Generative AI use cases in pharma
Let’s clarify the terminology first.
Generative AI in pharma relies on deep learning models to study complex data, such as DNA sequences and other genomic data, drug compounds, proteomic data, clinical trial documentation, and more, to produce new content that is similar to what it studied.
Feel free to check out our blog to understand the difference between artificial intelligence and Gen AI, learn about generative AI’s pros and cons, and explore top generative AI use cases for businesses.
Now let’s explore the key five Gen AI use cases in the pharmaceutical industry.
1. Drug discovery, development, and repurposing
Recent studies point out that traditional artificial intelligence can expedite drug discovery and help save 25% to 50% of the associated time and costs. Generative AI holds an even bigger promise for the pharmaceutical industry, prompting more companies to build and deploy pharma software solutions involving Gen AI in the coming years.
Gen AI in drug discovery
On our blog, we have a dedicated article highlighting the potential of Gen AI in drug discovery. Here is a summary of a few key points:
-
De novo drug design. Pharmaceutical companies can train Gen AI models on enormous sets of molecular data to generate novel, previously unseen molecular structures with the desired properties.
-
Virtual screening. Gen AI algorithms can study different drug compounds and predict their interactions among each other to form a drug for a specific biological target. It can also modify a drug’s molecular structure to enhance its properties.
-
Interactions between drugs. Gen AI can predict how drugs will interact with each other, helping to discover the side effects of taking several drugs together.
Gen AI in drug development
-
Assistance in manufacturing. Generative AI for pharma can predict how different compounds and their concentrations will affect the drug’s performance, such as bioavailability, stability, and toxicity. It can also optimize the chemical processes involved in drug manufacturing and suggest optimal formulations.
-
Quality control. Gen AI can foresee any potential issues that can impact the drug’s quality. It can predict any impurities, deviations from specifications, and more, basically telling quality inspectors where to look during audits.
Gen AI in drug repurposing
These models can “study” drug compound databases and predict which other purposes a particular drug can serve given its efficacy for treating particular symptoms. The technology can also start with a disease or a biological target and look for existing drugs or chemical compounds that can be repurposed to treat it while identifying potential side effects. Finally, Gen AI can take an existing drug and suggest structure changes to modify the drug’s healing potential, enabling it to treat other diseases.
Real-life example:
Insilico Medicine, a biotech company based in Hong Kong, revealed the first drug discovered and designed by Gen AI—INS018_055—which they intend to use to treat idiopathic pulmonary fibrosis, a rare lung disease that results in lung scarring. INS018_055 progressed to Phase Ⅰ trials after only 30 months since the discovery, which is approximately half of what it takes with the traditional approach. This process would cost around $400 million with the classic drug discovery, but Insilico Medicine spent only 10% of the amount thanks to Gen AI. The Phase Ⅰ trials proved the drug was safe, and it progressed to Phase Ⅱ trials.
2. Clinical trials and research
Companies can deploy Gen AI in pharma to facilitate clinical trials in four key aspects: clinical trial design, research, dataset augmentation, and documentation generation.
Clinical trial design
Pharma generative AI can simulate different trial scenarios, such as how patients respond to treatment and how their response changes when adjusting the dosage. Algorithms can make changes in real-time as new data comes in. Additionally, Gen AI can simulate trial designs, including randomization methods, exclusion criteria, sample sizes, etc.
These algorithms can serve as virtual assistants that can respond to trial-related queries and give real-time updates on the number of registered patients, trial progress, and more.
Clinical research
Generative AI excels at multimodal data fusion as it looks into diverse datasets, including clinical data, drug databases, genomics, and more, giving researchers the opportunity to consider multiple rich data sources. AI can execute queries like searching for real-world evidence that can prove the drug is safe.
Dataset augmentation
Generative AI in pharma can synthesize patient data. It can produce realistic patient information, which researchers can use during trials before involving people. For clinical studies relying on medical imaging, Gen AI can generate realistic scans representing the medical condition to augment the training/testing datasets.
Documentation generation
The technology can create textual content with natural language generation (NLG). It can document protocols, create trial reports, generate regulatory compliance documentation, and more. This can reduce medical writing time by 30%.
Real-life examples:
Bayer Pharma uses generative AI to mine research data, produce first drafts of clinical trial communications, and translate them to different languages. Another example comes from Sanofi. The company relies on Gen AI to support its trial-related activities, such as setting up the site and boosting participation of underrepresented population segments.
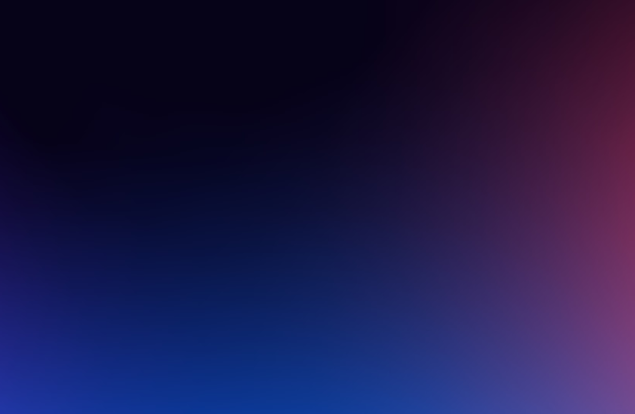
3. Personalized medicine
Here is how pharma generative AI can support personalized medicine and treatment plans tailored to individual patients:
-
Modeling how a disease can progress in a particular patient given their biological processes and how a specific illness will respond to the proposed drugs. This helps adjust the treatment by changing the dosage or suggesting a different path without waiting for the patient’s condition to deteriorate.
