Understanding Gen AI in life sciences
Generative AI is a subtype of artificial intelligence. Unlike classic AI, Gen AI can not only analyze data but also produce content that resembles human creation. Check out our blog for more information on how Gen AI differs from traditional artificial intelligence and generative AI applications in business.
Life sciences generative AI has three major advantages over classic AI. The technology can:
-
Generate novel content, such as structured legal documents, medical images, patient medical history datasets, and more
-
Search and analyze enormous amounts of unstructured data rather fast, something that classic AI struggles with
-
Imitate human conversation, engaging in personalized chats with patients and answering research queries
With all this potential, the global market for generative AI in life sciences is expanding. It is valued at $233.74 million in 2024 and is expected to reach $1544.13 million by 2034, growing at a CAGR of 20.78% during the forecast period.
Let’s see how Gen AI can transform the life sciences sector.
Top generative AI use cases in life sciences
Deloitte reports that generative AI can unlock $5–7 billion in value for the life sciences sector. The consultancy argues that 90% of this sum will come from three functions—clinical research, manufacturing and supply chain, and commercial.
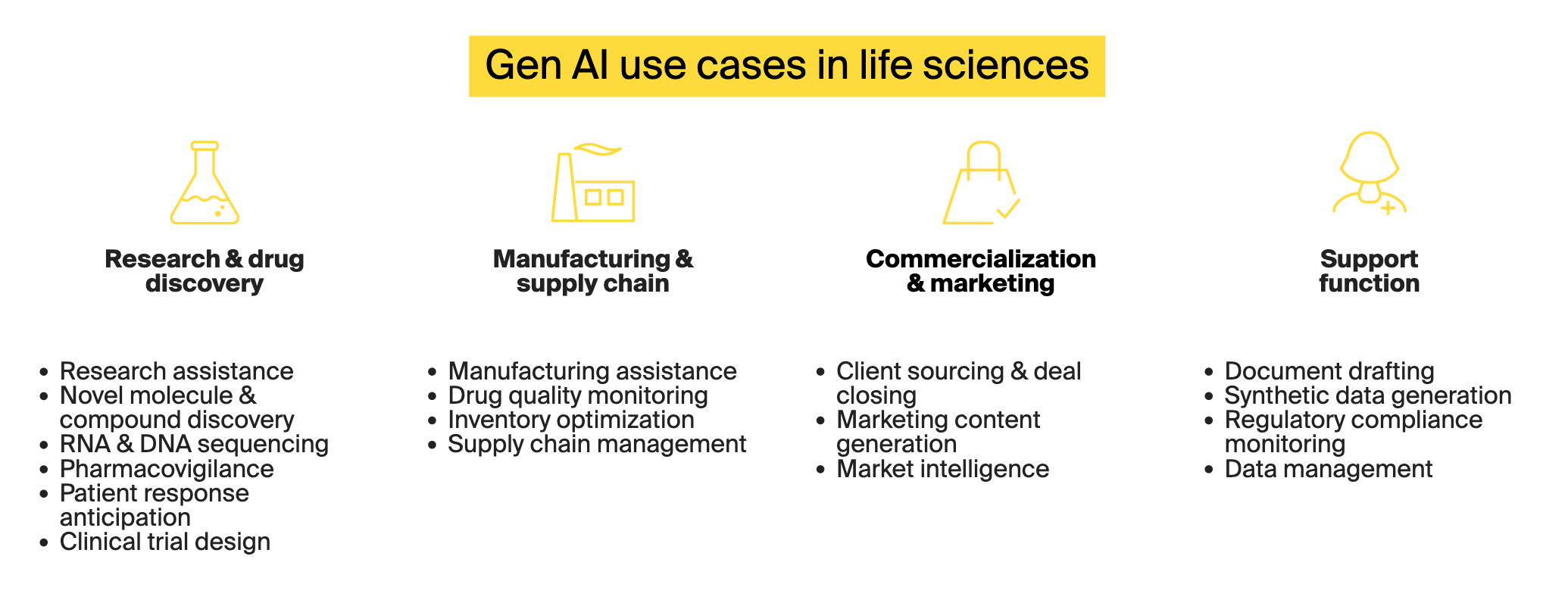
Research and drug discovery
Bringing a new medication to market costs around $2.6 billion and takes up to ten years. No surprise that biotech companies are looking to expedite the process. Gen AI might be the answer. It can summarize scientific literature, generate and refine novel compounds, simulate a candidate drug’s behavior in different patients, and much more. Here is what the full scope of Gen AI applications in this domain looks like.
