What is an AI proof of concept, and when is it essential for your project’s success?
An artificial intelligence proof of concept is a prototype or a demonstration of a proposed AI solution designed to test whether the solution is feasible and likely to be successful.
The purpose of creating an AI PoC is to validate the concept, assess the proposed solution’s potential benefits, and identify any potential challenges or limitations.
An AI PoC typically involves building a small-scale version of the proposed AI solution and testing it in a controlled environment to see how it performs and whether it meets the desired objectives. The results can then be used to inform further development and implementation of the solution.
Compared to ordinary software PoCs, AI PoCs may involve more complex considerations, such as the ability of the AI solution to learn and adapt over time and the potential ethical implications of the solution, such as AI bias.
The technology stack for AI proof of concept projects is different, too:
-
Machine learning algorithms. These algorithms allow the AI system to learn from structured data and make predictions or decisions based on that learning. There are many different types of machine learning algorithms, including supervised learning, unsupervised learning, and reinforcement learning algorithms.
-
Neural networks. These computational models are inspired by the structure and function of the human brain. Neural networks can process and analyze large amounts of unstructured data. They can learn to perform various tasks, such as image recognition, natural language processing, scenario modeling, and prediction.
-
Robotics. This technology can be used to build physical systems capable of autonomous operation and decision-making. Robotics solutions incorporate sensors, actuators, and other hardware components that allow engineers to build a robot that can interact with its environment and perform tasks.
-
Cloud computing. Cloud computing platforms like Microsoft Azure, Google Cloud, and AWS provide the computing power, storage resources, and pre-configured services needed to support the development and testing of AI PoCs. These platforms can also host and deploy AI solutions once they have been developed and tested.
When is there absolutely no way around the AI PoC?
It’s essential to start your project with a proof of concept in the following scenarios:
-
Your project relies on an innovative idea that was not tested before. Something that was studied at the business level but not attempted technically. Neither you nor your tech vendor are confident if this can be implemented at all.
-
If you need to demonstrate the feasibility of your idea to stakeholders or investors within a limited timeframe. A PoC will do the job better than an interactive prototype or something similar.
Are there situations where an AI PoC is a waste of time?
Even though an AI proof of concept is beneficial in most cases, there are a few exceptions. If your project falls under the following categories, PoC can be an overkill:
-
If your idea and approach are exceptionally well documented from the functional and technical perspectives. Such instances are rather rare.
-
If the solution you want to develop is standard and resembles common practices in the field. In this scenario, your development team already knows that your idea is feasible and possible to implement from a technical perspective.
-
If you want to build software that your front-end and back-end developers understand and have already worked on something identical before
Which benefits can you gain from AI proof of concept?
Using AI proof of concept brings about the following benefits:
-
Identifying potential challenges before committing more resources to this endeavor. PoC AI allows you to “fail fast, fail better.” If the development team encounters challenges they can’t overcome, all stakeholders will have time to regroup or maybe change the hypothesis to reach the same goals through other methods.
-
Minimizing business risks as you test innovative ideas in small steps instead of diving into a long-term project
-
Getting investors and other stakeholders on board
-
Saving time and resources. AI PoC might uncover business or process-related issues and give you time to fix everything before committing to a full-scale project.
-
Building expertise and creating knowledge owners who will mentor other team member on similar projects in the future
-
Testing the tech stack on a smaller scale to understand its suitability for the selected business case
Examples from our portfolio where AI PoC saved the day
Here are a few AI PoC examples from the ITRex portfolio that will help you appreciate the proof of concept approach even more.
Realizing that ML alone is not the answer
A large cargo logistics company performs 10,000–15,000 shipments per day, and every shipment is accompanied by bills of lading and invoices to cover the operations. Employees were exhausted by handling all the documentation manually. The company wanted to build an ML-powered solution that would use optical character recognition (OCR) to process scanned documents and identify different fields.
