Can generative AI really transform drug discovery as we know it?
Gen AI has the potential to revolutionize the traditional drug discovery process in terms of speed, costs, the ability to test multiple hypotheses, discovering tailored drug candidates, and more. Just take a look at the table below.
Traditional drug discovery | Generative AI-powered drug discovery | |
---|---|---|
Process |
Sequential |
Iterative |
Effort |
Labour intensive. Researchers design experiments manually and test compounds through a lengthy trial process. |
Data-driven and automated. Algorithms generate drug molecules, compose trial protocols, and predict success during trials. |
Timeline |
Time consuming. Normally, it takes years. |
Fast and automated. It can take only one third of the time needed with the traditional approach. |
Cost |
Very expensive. Can cost billions. |
Much cheaper. The same results can be achieved with one-tenth of the cost. |
Data integration |
Limited to experimental data and known compounds |
Uses extensive data sets on genomics, chemical compounds, clinical data, literature, and more. |
Target selection |
Exploration is limited. Only known, predetermined targets are used. |
Can select several alternative targets for experimentation |
Personalization |
Limited. This approach looks for a drug suitable for a broader population. |
High personalization. With the help of patient data, such as biomarkers, Gen AI models can focus on tailored drug candidates |
The table above highlights the considerable promise of Gen AI for companies involved in drug discovery. But what about traditional artificial intelligence that reduces drug discovery costs by up to 70% and helps make better-informed decisions on drugs’ efficacy and safety? In real-world applications, how do the two types of AI stack up against each other?
While classic AI focuses on data analysis, pattern identification, and other similar tasks, Gen AI strives for creativity. It trains on vast datasets to produce brand new content. In the context of drug discovery, it can generate new molecule structures, simulate interactions between compounds, and more.
Benefits of Gen AI for drug discovery
Generative AI plays an important role in facilitating drug discovery. McKinsey analysts expect the technology to add around $15-28 billion annually to the research and early discovery phase.
Here are the key benefits that Gen AI brings to the field:
-
Accelerating the process of drug discovery. Insilico Medicine, a biotech company based in Hong Kong, has recently presented its pan-fibrotic inhibitor, INS018_055, the first drug discovered and designed with Gen AI. The medication moved to Phase 1 trials in less than 30 months. The traditional drug discovery process would take double this time.
-
Slashing down expenses. Traditional drug discovery and development are rather expensive. The average R&D expenditure for a large pharmaceutical company is estimated at $6.16 billion per drug. The aforementioned Insilico Medicine advanced its INS018_055 to Phase 2 clinical trials, spending only one-tenth of the amount it would take with the traditional method.
-
Enabling customization. Gen AI models can study the genetic makeup to determine how individual patients will react to select drugs. They can also identify biomarkers indicating disease stage and severity to consider these factors during drug discovery.
-
Predicting drug success at clinical trials. Around 90% of drugs fail clinical trials. It would be cheaper and more efficient to avoid taking each drug candidate there. Insilico Medicine, leaders in Gen AI-driven drug development, built a generative AI tool named inClinico that can predict clinical trial outcomes for different novel drugs. Over a seven-year study, this tool demonstrated 79% prediction accuracy compared to clinical trial results.
-
Overcoming data limitations. High-quality data is scarce in the healthcare and pharma domains, and it’s not always possible to use the available data due to privacy concerns. Generative AI in drug discovery can train on the existing data and synthesize realistic data points to train further and improve model accuracy.
The role of generative AI in drug discovery
Gen AI has five key applications in drug discovery:
-
Molecule and compound generation
-
Biomarker identification
-
Drug-target interaction prediction
-
Drug repurposing and combination
-
Drug side effects prediction

Molecule and compound generation
The most common use of generative AI in drug discovery is in molecule and compound generation. Gen AI models can:
-
Generate novel, valid molecules optimized for a specific purpose. Gen AI algorithms can train on 3D shapes of molecules and their characteristics to produce novel molecules with the desired properties, such as binding to a specific receptor.