-
Building predictive models for patients based on their genetic makeup, including genetic variations, mutations, and biomarkers. These models can forecast different genetic diseases and other medical conditions and evaluate how various interventions, such as surgeries, diet, and lifestyle adjustments, can change the clinical picture.
Using Gen AI in personalized medicine is a novel idea, and we did not find any successful examples at the time of writing this article. But there are several research efforts in this direction. For instance, the aforementioned pioneer in AI-driven drug development, Insilico Medicine, is working on building a new model for drug discovery that will be based on identifying biological targets in individuals and then optimizing molecules to better inhibit those specific targets.
4. Marketing and patient engagement
Gen AI can support your marketing department by producing content that actually resonates with the audience and that is tailored to individual users and user groups. Here is how it works:
-
Generating marketing content. Generative AI in pharma can analyze existing marketing material, customer reviews, and current trends to compose articles, product descriptions, banner ads, video scripts, and other marketing text.
-
Enhancing advertising campaigns. Gen AI models can analyze historical data on previous campaigns and study the competition’s performance to produce new creative marketing campaigns and recommend adjustments to the existing ads. It can also generate several text versions for A/B testing and identify the best suited option.
-
Assisting with product positioning. Algorithms can study competitors’ offerings and how they interact with customers, along with market trends, to create captivating headlines, taglines, and narratives that will resonate with the target audience and make your products stand out from the competition.
-
Engaging customers through personalized messaging. Generative AI can study patients’ clinical pictures based on genetics, medical history, etc. and come up with personalized recommendations on exercise, diet, medical checkups, and more.
-
Managing social media. Gen AI-powered chatbots can interact with customers in real time, respond to their queries, and generate appropriate social media posts.
Real-life example:
Gramener, a data science and AI firm, built a Gen AI-powered solution for commercial pharma companies. It can generate promotional content, sales team support material, and more, while ensuring that the content is compliant with privacy regulations. The company claims their software can save up to 60% of the time spent on marketing tasks, resulting in quarterly savings of $200,000.
5. Inventory management and supply chain optimization
In its recent research, McKinsey reported that adopting AI-powered forecasting in supply chains can reduce lost sales by up to 65% while allowing companies to spend 10% less on warehousing and inventory expenses. Let’s see what Gen AI can do for the pharmaceutical sector.
-
Forecasting demand. Gen AI algorithms can analyze historical sales data and current trends to predict demand for different pharmaceutical products, allowing companies to optimize inventory levels and tune their production capacity accordingly.
-
Managing relationships with suppliers. Gen AI in pharma can process supplier performance data, including reliability, prices, etc., and suggest a list of potential suppliers. Afterwards, it can help with contract negotiations for favorable terms. The technology can also generate initial proposals and counteroffers, produce different contract versions, and simulate negotiation and risk scenarios. And during the negotiation process, it can offer real-time support by generating prompts as it analyzes conversation dynamics and prospective supplier’s sentiment.
-
Optimizing logistics. Gen AI can analyze delivery schedules, vehicle capacity, weather conditions, and other relevant data to propose route alternatives and even suggest real-time adjustments to a route plan of an ongoing delivery, enabling dynamic route optimization.
Real-life example:
A global pharmaceutical firm, Sanofi, deployed an AI-powered app that offers a 360-degree view of the company’s data in real time. The analytics supported by this app allowed Sanofi to forecast 80% of low inventory positions and take the corresponding actions.
Evaluating the impact of Gen AI in the pharma industry
Let’s take a look at the opportunities and challenges this technology brings.