The client believed that machine learning was the best choice for this case, so we proceeded with an AI PoC to test this assumption. Soon we realized that the documents were formatted differently, and the labels used for fields varied significantly. For instance, the Load ID field alone had 8 aliases. As a result, the ML model kept growing. It became slow and inefficient. Our team decided to accompany this model with a dynamic algorithm (e.g., a dictionary where different field labels are hard-coded). This modification improved the solution’s performance significantly and saved the client time and money.
If the client had decided to skip the AI proof of concept, they would’ve wasted seven months just to realize that their initial idea of a pure ML-based model was not the optimal solution here. With the artificial intelligence PoC, they came to this conclusion in merely two months. Since the successful completion of the AI PoC, we have built an MVP that can handle four types of documents, taking over around 25% of the manual processing load.
Taken aback by Meta’s restrictions on data usage
A client in the entertainment industry wanted to build an AI-driven analytical platform for independent artists. The solution was supposed to crawl social media, including Facebook and Instagram, to gather data. It would process all this information to gauge people’s sentiment towards the artists. Musicians could sign up for the platform and receive feedback on what social media behavior is the most beneficial for their success.
We proceeded with the AI proof of concept to test the idea. After just two weeks, we realized it was simply impossible to gather data from Facebook and Instagram and use it for the purpose described above. Typically, some of the data can be retrieved via Graph API. Combining this with a verified business account in Meta, we assumed we would gain access to the required information. However, the client couldn’t supply us with a verified business account, and the data from Graph API alone was not sufficient for this solution to work.
If the client had decided to skip the PoC, they would’ve wasted around $20,000 on the discovery project. This would include a detailed description of the solution and an estimation of the development costs. But as they chose to start with the AI PoC, they spent only around $5,000 before figuring out that this idea was impossible to execute due to data access restrictions enforced by the Meta company.
Eight steps to AI PoC with ITRex
Here are the eight steps that we will follow together to successfully go through your AI PoC.
Step 0: Identifying which issue(s) you want to tackle with AI
You can go through this step with your in-house technology team before contacting ITRex. Or we can help you identify the pressing issues your business is facing and decide whether AI technology is the best fit.
It is essential to specify what exactly you want to accomplish by implementing an artificial intelligence PoC. The selected use case needs to be of high value to your business. If you have doubts, a good place to start is to look into what others in your field are using AI solutions for.
After you’ve accumulated a list of opportunities, you can ask the following questions to determine which ones are the best fit for your project at the moment:
-
Is the problem you intend to solve specific enough? Can you evaluate the results to determine success?
-
Did you already attempt to solve this problem with other technologies?
-
Do you have the talent and the funding to support this project until the end? If there is no suitable in-house talent, can you hire an external dedicated team?
-
How will it impact your business? Is this effect significant enough to put in the effort?
-
Can you sell this idea to the executives? Is your organization ready to take on such projects?
-
Does your firm already have a data strategy? How will it align with this project?
-
What are the potential risks and limitations of using AI to tackle this problem?
Step 1: Composing a list of business objectives
Now that you’ve identified the issues you’d like to tackle with AI, it’s time to match AI implementations against specific business objectives and success metrics. For example, if you work in the manufacturing sector and want to cut equipment maintenance costs, one measurable business objective would be predicting equipment failure with 95% accuracy.
We will still dig deeper into the objectives. Our team will work closely with you to understand the intricacies of your manufacturing process (following the example above) and the types of equipment involved. We will consider factors such as the frequency and severity of past breakdowns, the cost implications of unplanned downtime, and the criticality of each piece of equipment to your operations. And based on this information, we will compose a list of SMART objectives.
Step 2: Formulating hypotheses
Our team will brainstorm several hypotheses for the solution. One hypothesis can be a combination of several artificial intelligence models. We may also suggest combining AI and with other technologies, such as robotic process automation. Our team will quickly evaluate each assumption based on data requirements, impact, and scalability to eliminate the ones with the least potential and test the remaining hypotheses during the AI PoC. Even if you turn to us with a specific AI solution in mind, our consultants will leverage their AI expertise to recommend alternative options and test them all to figure out what suits you best.
