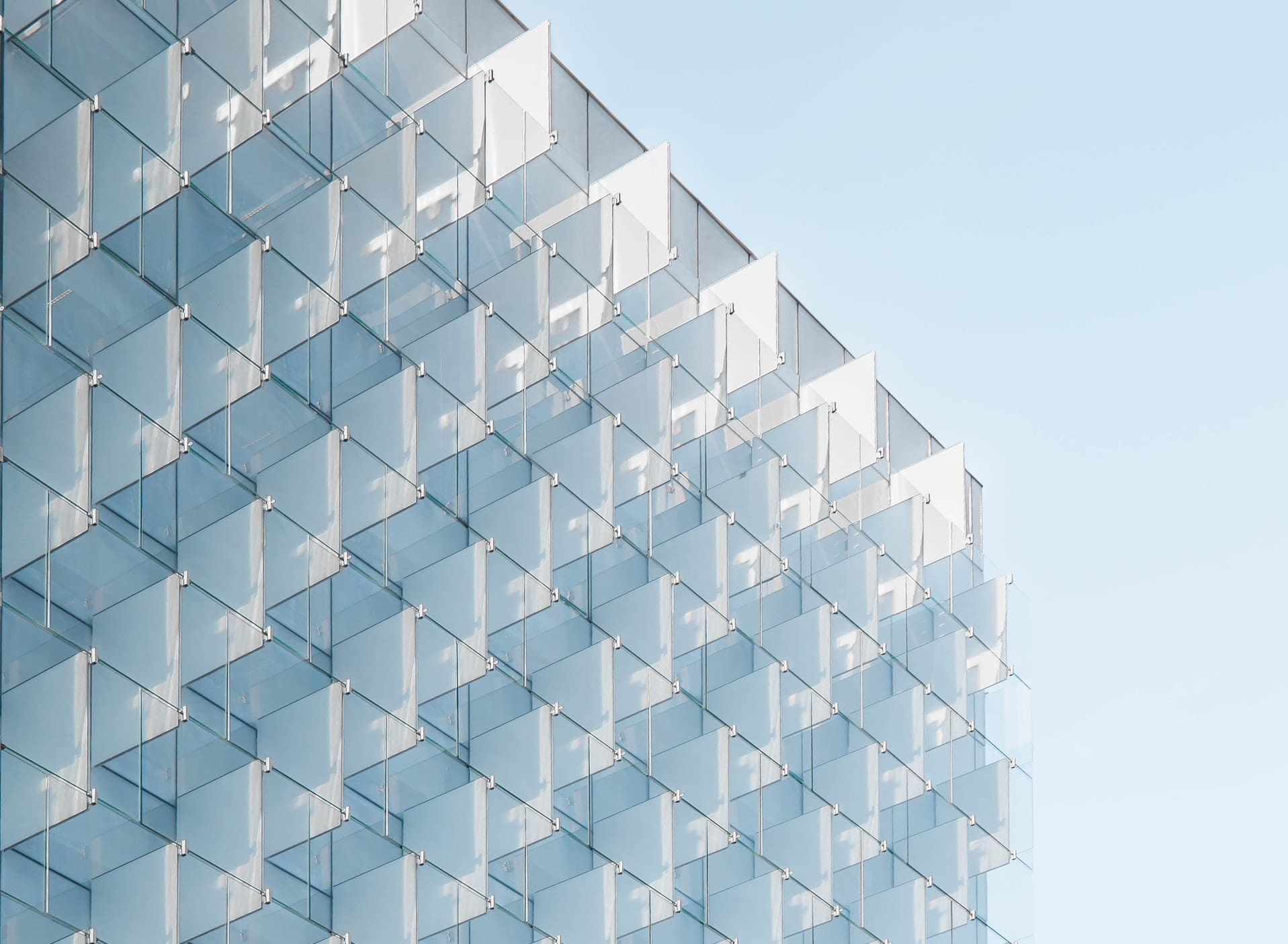
Overall, only about 20% of companies are reporting success with managing their data, with less than a third saying it is “very easy” for users to access data and extract insights.
Poor data quality and a lack of expertise in data governance or process automation remain serious barriers on the big data journey. It would be hardly possible to cover in detail all tactical steps that building a successful data management strategy involves. However, there are some best practices any organization starting at square zero should keep in mind.
Dive in.
The five steps to building a successful data management strategy:
-
Define goals around data. Your actual business problem should inform your data management strategy. Otherwise, it will be just a cargo cult wasting resources.
So, the first question your business stakeholders should answer is what business goals they try to achieve with data. Do they want to understand customers, come up with an innovative product, fix a business process, cut operational costs, or control specific risks better?
The answer will help you define the focus of your data management strategy, tools, and processes down the line. You will also be able to set metrics of success.
-
Set data processes. Once your end goal is clear, you will need to figure out what processes should be put in place as part of data activities. The following questions will be useful:
For data sourcing: How and when will you collect data? This includes an understanding of data sources you should use or acquire.
For data enablement: Where and how will your data be stored and integrated? How will you maintain and improve data quality, integrity, access, and security?