-
Perform multi-objective molecule optimization. Models that are trained on chemical reactions data can predict interactions between chemical compounds and propose changes to molecule properties that will balance their profile in terms of synthetic feasibility, potency, safety, and other factors.
-
Screen compounds. Gen AI in drug discovery can not only produce a large set of virtual compounds but also help researchers evaluate them against biological targets and find the optimal fit.
Inspiring real-life examples:
-
Insilico Medicine used generative AI to come up with ISM6331—a molecule that can target advanced solid tumors. During this experiment, the AI model generated more than 6,000 potential molecules that were all screened to identify the most promising candidates. The winning ISM6331 shows promise as a pan-TEAD inhibitor against TEAD proteins that tumors need to progress and resist drugs. In preclinical studies, ISM6331 proved to be very efficient and safe for consumption.
-
Adaptyv Bio, a biotech startup based in Switzerland, relies on generative AI for protein engineering. But they don’t stop at just producing viable protein designs. The company has a protein engineering workcell where scientists, together with AI, write experimental protocols and produce the proteins designed by algorithms.
Biomarker identification
Biomarkers are molecules that subtly indicate certain processes in the human body. Some biomarkers point to normal biological processes, and some signal the presence of a disease and reflect its severity.
In drug discovery, biomarkers are mostly used to identify potential therapeutic targets for personalized drugs. They can also help select the optimal patient population for clinical trials. People that share the same biomarkers have similar characteristics and are at similar stages of the disease that manifests in similar ways. In other words, this enables the discovery of highly personalized drugs.
In this aspect of drug discovery, the role of generative AI is to study vast genomic and proteomic datasets to identify promising biomarkers corresponding to different diseases and then look for these indicators in patients. Algorithms can identify biomarkers in medical images, such as MRIs and CAT scans, and other types of patient data.
A real-life example of generative AI in drug discovery:
The hyperactive in this field, Insilico Medicine, built a Gen AI-powered target identification tool, PandaOmics. Researchers thoroughly tested this solution for biomarker discovery and identified biomarkers associated with gallbladder cancer and androgenic alopecia, among others.
Drug-target interaction prediction
Generative AI models learn from drug structures, gene expression profiles, and known drug-target interactions to simulate molecule interactions and predict the binding affinity of new drug compounds and their protein targets.
Gen AI can rapidly run target proteins against enormous libraries of chemical compounds to find any existing molecules that can bind to the target. If nothing is found, they can generate novel compounds and test their ligand-receptor interaction strength.
A real-life example of generative AI in drug discovery:
Researchers from MIT and Tufts University came up with a novel approach to evaluating drug-target interactions using ConPLex, a large language model. One incredible advantage of this Gen AI algorithm is that it can run candidate drug molecules against the target protein without having to calculate the molecule structure, screening over 100 million compounds in one day. Another important feature of ConPLex is that it can eliminate decoy elements—imposter compounds that are very similar to an actual drug but can’t interact with the target.
During an experiment, scientists used this Gen AI algorithm on 4,700 candidate molecules to test their binding affinity to a set of protein kinases. ConPLex identifies 19 promising drug-target pairs. The research team tested these results and found that 12 of them have immensely strong binding potential. So strong that even a tiny amount of drug can inhibit the target protein.
Drug repurposing and combining
Gen AI algorithms can look for new therapeutic applications of existing, approved drugs. Reusing existing drugs is much faster than resorting to the traditional drug development approach. Also, these drugs were already tested and have an established safety profile.
In addition to repurposing a single drug, generative AI in drug discovery can predict which drug combinations can be effective for treating a disorder.
Real-life examples:
-
A team of researchers experimented with using Gen AI to find drug candidates for Alzheimer’s disease through repurposing. The model identified twenty promising drugs. The scientists tested the top ten candidates on patients over the age of 65. Three of the drug candidates, namely metformin, losartan, and simvastatin, were associated with lower Alzheimer’s risks.