Don’t worry; having several alternatives won’t make your AI proof of concept unmanageable. We will test the hypotheses within the tight timeframe of two weeks or any other deadline that you prefer, report the findings, and help you decide what to do next. If you want to investigate further, we will continue with the proof of concept.
Step 3: Experiment design
Now, we carefully plan and structure the AI PoC. This step involves specifying the data we’ll use and setting up the experimental environment, making sure that it’s as close to the actual conditions as possible.
Preparing the data
We will help you aggregate and prepare the training data for the AI algorithms. Here are several options for data acquisition:
-
Checking which data is available for use within your company
-
Generating semi-synthetic and synthetic data using specific, ready-made applications or your own solution
-
Purchasing datasets from reliable providers
-
Using open-source data
-
Hiring people to scrape the data that will fit your purpose
Our data scientists will run the initial data screening. They will perform the following tasks:
-
Structure the data
-
Clean it by eliminating noise
-
Add any missing data points, in case of tabular dat
-
Perform feature engineering (i.e., adding and deleting data fields)
-
Apply manipulations, such as combining or filtering data
When the data is ready for usage, it is split into three sets:
-
Training set, which the model will use to learn
-
Validation set to validate the model and iterate on training
-
Testing set that will evaluate the algorithm’s performance
Setting up the environment
We carefully select the environmental components, such as:
-
Infrastructure. Setting up the computational resources needed for the AI models. This can include cloud services, on-premises servers, or a hybrid approach, depending on your infrastructure and preferences.
-
Tools and platforms. Selecting the appropriate AI tools and platforms for model development and testing. Our team can use platforms like TensorFlow, PyTorch, or proprietary solutions tailored to your needs.
-
Simulated vs. real-world settings. Deciding whether the experiments will be conducted in a controlled, simulated environment or in real-world settings. For example, testing predictive maintenance models might initially occur in a simulation before being applied to actual machinery.
Step 4: Architect and build or buy the solution
You are probably wondering whether you should build the model yourself or procure an existing solution.
Here’s when it makes sense to create an AI model from the ground up:
-
Your solution is innovative and doesn’t conform to an existing standard
-
Ready-made AI solutions addressing your business problems are costly to customize and scale
-
The closest off-the-shelf model is overkill, doing much more than you actually need
Consider procuring a ready-made model if:
-
The costs of buying,customizing, and scaling the model are less than building it from the ground up
If you decide to build the AI algorithm from scratch, it will give you more control over its accuracy. It will take longer to complete the task, but the model will be tailored to your business problem and your internal processes. You won’t need to make changes to your system to accommodate external software.
Regarding the infrastructure for algorithm training and implementation, you can rely on the cloud instead of using local resources. There are four parameters that you can consider when deciding what suits you best:
-
Security. If your data is very sensitive in terms of security, then you better keep everything on-premises.
-
Performance. If the processing load is rather heavy, opt for the cloud.
-
Costs. Evaluate what will cost you more—acquiring the resources locally or paying for the cloud usage over time.
-
Accessibility. If you will only use the solution locally, you can depend on your in-house servers. And if it needs to be accessible from different geographical locations, then it’s worth considering the cloud. You can also use a hybrid setup, with the most sensitive information being stored on premises.
The cloud and on-premises infrastructure options both have their advantages and downsides. If you are operating in the healthcare sector, consider reading our article about the pros and cons of cloud computing in healthcare. Otherwise, reach out to our AI experts to choose the best technology stack and infrastructure setup options for algorithm training.
Step 5: Conducting the AI PoC (testing the hypotheses)
During this step, we move from planning to action, conducting a series of experiments to validate the AI models we’ve hypothesized in Step 2. Our goal is to gather empirical evidence on the effectiveness of these solutions and how they align with the business objectives from Step 1.
As mentioned in Step 2, we will generate several hypotheses and test all of them. If the resources permit, we can test several hypotheses in parallel, shortening the time needed for AI PoC. Otherwise, we can use the timeboxing technique to limit the number of days allocated to each hypothesis.