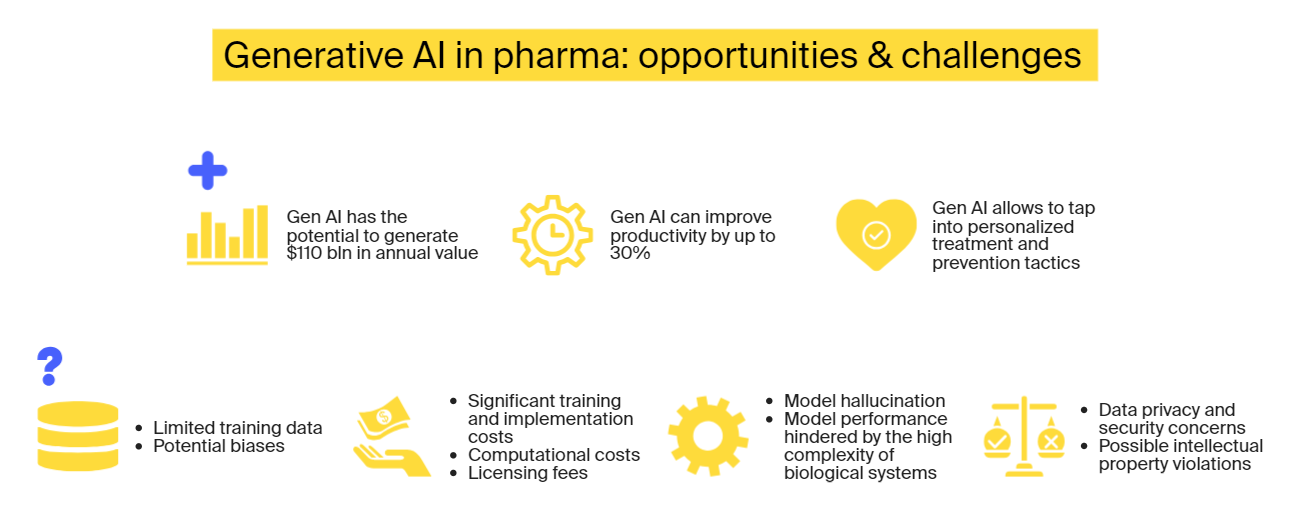
Opportunities for generative AI in pharma
Economic impact
McKinsey predicts that Gen AI can add up to $110 billion of annual economic value for the pharmaceutical sector. Here is how you can use Gen AI to cut down costs:
-
Expediting drug development by identifying compounds and biological targets much faster
-
Saving on clinical trials as companies can partially rely on Gen AI trial simulations
-
Repurposing existing drugs. Research suggests that repurposing generic drugs is 40-90% cheaper than discovering new compounds
Productivity
According to Boston Consulting Group, generative AI in pharma has the potential to bring 30% productivity improvement. And Accenture claims that the technology will impact 40% of life science work hours. Here is what Gen AI can do in this regard:
-
Generating clinical trial documentation and marketing material
-
Acting as personal assistant to support in research and clinical trial management
-
Generating sales scripts and assisting the sales team in real time
Health outcomes
Gen AI in pharma can largely improve health outcomes by developing personalized medicine that is tailored to particular patients. This approach will help pharmaceutical companies choose the right drug or a combination of drugs and minimize side effects.
Challenges that generative AI brings to pharmaceutic
-
Training dataset quality and availability. Gen AI models should be trained on large datasets for optimal performance. But in the pharmaceutical sector, training data is usually scarce. Estimates show that only 25% of health data is available for research. Luckily, Gen AI models can also be part of the solution as they can synthesize patient information.
-
Potential bias and discrimination. A model’s performance depends on the training dataset. If, let’s say, a marketing model was trained on data geared towards one population segment, this model could produce materials that are not suitable or even inappropriate for other cohorts. Also, if the model decides who can view ads, it can also discriminate against certain populations.
-
Hallucination. Gen AI algorithms can generate sound but incorrect results. For example, they can deliver protein structures that can’t be created in real life. And if you use such models as research assistants, they can give plausible but wrong answers. In yet another hallucination example, generative AI models for pharma can produce advertising material claiming that one drug is more effective or even safer than it actually is.
-
Complexity of biological systems. Gen AI models need to be comprehensive enough to understand the complexity of biological processes and the interactions between compounds at different levels. What complicates matters is that biological systems can have emergent properties, meaning that the behavior of the entire system can’t be predicted solely from properties of its individual components.
-
Infrastructure and computational resources. Gen AI models are large. They are expensive to train and run. So, it’s important to decide on the infrastructure that you want to use, whether it’s on premises with local servers or in the cloud. If you opt for on-premises deployment, you are likely to pay up to $30,000 in GPU costs. Also, if you decide to run the model on local infrastructure, make sure that everything else will still work under this additional load. If you go with a cloud provider, your computing expenses alone can range from $10-24 per hour. And these are not the only costs involved.
-
Privacy and ethical considerations. Pharmaceutical firms are dealing with sensitive patient information and need to comply with their local standards and privacy regulations. Pharma needs to implement robust consent practices, access control, and other security measures when letting Gen AI models use and train on personal information, like genomic data and patient medical history. Lack of formal regulations governing data usage aggravates this concern.
Another ethical issue is intellectual property. If you use a ready-made Gen AI model that you don’t own for drug development, how do you manage the intellectual property for this drug?
Wrapping up
Gen AI in pharma can revolutionize drug development, testing, and marketing. But the technology can have dire consequences if not used carefully.
Get in touch if you want to balance the risks and the outstanding benefits generative AI brings to the pharmaceutical sector. To offset the risks, we can help you implement a human-in-the-loop approach where people participate in AI training and make adjustments to the model. We can also look into explainable AI if needed.
In general, our AI consultants can help you find the right Gen AI model that fits your needs without spending more than you need in computing power and costs. We will retrain the model on your dataset, integrate it into your system, and offer maintenance and support.
Based on our experience in building AI solutions for healthcare, we have written several articles that might help you gain ideas for new projects or just better understand the technology: