What is generative AI in a supply chain?
Generative AI is a technology that can create new content, such as text, images, and even documents, similar to the examples it witnessed during training. It’s like a smart assistant that can produce new content on demand without being specifically programmed for each content type.
In the supply chain context, generative AI trains on vast amounts of supply chain-related data, such as logistics information, sales history, inventory records, and so on, and produces different types of insights including optimized route maps, demand forecasts, supplier evaluation reports, restocking strategies, and more.
How is generative AI different from traditional AI technology?
Conventional artificial intelligence excels at analyzing historical data and identifying patterns. It has a wide range of capabilities including natural language processing, computer vision, machine learning, and more, while generative AI solely focuses on producing content that looks like it was generated by humans.
Examples of traditional AI in our everyday life include self-driving cars, recommendation engines on your favorite shopping website, or voice assistants like Siri or Alexa. Examples of generative AI revolve around content creation. They include ChatGPT, which produces human-like text, and DeepDream, which generates images.
You can learn more about conventional AI’s role in achieving supply chain resilience on our blog.
Benefits of generative AI for supply chain for your business
After deploying generative AI, even if you apply it for one or two use cases, your company will experience some or all of the following benefits:
-
Increased efficiency as generative AI can optimize processes, such as restocking and procurement, as well as find faster and cheaper shipping alternatives
-
Reduced labor costs as it will automate tedious tasks like forecasting and report generation
-
Improved scalability as AI can take over additional load without the need to hire more people
-
Enhanced customer satisfaction as algorithms can forecast demand and ensure the inventory never runs out of your customers’ favorite products
-
Streamlined operations as AI can predict and resolve supply chain issues
-
More productive employees as they now can focus on tasks that better fit their qualifications while AI models handle large report generation and other tedious tasks
Does it make sense to build custom or fine-tune existing generative AI models?
There are ready-made generative AI solutions, such as the C3 Generative AI, which can be used to improve supply chain visibility. While these solutions can be very powerful, a company using them for supply chain optimization can encounter the following challenges:
-
Lack of expertise in your domain because these solutions are often designed as general-purpose models
-
The outcome will depend on the training dataset. If it was of poor quality, contained bias, or simply didn’t match your company’s data, the generated content will reflect these issues.
-
The algorithms might produce something irrelevant to your business as they don’t understand the specifics of your data
For optimal performance, every organization can hire an IT for supply chain consultant to develop new or fine-tune existing AI models to add domain-specific knowledge. This approach will have the following benefits:
-
Increased accuracy
-
Tailored to your organizational needs
-
Easy to integrate into your processes
-
Full ownership of the technology in the case of custom development
-
Adhering to industry-specific compliance requirements
But keep in mind that custom-built algorithms are more expensive and take longer to deploy as they are built from scratch and need to be trained and validated. So, the final choice is a tradeoff between your business needs and the available budget.
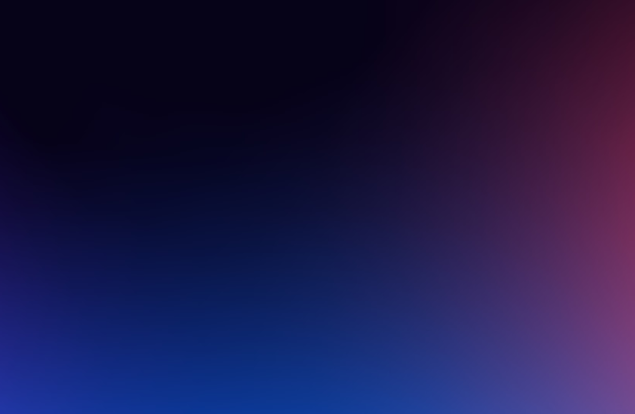
5 key generative AI use cases in supply chain
Use case 1: Approaching inventory with efficiency
Generative AI can analyze large quantities of data and come up with policies and suggestions on how to better handle stock given the current trends. Here is how this technology can contribute to inventory management:
-
Recommending dynamic inventory policies. Algorithms constantly analyze sales information and demand trends to suggest adjustments to inventory levels of various items in real time to respond to market needs.