-
Research assistance. During the preliminary research stage, scientists have to extract information from medical publications, trial data, patent reports, and more. If they do it manually, they can end up with incomplete information. When combining generative AI and life sciences, researchers can outsource browsing scientific literature to specialized large language models (LLMs), such as Google’s Med-PaLM and BioGPT, that can answer research queries.
Gen AI models can also summarize scientific literature, extracting valuable insights and saving researchers’ time on reading. For example, with Gen AI, Pfizer could save its researchers 16,000 hours of search time per year.
-
Novel molecules and compounds discovery. Foundation models can learn the principles of molecular chemistry and generate novel small and large molecules with desired properties and low toxicity. They can also predict the molecule’s binding affinity with the target and optimize these molecules when needed.
-
RNA and DNA sequencing. Researchers can rely on generative AI in life sciences to analyze and manage the vast data produced by DNA and RNA sequences. Gen AI models can interpret this data, identify patterns, and even discover novel genes and determine their function. The technology can also help eliminate noise in single-cell RNA sequencing, a complex technique that captures gene expressions of individual cells.
By scanning genomic data, Gen AI models can identify similarities in molecule structures of different diseases and suggest existing drugs that can be repurposed to treat other disorders.
-
Pharmacovigilance. Generative AI can simulate molecule interactions to predict possible adverse drug reactions (ADR) and their likelihood. ADRs are not only expensive but also cause mortality and hospitalization. Estimates show that ADRs cost the US healthcare system $76–152 billion annually.
Gen AI can look into genomic and proteomic data to learn how different genetic makeups will respond to a candidate drug. These models can also analyze drug-protein interactions to predict if the drug will bind to non-target proteins, resulting in toxicity.
-
Patient response anticipation. Generative AI in life sciences can help predict patient response to the proposed treatment. One approach is to use virtual patients—digital twins of real patients. A virtual patient has a detailed health profile identical to a real person, and Gen AI can simulate a digital twin’s interaction with novel drugs and capture its response.
-
Clinical trial design. Gen AI can analyze existing clinical trial documentation and recommend various trial aspects, such as protocols, patient enrollment modes, and geographical location, among others. Gen AI can produce trial strategies and simulate different scenarios to compare their outcomes. Further into the trial, these models can suggest adaptations to the strategy based on intermediate results.
Check out our blog for more information about generative AI benefits in drug discovery and the use of artificial intelligence in clinical trials.
Real-life examples
The Hong Kong-based biotech startup Insilico Medicine is a leader in AI-powered drug discovery. The company’s INS018_055 is the first AI-generated drug to enter clinical trials and be administered to human patients. This drug was designed to treat idiopathic pulmonary fibrosis, which causes lung scarring. What’s really captivating is that the startup spent one-tenth of the funding that is typically allocated to a drug discovery process using the traditional approach. Insilico Medicine has already developed five successful compounds with the help of Gen AI.
Another example is Chat Nucleotide Transformer (ChaNT), a Gen AI model from InstaDeep and BioTech, that aids researchers in gene understanding. The model studies gene sequences and answers specific questions. It functions like ChatGPT but for genomics; researchers show ChatNT a DNA sequence and inquire how the behavior will change given specific modifications.
Manufacturing and supply chain
Another Gen AI use case in life sciences is facilitating drug manufacturing and raw material supply. Gen AI can assist you at every stage of the process, from acting as a manufacturing assistant to negotiating with prospective suppliers.
-
Manufacturing assistance. Generative AI in life sciences can support factory managers by sourcing manufacturing standards, generating checklists for production, and monitoring the factory floor for abnormalities, such as leaks of toxic substances. If the factory is equipped with IoT devices, Gen AI can aggregate and analyze sensor readings to monitor machine performance, detect anomalies, and notify appropriate managers. It can also optimize maintenance schedules when needed.
-
Quality monitoring. Gen AI models can inspect the drug manufacturing process in real time with the help of computer vision. Algorithms can catch on changes in key parameters, such as temperature, pressure, and mixing time. They can also monitor batch consistency and detect any variations in pill size, shape, and surface. Upon spotting anomalies, generative AI for life sciences can issue a warning and suggest real-time adjustments to maintain the drug’s chemical composition and consistency.
-
Inventory optimization. Gen AI models can predict demand for specific drugs based on purchase orders and pharmaceutical market trends, preventing product and raw material shortages. This allows life science companies to proactively ramp up production and restock on raw materials, avoiding disruptions in product supply. Besides demand-driven stocking, generative AI in life sciences can also track expiration dates and produce optimal utilization plans for drugs and compounds.
