What is predictive analytics in healthcare?
Deloitte describes predictive analytics as a branch of advanced data analytics used to make predictions about unknown future activities.
Unlike traditional approaches to analytics that simply present processed information from the past, predictive analytics uses historical and real-time data to forecast future events and identify trends in patient care. To achieve this, predictive analytics employs a variety of techniques, including data mining, statistics, artificial intelligence, and machine learning.
Simply put, predictive analytics solutions answer the question: what can possibly happen next?
How does predictive analytics work in the healthcare sector?
Healthcare predictive analytics aggregates vast amounts of patient data incoming from electronic health records (EHR), insurance claims, administrative paperwork, medical imaging, etc. and processes it searching for patterns. With predictive analytics, healthcare providers get to answer the following questions:
-
Which diseases patients are likely to develop?
-
How will they respond to different treatments?
-
Will they be a no-show in their next medical appointment?
-
Will they return to the hospital within 30 days of discharge?
-
And more
Benefits of predictive analytics in healthcare
Here are some examples of how healthcare leaders can benefit from predictive analytics:
-
Reducing costs on appointment no-shows and readmission penalties
-
Speeding up administrative tasks such as discharge procedures and insurance claims submission
-
Preventing ransom and other cyberattacks by analyzing ongoing transactions and assigning risk scores
-
Proactively preparing for upcoming population health trends
-
Attracting new patients through personalized campaigns
7 examples of predictive analytics in healthcare
Predictive analytics is transforming the medical field in multiple ways. Here are seven exciting examples of predictive analytics in the healthcare sector:
-
Preventing readmissions
-
Managing population health
-
Enhancing cybersecurity
-
Predicting disease onsets
-
Speeding up insurance claims submission and processing
-
Predicting suicide attempts
-
Forecasting appointment no-shows
1. Preventing readmission
The costs of hospital readmission are rather high. The US healthcare system spends around $52.4 billion on readmissions per year. Additionally, hospitals are subject to heavy penalties under Medicare’s Hospital Readmission Reduction Program, which adds financial incentives to combat readmission. As research shows, 82% of hospitals in this program received this type of penalty.
Healthcare predictive analytics can identify patients with traits indicating a high possibility of readmission, so that doctors can allocate additional resources for follow-ups and personalize discharge protocols to prevent a quick turnaround.
Real-life example:
A research team at Corewell Health deployed AI and predictive analytics in healthcare to identify patients with a high probability of readmission. The researchers studied the patients who had a hard time recovering after hospitalization and devised a recovery plan.
This plan had three pillars: behavioral health, clinical challenges, and social determinants of health. As soon as the predictive analytics tool spotted a potential candidate for readmission, an interdisciplinary team worked with the person to address those three aspects. This approach enabled Corewell Health to prevent the readmission of 200 patients and save $5 million in associated costs.
2. Managing population health
This is another prominent healthcare predictive analytics example, which covers two aspects.
Identifying public health trends with predictive analytics
Predictive analytics in the healthcare industry helps identify potential population health trends. The Lancet Public Health journal published a study that used predictive analytics to uncover health trends. This study found that unless alcohol consumption patterns change in the US, alcohol-related liver diseases will rise, causing deaths.
Detecting disease outbreaks with predictive healthcare analytics
When speaking of outbreak predictions, one can’t help but ask, “Could predictive analytics have foreseen the COVID-19 pandemic?” The answer is yes. BlueDot, a Canadian company building predictive analytics and AI solutions, issued a warning about the rise of unfamiliar pneumonia cases in Wuhan on December 30, 2019. Only nine days later, the World Health Organization released an official statement declaring the emergence of the novel coronavirus.
3. Enhancing cybersecurity
According to the HIPAA Healthcare Data Breach Report, cyberattacks are frequent in the healthcare sector. The report revealed that between January and October 2023, over 82.6 million healthcare records were exposed, and hacking was the most common cause of these breaches.