-
Calculating safety stock levels to make sure the inventory doesn’t run out of popular items. AI models can compute optimal safety levels based on fluctuating demand, seasons, and other factors.
-
Simulating different scenarios that can affect stock, such as a sharp increase in demand and supply disturbances. This enables companies to come up with a contingency plan for restocking when needed.
-
Reducing inventory waste. Generative AI can spot slow-moving stock items that result in high holding costs and recommend strategies to improve product flow, like discounts and marketing campaigns.
-
Coming up with the most effective storage and distribution tactics for different products
Real-life example:
Stitch Fix is a fashion company headquartered in California. It trained generative AI algorithms on its extensive customer preference data and other information, and the model predicted which clothing products would be in high demand and made inventory restocking recommendations. As a result, the company reported a 25% decrease in costs associated with storage and item handling.
Use case 2: Delivering shipments faster and cheaper
Companies can use generative AI for supply chain management to analyze large amounts of data on weather conditions, traffic patterns, shipment, etc. to build optimized route maps so that suppliers can deliver products/materials faster and cheaper.
These models can also monitor data in real time to redirect shipments that are already in transit if there are traffic jams, accidents, or any other issues along the planned route. Such dynamic routing plans will help drivers adjust on the fly and avoid wasting hours being stuck in traffic.
Companies recognize this benefit, and the generative AI in logistics market is growing rapidly. It was valued at $412 million in 2022 and is expected to skyrocket to $13948 million by the end of 2023, growing at a CAGR of a whopping 43.5%.
Real-life example:
One manufacturer integrated generative AI into its operations to manage stock and optimize supply chain processes. The system analyzed real-time data and recommended re-routing options. The company reported cutting logistics expenses by 12% within the first six months of AI deployment.
Use case 3: Ensuring a sustainable and ethical supply chain
Research shows that business leaders are moving towards an ethical supply chain, which includes sustainability efforts, and generative AI can help in this initiative. Algorithms can analyze publicly available supplier data, such as energy efficiency, waste production, sustainable manufacturing practices, and raw material sourcing, to decide which supplier fits better into your environmental impact goals.
Additionally, AI models can pinpoint areas where your existing contractor can reduce waste. For instance, it can suggest changing the packaging design or the logistics process. You can share these insights with your supplier if they are open to eco-friendly practices. This way, you can still hit sustainability targets without terminating your supplier partnership.
Real-life example:
Companies rely on generative AI algorithms to spot unsustainable and unethical practices within their supply chain. For example, Siemens and Unilever use this approach to identify suppliers connected to the repression of Uyghur Muslims mentioned in the introduction.
Another example comes from the California-based Frenzy AI, which built a generative AI model that analyzes data, such as customs declarations and shipping documents, to trace products back to different suppliers and check if their own products are used ethically.
Use case 4: Anticipating customers’ needs
Generative AI models can process different types of data like historical sales, seasonal trends, economic data, competitor activities, customer sentiment, etc. to forecast demand. The algorithms can monitor all this in real time, informing you of upcoming trends as soon as they emerge. Generative AI can accomplish the following tasks:
-
Predict demand for different products and services, allowing companies to notify their suppliers, restock, and serve customers better
-
Model different scenarios of how demand can change so that companies can prepare. For example, it can show how altering pricing and marketing strategies impact demand.
Real-life example:
Walmart relies on a generative AI-based demand forecasting system to anticipate what customers will need at each individual store. Also, the retail giant uses the technology to analyze customer behavior during Black Friday events and foresee any potential bottlenecks.
Use case 5: Finding the right supplier and negotiating with them
As it can analyze large amounts of supply chain data, generative AI can give valuable recommendations and assist in supplier vetting. Here is what the technology can do:
-
Ranking suppliers. Algorithms can rank suppliers based on predefined criteria, such as pricing and quality of raw materials.