-
Researchers at IBM evaluated the potential of Gen AI for finding drugs that can be repurposed to address the type of dementia that tends to accompany Parkinson’s disease. Their models worked on the IBM Watson Health data and simulated different cohorts of individuals who did and didn’t take the candidate drug. They also considered differences in gender, comorbidities, and other relevant attributes.
The algorithm suggested repurposing rasagiline, an existing Parkinson’s medication, and zolpidem, which is used to ease insomnia.
Drug side effects prediction
Gen AI models can aggregate data and simulate molecule interactions to predict potential side effects and the likelihood of their occurrence, allowing scientists to opt for the safest candidates. Here is how Gen AI does that.
-
Predicting chemical structures. Generative AI in drug discovery can analyze novel molecule structures and forecast their properties and chemical reactivity. Some structural features are historically associated with adverse reactions.
-
Analyzing biological pathways. These models can determine which biological processes can be affected by the drug molecule. As molecules interact in a cell, they can create byproducts or result in cell changes.
-
Integrating Omics data. Gen AI can refer to genomic, proteomic, and other types of Omics data to “understand” how different genetic makeups can respond to the candidate drug.
-
Predicting adverse events. These algorithms can study historic drug-adverse event associations to forecast potential side effects.
-
Detecting toxicity. Drug molecules can bind to non-target proteins, which can lead to toxicity. By analyzing drug-protein interactions, Gen AI models can predict such events and their consequences.
Real-life example:
Scientists from Stanford and McMaster University combined generative AI and drug discovery to produce molecules that can fight Acinetobacter baumannii. This is an antibiotic-resistant bacteria that causes deadly diseases, such as meningitis and pneumonia. Their Gen AI model learned from a database of 132,000 molecule fragments and 13 chemical reactions to produce billions of candidates. Then another AI algorithm screened the set for binding abilities and side effects, including toxicity, identifying six promising candidates.
Want to find out more about AI in pharma? Check out our blog. It contains insightful articles on:
Still curious about the transformative potential of Gen AI and how it can elevate your business strategies? Dive into our latest eBook, Generative AI for Business Leaders, meticulously crafted to empower forward-thinking professionals like yourself. Whether you’re an industry veteran or an entrepreneur, this comprehensive guide equips you with invaluable insights to revolutionize your operations and spur innovation.
Don’t miss out—enter your corporate email address to claim your free copy today!
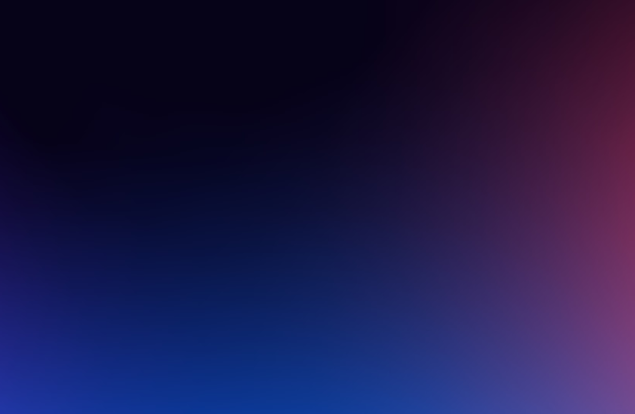
Challenges of using Gen AI in drug discovery
Gen AI plays an important role in drug discovery. But it also presents considerable challenges that you need to prepare for. Discover what issues you may encounter during Gen AI deployment and how our generative AI consulting company can help you navigate them.
Challenge 1: Lack of model explainability
Generative AI models are typically built as black boxes. They don’t offer any explanation of how they work. But in many cases, researchers need to know why the model makes specific recommendation. For example, if the model says that this drug is not toxic, scientists need to understand its line of reasoning.
How ITRex can help:
As an experienced pharma software development company, we can follow the principles of explainable AI to prioritize transparency and interpretability. We can also incorporate intuitive visualization tools that use molecular fingerprints and other techniques to explain how Gen AI tools reach a conclusion.