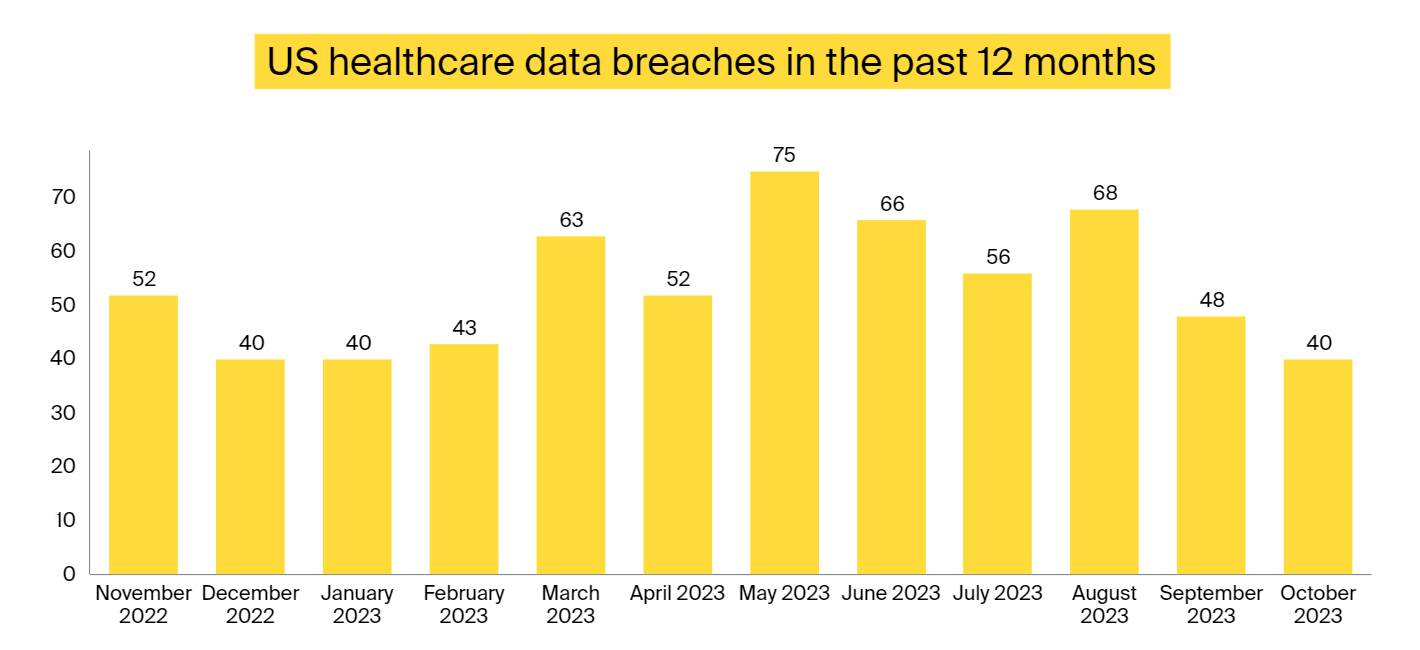
Cybersecurity predictive analytics in healthcare can positively contribute to this situation. Healthcare organizations can use predictive analytics coupled with artificial intelligence solutions for the medical sector to calculate risk scores for different online transactions in real-time and respond to events based on their scores.
For example, during a login event, the system will grant access to a low-risk process, and block a high-risk process or challenge it for multi-factor authentication. Furthermore, predictive modeling in healthcare can monitor data access and sharing to identify any changes in patterns, which could indicate an intrusion.
Predictive analytics in cybersecurity comes in two high-level types. Each has many subtypes of its own:
-
Vulnerability-based solutions are searching for weaknesses in the healthcare system that can be exploited. These vulnerabilities range from misconfigurations to Common Vulnerabilities and Exposures (CVEs) that were not patched.
-
Threat-focused platforms that look for potential threats.
Saif Abed, CEO of Clinical Cyber Defense Systems, advises healthcare clinics to consider outcome-based variants of both types. Here is what he said, “I recommend healthcare providers focus on outcomes-based analytics: solutions that can correlate technical findings with actual clinical and business metrics. If a solution can chart, forecast and predict whether patient harm will happen or whether services will be shut down, then it’s a solution able to capture the attention of hospital executives at the highest levels irrespective – of their technical acumen.”
4. Predicting disease onsets
Predictive analytics with machine learning can forecast diseases when patients don’t show any visible signs or even tendencies for onsets.
Real-life examples:
A research team experimented with predictive analytics models in healthcare to identify patients with early onsets of diabetes. The scientists used medical data from Hospital de Santa Luzia and found that it was possible to build a reliable ML model using patient profiles and the prescribed medication the person has been consuming.
In another example, a research team built an ML-powered tool that can predict multiple myeloma. This solution takes into account tumor genomics and the prescribed treatment. During the course of this project, researchers identified 90 genes that can be present in a tumor and have a high potential for mutation. With this knowledge, the tool could predict 12 different types of multiple myeloma.
In yet another research project, the University of Massachusetts just recently started its initiative to build a deep learning model that can predict Alzheimer’s as early as two years before the first symptoms start showing.
You can find more information on similar experiments in our ML in bioinformatics article.
5. Speeding up insurance claims submission and processing
Predictive analytics in healthcare can spot insurance claims that are likely to be rejected based on historical data and rejection patterns. The technology can also detect insufficient reimbursement codes and suggest matching fields. Such tools can help hospitals prepare insurance claims faster while minimizing errors.
Real-life example:
Headquartered in California, Apixio builds analytics-powered tools for healthcare. The company developed software that helps hospital coders identify the correct codes for insurance claims.