Our team loads the data from Step 3 into the AI models and ensures that real-time data is accessible where needed. Then we deploy the models into the testing environment. We can use one of or both testing styles:
-
Controlled testing. Running controlled experiments to compare AI-driven processes against solutions that your company currently deploys, or any other hypothetical methods. For example, if we are testing a predictive maintenance model, we can compare the performance of the AI algorithm with the traditional approach to maintenance scheduling.
-
Real-world scenarios. Simulating real-world conditions to assess how the proposed AI models perform under practical circumstances. This might include varying operational conditions, stress testing, and scalability assessments.
Step 6: Assessing the AI proof of concept’s potential to generate value
This step is about evaluating whether the AI PoC can live up to expectations. There are several ways to perform the assessment:
-
Going back to the business objectives in Step 1 and testing the solution against them
-
Collecting data on how your system operated before the AI proof of concept deployment. This would include the time spent on a particular manual task, the number of errors, etc. Next, use the information to evaluate the impact of the PoC.
-
Comparing the solution’s performance to other products that are regarded as the benchmark for this type of problems or the industry. For instance, a benchmark for image classification-related issues would be a model that delivers accurate results on popular datasets, like ImageNet.
-
Gathering user feedback either through focus groups or online surveys to gauge the levels of satisfaction and determine what is missing
-
Conducting cost-benefit analysis to understand the financial impact of this solution on the organization
Following the assessment, ITRex will also supply you with detailed documentation on the results we achieved with the AI proof of concept, justifying every decision we made along the way.
Step 7: Deciding on what to do next
After completing and evaluating the AI PoC, you have four options to choose from:
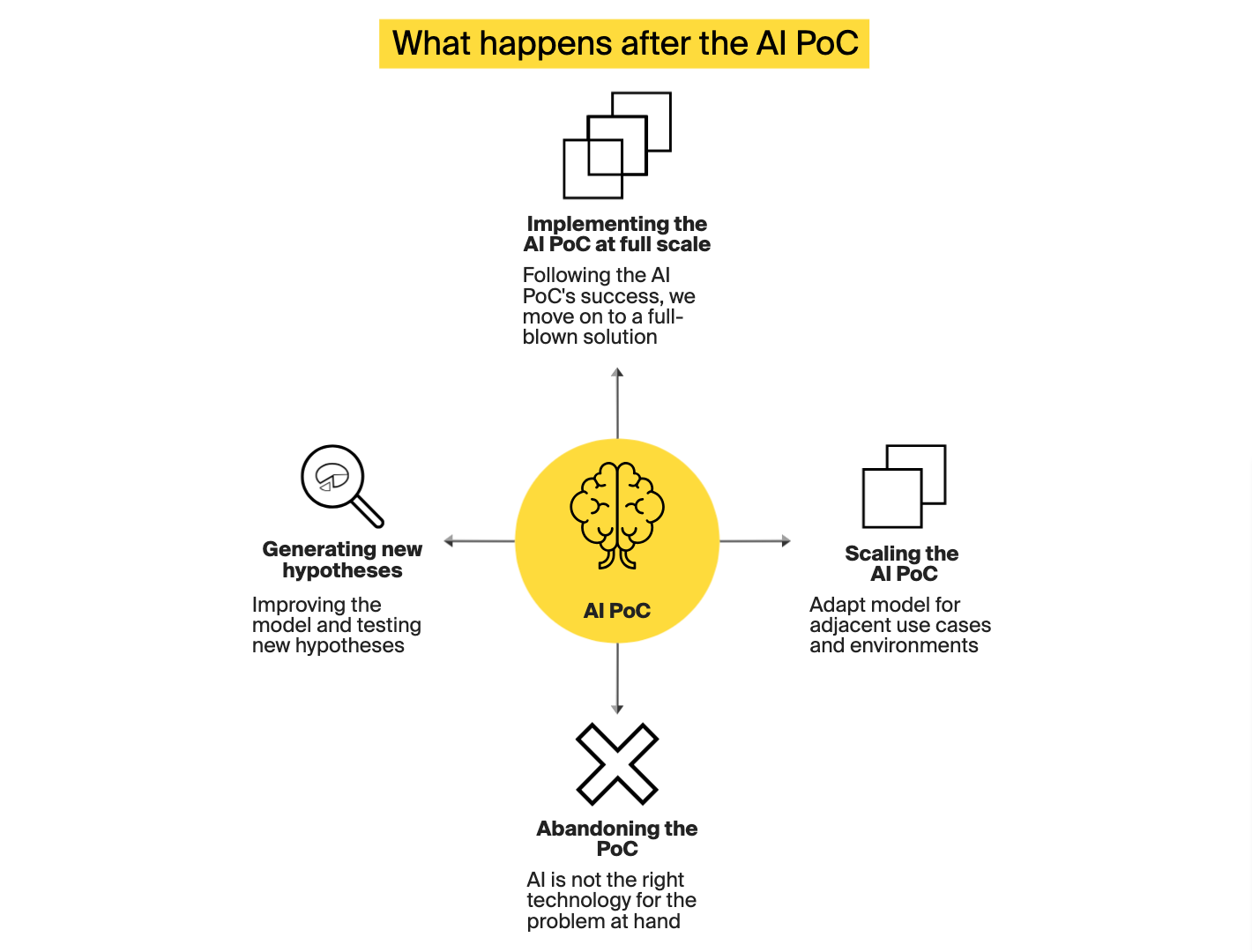
Option 1: Modifying the model and generating new hypotheses
If the results that you received in the previous step were not up to par, you might consider modifying the AI models, generating new hypotheses, and iterating the entire process—and ITRex will gladly assist you with that! We can make changes to the AI algorithms and measure their performance with each adjustment. Our team can also experiment with different hardware components or alternative cloud service models.
Option 2: Abandoning the AI PoC all together
During the AI proof of concept, you might understand that artificial intelligence is not a suitable solution to your problem and go on to explore other options.
Option 3: Scaling the AI PoC