-
Supply chain management. Generative AI has many benefits for the supply chain. A Gen AI-powered assistant can help you select the right partner. It can analyze supplier performance, costs, reliability, and other data to identify the most suitable candidates. Gen AI can draft contracts and assist in negotiations. But its role doesn’t end there. It can help manage risks during transportation by analyzing traffic, geopolitical issues, and other factors that can cause delays and assist biotech companies in developing backup plans.
You can learn more about how artificial intelligence facilitates manufacturing on our blog.
Real-life example:
Sanofi, a large pharmaceutical company with a global presence, launched its company-wide AI system, Plai, in mid-2023. Later, the company integrated Gen AI to streamline its operations. Sanofi employs this AI-powered platform in different functions, including inventory management, where Gen AI is responsible for optimizing raw material utilization in drug manufacturing to avoid spoilage, handling quality assessment processes, and more. With this platform, Sanofi was able to predict 80% of low inventory positions and restock before it affected sales and manufacturing.
Commercialization and marketing
Life science firms can also rely on generative AI for drug marketing and selling. Here’s what the technology can do in this domain.
-
Client acquisition and deal closure. Gen AI-powered sales assistants can craft customized pitches and support the human sales force by offering the right piece of information at the right time. When you find a prospective buyer, Gen AI models can help you prepare for deal negotiation, model negotiation scenarios, and draft contracts.
-
Marketing content generation. Employing generative AI in life sciences will enable you to move some of your marketing capabilities in-house instead of relying on advertising agencies. AI-driven marketing assistants can generate campaign ideas and assemble customized marketing material that is both informative and compliant with pharma regulations. AI-driven chatbots can respond to customers’ questions 24/7 with a personalized conversation.
Additionally, Gen AI can scrutinize marketing content for inconsistencies and high-risk claims and flag them for additional review.
-
Market surveillance. Generative AI can be useful for life sciences companies even after the novel drug is released to the public. Algorithms can monitor social media and pharma websites for customer feedback on the drug to spot any side effects and flag them for investigation. Gen AI is also useful in competitor intelligence, analyzing your rivals’ campaigns and assessing the performance of similar medicines.
Real-life example:
Pfizer collaborated with a French advertising and public relations company, Publicis Groupe, to develop a Gen AI-drive platform for pharma marketing called “Charlie.” This platform can generate marketing content, fact-check and edit existing copies, and ensure the material abides by the strict industry regulations. Marketing professionals can also use Charlie to understand customer behavior and expectations, craft personalized campaigns, and create medical articles that are easy to read.
Support function
Besides the core functionality, generative AI in life sciences has applications in support areas. For instance, the technology can help handle regulatory submissions and requests, produce synthetic training data, and more. Scroll down to learn more about these non-obvious use cases.
-
Document drafting. During the drug discovery process, medical writers need to submit legal documents to the FDA and other regulatory organizations. These documents are thousands of pages long and rely on datasets that need to be processed beforehand. Generative AI in life sciences can analyze these massive volumes of information and produce first drafts that human writers can review and submit. Moreover, Gen AI models can identify any inconsistencies in research findings and notify the responsible personnel.
Besides regulatory submissions, Gen AI can create clinical reports and any other documentation.
-
Synthetic data generation. It’s typically challenging for clinical researchers to obtain sufficient data for clinical trials, especially when it involves rare diseases. Gen AI in life sciences can generate synthetic patient data that resembles the real world.
-
HAQ automation. While a drug is being developed, regulatory agencies can contact life science firms with health authority queries (HAQ), which are questions about this drug as a part of the approval process. If answered manually, such requests can be a bottleneck in the development process. Gen AI-driven intelligence engines can draft answers based on drug information and successful HAQ response patterns. Research shows that such tactics can lead to 50% fewer HAQ follow-ups.
-
Data management. Clinical trials generate vast amounts of data, which includes incoming patient information, doctors’ notes, and more. Generative AI, in combination with other analytical tools, can help automate data capture and classify the information, making it easily accessible for clinical researchers.
Real-life examples:
California-based AI company Narrativa built a Gen AI-powered content automation platform that helps medical writers produce regulatory documents supporting clinical trials. As a first step, the models will analyze medical data, looking for inconsistencies. When Gen AI familiarizes itself with the data, researchers can ask questions about the datasets, and the model will respond. When all the data has been aggregated and cleaned, the Gen AI platform will create regulatory drafts that human writers can validate and improve by providing feedback. The model can then produce a second iteration based on the feedback it has received.
In another example, PwC teamed up with Amazon to combine its own life sciences experience and the capabilities of AWS. Together, they implemented a Gen AI-powered solution that will support clinical trial research and administration. This tool could draft technical source material, patient experience summaries, and other types of trial reports. PwC claims that clients who used this platform experienced a $2–10 million cost reduction per trial and cut the time spent on trial reports by 90%.