Challenge 2: Model hallucination and inaccuracy
Gen AI models, such as ChatGPT, can confidently present you with information that is plausible but yet inaccurate. In drug discovery, this translates into molecule structures that researchers can’t replicate in real life, which isn’t that dangerous. But these models can also claim that interactions between certain compounds don’t generate toxic byproducts, when this is not the case.
How ITRex can help:
It’s not possible to eliminate hallucinations altogether. Researchers and field experts are experimenting with different solutions. Some believe that using more precise prompting techniques can help. Asif Hasan, co-founder of Quantiphi, an AI-first digital engineering company, says that users need to “ground their prompts in facts that are related to the question.” While others call for deploying Gen AI architectures specifically designed to produce more realistic outputs, such as generative adversarial networks.
Whatever option you want to use, it will not eradicate hallucination. What we can do is remember that this challenge exists and make sure that Gen AI doesn’t have the final say in aspects that directly affect people’s health. Our team can help you base your Gen AI in drug discovery workflow on a human-in-the-loop approach to automatically include expert verification in sensitive cases.
Challenge 3: Bias and limited generalization
Gen AI models that were trained on biased and incomplete data will reflect this in their results. For example, if an algorithm is trained on a dataset with one predominant type of molecule properties, it will keep producing similar molecules, lacking diversity. It won’t be able to generate anything in the underrepresented chemical space.
How ITRex can help:
If you contact us to train or retrain your Gen AI algorithms, we will work with you to evaluate the training dataset and ensure it’s representative of the chemical space of interest. If dataset size is a concern, we can use generative AI in drug discovery to synthesize training data. Our team will also screen the model’s output during training for any signs of discrimination and adjust the dataset if needed.
Challenge 4: The uniqueness of chemical space
The chemical compound space is vast and multidimensional, and a general-purpose Gen AI model will struggle while exploring it. Some models resort to shortcuts, such as relying on 2D molecule structure to speed up computation. However, research shows that 2D models don’t offer a faithful representation of real-world molecules, which will reduce outcome accuracy.
How ITRex can help:
Our biotech software development company can implement dedicated techniques to help Gen AI models adapt to the complexity of chemical space. These techniques include:
-
Dimensionality reduction. We can build algorithms that enable researchers to cluster chemical space and identify regions of interest that Gen AI models can focus on.
-
Diversity sampling. Chemical space is not uniform. Some clusters are heavily populated with similar compounds, and it’s tempting to just capture molecules from there. We will ensure that Gen AI models explore the space uniformly without getting stuck on these clusters.
Challenge 5: High infrastructure and computational costs
Building a Gen AI model from scratch is excessively expensive. A more realistic alternative is to retrain an open-source or commercial solution. But even then, the expenses associated with computational power and infrastructure remain high. For example, if you want to customize a moderately large Gen AI model like GPT-2, expect to spend $80,000–$190,000 on hardware, implementation, and data preparation during the initial deployment. You will also incur $5,000–$15,000 in recurring maintenance costs. And if you are retraining a commercially available model, you will also have to pay licensing fees.
How ITRex can help:
Using generative AI models for drug discovery is expensive. There is no way around that. But we can work with you to make sure you don’t spend on features that you don’t need. We can look for open-source options and use pre-trained algorithms that just need fine-tuning. For example, we can work with Gen AI models already trained on general molecule datasets and retrain them on more specialized sets. We can also investigate the potential of using secure cloud options for computational power instead of relying on in-house servers.
To sum it up
Deploying generative AI in drug discovery will help you accomplish the task faster and cheaper while producing a more effective and tailored candidate drugs.
However, selecting the right Gen AI model accounts for only 15% of the effort. You need to integrate it correctly in your complex workflows and give it access to data. Here is where we come in. With our experience in Gen AI development, ITRex will help you train the model, streamline integration, and manage your data in a compliant and secure manner. Just give us a call!