For data use: Who will use the data and for what purposes (predictive analytics, prescriptive analytics, operational analysis, etc.)?
Think at once about automating your data processes wherever possible. According to recent findings, 80% of data practitioners’ time is spent simply finding and preparing data instead of extracting insights from it.
-
Identify the organizational model. At this point, you need to understand how your people and units will collaborate in data management. So the key question to answer here is whether your operating model will be centralized, decentralized, or hybrid. In other words, will there be a single data management owner responsible for data governance, stewardship, metadata management, data quality, master data management, and data architecture? Or will these responsibilities be distributed across different business lines and IT teams?
-
Find the right people and technology. You will need to find a qualified CDO or an external consultant. Their knowledge will help select the right tech stack for your data ecosystem, from data management platforms to databases and integration software.
Based on your data needs, you might also want to set up a strong data team. Here it’s important to identify employees that are already performing some data functions to involve them first. Database administration is the core skill required to manipulate data, while in many organizations, database administrators were previously software developers. Other fundamental skills include data infrastructure, data architecture, data governance, data visualization, and data analytics. Hire more talent only when your data management and governance evolves.
-
Educate your stakeholders. Your data management strategy will fail if the policies and procedures are not followed. All people who deal with data should be on the same page. That’s why it is critical to profile and educate your stakeholders.
Who will be affected by data management and in what way in terms of their roles and responsibilities? How may they respond to proposed changes? What worries might they have? Who could disrupt data management initiatives?
Answers to these questions will help identify the best approach to engaging different stakeholders.
Also, consider a company-wide education campaign to make sure leaders and teams understand the company’s data management goals and their role in achieving them.
Offensive vs. defensive data strategies: Which is right for your company
In an article published by Harvard Business Review back in 2017 to provide an overview of approaches taken by big names to designing data management, its authors say that organizations where data is both tightly controlled and flexible are extremely rare. To manage data efficiently, they suggest, companies should first determine where they want to be on the offense-defense scale.
Defensive data strategies emphasize strict controls over data security, privacy, and integrity to ensure regulatory compliance. Analytics is used to prevent fraud, and much effort is invested in creating systems to protect business from data theft.
Offensive data strategies, in contrast, focus on more sophisticated data science solutions and hence, flexibility in using data. They are primarily aimed at supporting customer-focused business lines in achieving their KPIs, from revenue to profitability to various customer service metrics.
Every organization needs both offense and defense in its data management strategy. However, for many, it is wiser to shift to one end of the spectrum or the other because resources are always limited.
For instance, companies in heavily regulated industries such as insurance, health care, and financial services should favor data defense.
In ultra-competitive industries like retail, the focus should be on offense to unearth new customer insights.
The defense vs. offense orientation is quite often used to identify an optimal design of data management function. A centralized data function with a CDO accountable for data management across the organization is more suitable for businesses that focus on data defense. Data offense can be better executed through decentralized data management, which typically has a CDO for each business unit reporting to the company CDO.
This control vs. flexibility concept is quite straightforward. However, things have changed since 2017.
Though the temptation to attack under incredible competitive pressure has never been stronger, defense has become an imperative for everyone.
With the adoption of far-reaching data privacy regulations, including the GDPR and the California Consumer Privacy Act, almost every company is now a regulated company regardless of the industry.
It means that companies can no longer afford saving time and resources on defense activities as part of implementing their data strategies. They need strong data governance and robust solutions to automate their data assets safely and at scale.
A better choice today would be a hybrid data strategy that also places a strong emphasis on compliance that is enabled by data governance.