-
Evaluating sustainability practices. This includes assessing the potential supplier’s environmental footprint, social responsibility, waste production, and more.
-
Assessing risks associated with each supplier like geopolitical risks, economic factors, and other vulnerabilities
-
Generating contract negotiation strategies tailored to each supplier
Also, AI algorithms can continue monitoring partner suppliers to make sure they live up to their contractual obligations and maintain the expected quality levels.
Real-life example:
Walmart experimented with a generative AI bot from Pactum AI that can negotiate deals with suppliers. This approach helped the retailer save around 3% on contract expenses. Surprisingly (or not), three out of four suppliers actually preferred to negotiate with the bot.
You can find more generative AI use cases for business on our blog.
Challenges you might encounter with generative AI in supply chain management
If you are interested in implementing generative AI, be prepared to face the following set of challenges:
Data-related issues
Generative AI models need large amounts of quality data to do their job. If the data is fragmented, incomplete, and outdated, the results will not be accurate. And you can’t control which kind of supplier data is publicly available, so try to set reasonable expectations when you rely on data offered by others.
The models will also operate on your organizational data, such as history of sales, financial stats, and more. This is the data that you can control, so make sure it’s clean, free of bias, and accessible.
Integration with existing systems
Your new AI solution needs to seamlessly fit into the existing system and connect to other applications to access their data. This might involve adapting legacy systems, which is an enormous challenge. And you might have to re-engineer some of your processes. Also, generative AI is very powerful and requires considerable computational resources and data storage capacity. Consider adapting your infrastructure or arranging for cloud hosting.
Additionally, you will probably need to integrate with your suppliers’ software and align with their workflows. Check if they have a dedicated API that you can use for data collection.
Challenges associated with AI usage
-
Sometimes, lack of AI explainability can be an issue. It’s not always possible to explain why generative AI produced this response/suggestion/strategy. Take compliance reports as an example. If an organization wants to obtain ISO certification, it needs to document its processes to display compliance. But if it relies too much on generative AI for reporting, it might not be able to do so.
-
You need to comply with your industry standards for using AI and the general privacy regulations in your area of operations
Post-deployment issues
-
Security. Any AI system has to be designed with security in mind, as it works with large amounts of sensitive information. There is a set of practices that companies need to follow to ensure data security. This includes encrypting data during transfer and at rest, implementing authentication mechanisms, and monitoring for unauthorized access, to mention a few. Also, you will need to share data with your supplier network. Make sure this is secure as well.
-
Maintenance. AI models require regular audits, performance evaluations, and updates to remain efficient and relevant.
The human factor
After deploying generative AI for supply chain management, you want employees to accept it, use it, and contribute to its improvement. It’s best to formalize rules that will govern human-AI collaboration and specify who is responsible for the final results. And this is a challenge. Who is to blame if the inventory was flooded with products that nobody wanted to buy? And who is responsible if the supplier selected by AI fails to deliver on time twice in a row?
The company is also responsible for training its employees to work with AI and follow secure data practices.
Convinced that you need generative AI? Here’s what to do next
Here are nine tips that will help you get started with AI implementation:
-
Define your business objectives and what you want to achieve with generative AI for a supply chain. This will determine which data your models need to access.
-
Consider automated data collection so that your algorithms have access to up-to-date information
-
Prepare your data to be used by AI and ML algorithms
-
Make sure you obtain consent for using the training data when needed and comply with data privacy regulations
-
Establish strong data governance practices or tap into third-party data management services
-
Foster data collaboration between your organization and suppliers
-
Hire a reliable AI vendor to build or customize generative AI algorithms that can address your unique needs
-
Start with a small-scale pilot project and learn from failures
-
Monitor the models after deployment. Implement a feedback loop that allows users to report their concerns and recommendations.
If you are wondering about the costs associated with AI implementation, check out our detailed article on how much artificial intelligence costs.
Get in touch if you have any questions regarding the use of generative AI for supply chain management or want an accurate cost estimate for your Gen AI project. We will help you build/customize AI models, support you in data collection and cleaning, and audit your models on demand.