If you are content with the AI PoC’s results, you can work on scaling it in different directions. Here are a few examples:
-
Apply the AI PoC to other business cases. Look for other applications of this new solution within your business.
-
Scale the infrastructure. Review the technology used to run this software. Can you dedicate more processing power or more data storage capacity? Such modifications will enable you to use more data, decrease latency, and maybe deliver results in real time. It will also minimize the possibility of bottlenecks in the future.
-
Optimize the AI PoC solution. Even though you already got reasonable results in the previous step, it might be worth looking for ways to improve model accuracy. You can keep training your algorithms using new data, more accurately labeled data, etc. Or you can even experiment with implementing tweaks and changes to achieve better results.
Option 4: Deploying the AI model at full scale
If you decide to adopt AI company wide following the proof of concept phase, you can find helpful tips in our guide on how to implement AI in your organization. If you want to continue with ITRex, then we move together to Step 8.
Step 8: Moving to a full-scale AI implementation
If you want to move on to a full-blown AI implementation, here is what you can expect from our team:
-
Integrating each AI model independently into your pipeline while performing integration tests
-
Implementing automation testing when possible, using different techniques, such as large language model-based testing
-
Adopting the architecture decision records (ADR) practice so that we can describe and explain every choice we make
-
Monitoring the market for any novel models and solutions that satisfy your business objectives and can surpass the AI models we currently deploy
Why partner with ITRex on AI PoC?
AI PoC allows companies to test the viability of AI solutions quickly with minimal risks and investments. If you are currently searching for a reliable partner to assist you with AI proof of concept, look no further. Here are the perks that you will get if you partner with ITRex:
-
We limit the duration of AI proof of concept. We prefer to stick to a two-week deadline and report our findings. If the client wants to investigate additional solutions, we can expand this timeframe.
-
We always document every choice we make along the course of the AI PoC. You will receive an extensive document describing every step and decision. You can submit this document to investors, clients, developers, etc.
-
Even if you turn to us with a specific AI model in mind, we will draw on our expertise to suggest any alternatives that we believe are better suited to your business needs
-
ITRex takes your budget into account. We will look for a tradeoff between costs, speed, and efficiency.
-
We are driven by your unique business needs, not by the desire to implement a specific technology
Get in touch and let your journey to AI adoption begin!