Traditionally, hospital coders sift through vast amounts of information to find the right codes. Apixio’s tool mines patient medical records for relevant data and presents the coders with the selected pieces that will help them determine the best code options.
6. Predicting suicide attempts
Suicide is the tenth leading cause of death in the US, taking lives of 14 Americans out of 100,000 annually. Predictive analytics tools for healthcare can factor in a patient’s previous attempts, medical notes, and social and economic conditions to determine the likelihood of future attempts.
Real-life examples:
A research team at the Vanderbilt University Medial Center (VUMC) developed a predictive analytics model that uses patients’ electronic health records to forecast the likelihood of suicide attempts by particular patients. The tool was tested at VUMC for 11 months. While doctors were receiving patients, the algorithm was running in the background, predicting the risk of patients returning for attempted suicide treatment.
This predictive analytics in healthcare tool classified patients into 8 groups based on their estimated risk factors. The VUMC research team noticed that the highest-risk group accounted for over 33% of all suicide attempts. The team concluded that anyone who is placed into the high-risk groups should be checked for suicidal tendencies.
In another example, scientists applied predictive analytics in healthcare to detect suicide ideation and suicide attempts in South Korean adults. They achieved high accuracy when analyzing factors such as income, self-esteem, and depressive symptoms.
7. Forecasting appointment no-shows
The US healthcare system is losing about $150 billion per year on appointment no-shows, not to mention the associated administrative burden. For individual practitioners, the costs are averaging $200 per missed appointment. Predictive analytics in healthcare can improve cost efficiency by allowing hospitals and private clinics to forecast which patients are likely to skip appointments without prior notice. Consequently, they can prevent revenue loss and increase provider satisfaction.
Real-life example:
A team of researchers cooperated with Doctor Luis Calvo Mackenna Hospital, a pediatric facility in Chile, which reported a high no-show rate of 29%. The researchers used healthcare predictive analytics and ML to process patient data, such as demographics and social conditions, to spot patients prone to no-shows and call them with a reminder. During the eight-week experiment, the researchers managed to reduce no-shows by 10.3%.
Challenges associated with healthcare predictive analytics
Predictive analytics in healthcare brings its risks that medical facilities need to address before unlocking the tools’ full potential.
-
Gaining doctors’ acceptance. Healthcare providers are finding themselves in constant need to advance their computer skills. And with predictive analytics, they will not only need to access dashboards but also keep capturing and processing patient data. It can be a challenge to find the balance between patient care and data collection during appointments.
To overcome this challenge, medical facilities can involve their staff in the development of predictive analytics tools in healthcare and incorporate their feedback.
As Oscar Marroquin, Chief Clinical Analytics Officer at the University of Pittsburgh Medical Center, stated, “Being able to win acceptance of your models by clinicians—who are skeptics by nature—is often a challenge. By making end-users part of the whole process, we’ve been able to overcome that in the majority of cases.
-
Ethics and moral hazards. People are more likely to engage in risky behavior when they know someone’s got them covered. This is what we observe in healthcare settings where some doctors may rely excessively on predictive analytics for decision-making, under the assumption that these tools bear the responsibility for outcomes.
To mitigate this risk, it’s crucial to emphasize that decisions made by analytical tools are not definitive mandates but rather recommendations. Clinicians must critically evaluate these suggestions and, if needed, involve patients in the decision-making process.
-
Algorithm bias and lack of regulations. There are several types of algorithmic bias that can affect a model’s performance on particular datasets. What makes things harder is that there are no explicit regulations governing algorithm development. At the moment, the responsibility of producing fair tools falls on the vendor’s shoulders.
To reduce the risk of bias, vendors can use feedback loops to improve their tools and eliminate any bias that still gets in. Also, organizations that are using predictive analytics in healthcare need to conduct regular audits to make sure the algorithms are still relevant and bias-free.
-
Model explainability. Many powerful healthcare predictive analytics models have a black-box architecture, meaning they don’t explain how they produce their output. This approach is acceptable in administrative matters, such as predicting which insurance claims will be declined. But doctors will not take any recommendation that affects patients’ wellbeing without understanding the motives.
As a solution, clinics can look into explainable AI to cover the use cases directly impacting people’s health.
On a final note
The global predictive analytics in healthcare market was valued at $11.7 billion in 2022 and is expected to grow at a CAGR of 24.4% from 2023 to 2030. It provides plenty of opportunities for healthcare providers and health tech companies. If you are a medical facility manager, search for ways to deploy predictive analytics at your organization and ensure doctors and executives are on board. If you are a health tech startup founder, make sure the algorithms you develop are fair and suitable for the targeted population segments.
And don’t hesitate to get in touch if you need any assistance. We, at ITRex, have vast experience in AI, ML, and data analytics and will be happy to help you implement and deploy digital healthcare solutions. Moreover, we can share established practices on data management, automated data collection, and how to prepare your data for AI models.