In-house and outsourced data management
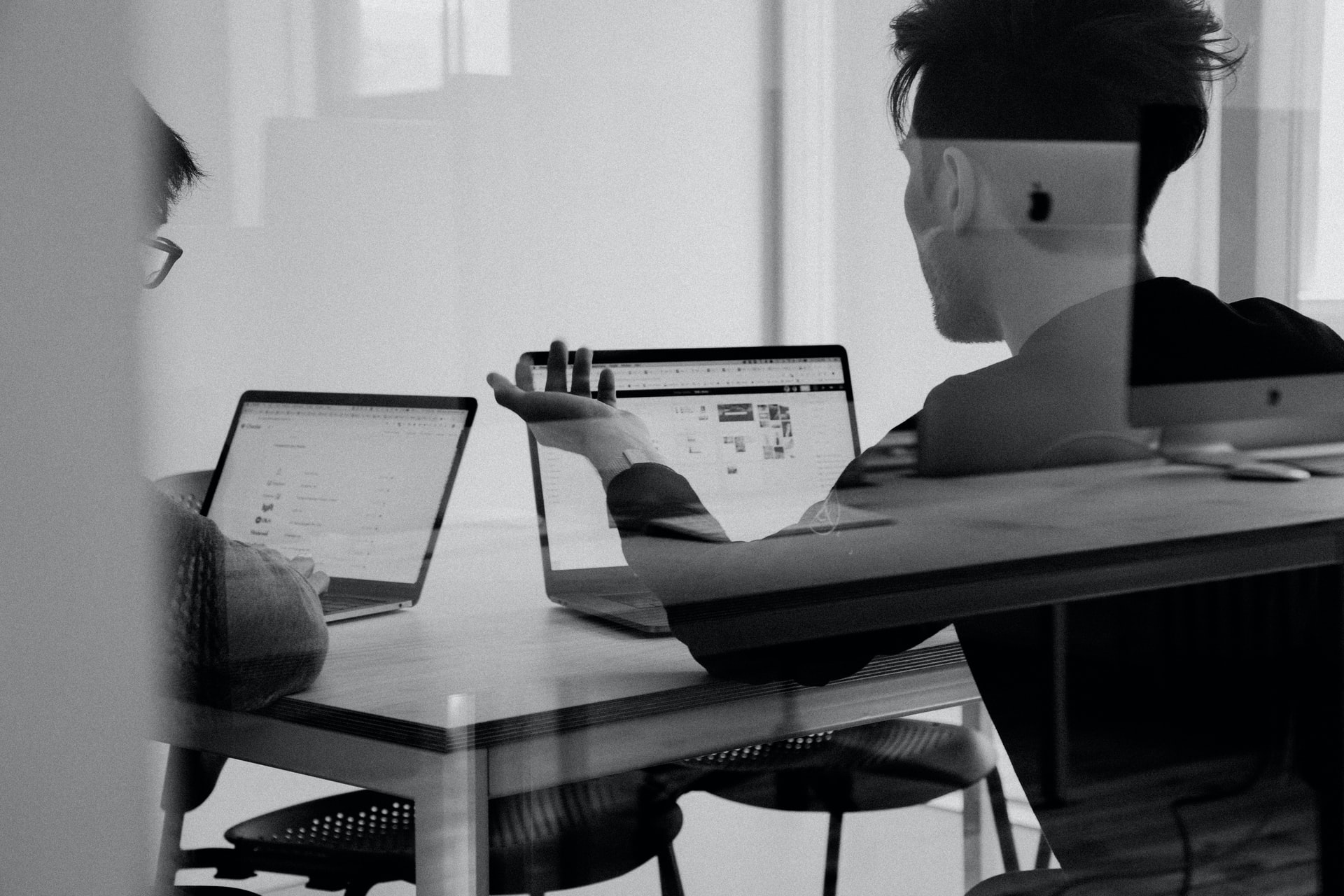
There’s a problem though.
Despite the fact that the number of organizations installing a CDO reached 65% in 2021 from just 12% in 2012, a talent shortage remains a roadblock to improving data management practices.
Finding much-sought-after data professionals is a big challenge today with demand long surpassing supply. Reskilling or upskilling one’s own IT staff takes time.
As a result, many turn to external expert help for designing a robust data management strategy.
In our experience, what such clients usually need is a roadmap outlining their key steps on how to get started.
One example from our client portfolio would be an owner of agricultural machinery spare parts plants that has acquired two companies in different countries. A year into the integration process, they still could not figure out how many spare parts of a particular type they were making a year. Their data silos were huge due to different analytics systems used in different locations, ruling out any business transparency.
Data was only part of their CIO’s responsibilities. Besides, the CIO lacked experience in data integration. So they applied to our data management experts for assistance with designing a solution to integrate disparate systems, from ERPs and CRMs to production databases.
Another client was a global retailer that wanted to build one system acting as a single source of truth for all data systems. However, they were not even sure if they had the right data or if it was possible to integrate it.
This client did have a big and versatile data team. What they needed was a leading-edge innovation arm. They wanted speed and agility, assuming that an external consultant would not be dragged down by red tape.
They made a good shot.
We were able to jump into their project right away, setting up a team fully devoted to the task at hand. We did a fast analysis of their data and available technologies, developed an implementation roadmap, and built a prototype. We innovated for them, too. Choosing graph data modeling as the best-fit approach and an open-source platform, we were probably the first guys in the world to build a custom security level for this platform to control access rights to data.
Finally, there have been clients with no data capabilities whatsoever, from a casino trying to get its head around online business performance to a restaurant owner struggling to understand what data they should capture in the first place.
To sum it up, an in-house data management function is a must for designing and implementing a value-adding data management strategy. However, an external experienced partner can also be of great help. This may be the case when a company does not know where to begin with, or lacks experience and diverse skills, or needs agility and innovation.
Final thoughts
As AI/ML solutions are becoming mainstream, the importance of data is only expected to increase.
Augmented analysis using ML algorithms empowers organizations to identify business opportunities through more and larger data sets available for discovery. The data they unearth is used to build predictive models that anticipate customer needs and drive innovation in products and services.
ML algorithms can also automate complex time-consuming tasks, while natural language processing (NLP) allows business users with no coding knowledge to make sense of data to derive critical insights.
On a more technical side, the exponential growth of graph technology used in data modeling is bringing efficiency, speed, and flexibility to data analysis.
The new-age technologies provide a windshield view for business, offering a better vision into where the company is going to.
But these benefits are out of reach without a proper data management strategy.