How to approach generative AI implementation in life sciences
If you are considering embarking on the Gen AI adoption journey, here are some important factors to consider.
Choosing between off-the-shelf vs. customized Gen AI models
If you want to deploy generative AI in life sciences, you have two choices—find a suitable ready-made model and use it as is or retrain this model on your data to customize it to your needs. A fine-tuned model will be more accurate and easier to integrate into your workflows, but it will also be more expensive. For example, customizing a moderately large open-source LLM, like GPT-2, will cost you anywhere from $80,000 to $190,000, including implementation, hardware, and data preparation expenses. This estimation doesn’t account for the licensing fees companies will have to pay if they choose a commercial solution.
McKinsey reports that life science companies that invested in customizing their generative AI models witness double the impact. So, such an investment seems worthwhile.
You can find more information in our detailed guide on generative AI costs.
Crafting your enterprise data strategy
Gen AI models are fueled with vast amounts of data. So, before diving into Gen AI implementation or building any AI system, devise a comprehensive enterprise data strategy.
Data strategy addresses the technical aspects of data storage, security, and accessibility. Because much of the data in life sciences involves sensitive information, such as patient health records or proprietary research, strong data governance practices are essential to protect privacy and comply with regulations like the Health Insurance Portability and Accountability Act (HIPAA) and the General Data Protection Regulation (GDPR). By ensuring data is both accessible and securely managed, companies can maximize the potential of generative AI while minimizing risks associated with data breaches or regulatory non-compliance.
Considering possible model hallucinations
Generative AI algorithms, while powerful, sometimes produce plausible yet incorrect results, known as “hallucinations.” In life sciences, this can lead to models generating protein structures that are impossible to create in real life, providing inaccurate information as research assistants, and other critical errors.
At the moment, it seems impossible to fully overcome this problem. Even if you invest heavily in customizing a Gen AI solution, it can still hallucinate. What you can do is implement checkpoints that will verify AI’s output. Resort to the human in the loop approach to ensure fact-checking. For example, don’t let Gen AI answer patient’s questions directly without any prior reviews.
Addressing ethical considerations and possible bias
In life sciences, generative AI models’ performance hinges on the quality and diversity of the training data. For instance, in drug discovery, an AI model trained on a dataset with limited chemical diversity will continue to produce similar molecule structures, potentially overlooking promising compounds in underrepresented chemical spaces. This lack of diversity can limit the innovation potential.
Ethical and regulatory considerations further complicate the use of generative AI in life sciences. Biotech companies work with sensitive personal data, including genomic sequences and medical histories, making compliance with privacy regulations critical. Ensuring informed consent and safeguarding data privacy are all essential when training Gen AI on such information. Additionally, the issue of intellectual property arises when firms use generative AI models in drug development. Companies must decide how to manage intellectual property rights for novel compounds discovered through AI, especially when the AI models themselves are fine-tuned by a third party.
Selecting a reliable Gen AI partner
Life science companies embarking on generative AI projects need a reliable, expert partner to navigate the complexities of this technology. Implementing generative AI in domains like drug discovery, manufacturing, and marketing demands not only technical proficiency but also a deep understanding of data integrity and regulatory compliance. The right generative AI development partner offers end-to-end support, monitors for potential bias, validates model outputs, and more. This gives you the opportunity to focus on innovation without being drowned in technicalities and data privacy violation fees.
Here at ITRex, we are well-versed in life science software development. We have capable data consultants and experience in cutting-edge technologies, such as AI, computer vision, and IoT. ITRex can be your one-stop shop for deploying and scaling generative AI.
To conclude
Still hesitant to start with Gen AI deployment in life sciences?
Deloitte suggests following the “no regrets bet” approach and starting with low complexity and high value business cases, such as summarizing medical publications, drafting trial documentation, and gathering competitive market intelligence.
At ITRex, we offer an AI proof of concept (PoC) development service, enabling our clients to experiment with the technology without investing the resources in a full-fledged project. You can find more about AI PoC on our blog. So, select your “no regrets bet” case study and test it with Gen AI through our PoC.
Already implemented your first Gen AI project and want to scale?
Research shows that life science firms are eager to experiment with generative AI, but the scale of adoption remains limited. A recent study by McKinsey reports that 74% of the surveyed leaders have implemented five or fewer Gen AI use cases.
You can start with AI PoC to test the technology on one use case. But when the first step is taken, look beyond individual use cases into how Gen AI can support your enterprise-wide transformation. Invest in the building blocks of the generative AI value chain to have a solid foundation for your initiatives. You can put together several use cases for incremental value and combine them with other cutting-edge technology, such as machine learning and IoT.