Why do traditional approaches to omics data analytics fall short?
The concise response is that omics data possesses unique characteristics that are specific to large, multi-dimensional datasets. These characteristics render traditional data analytics techniques ineffective. But first, let us define omics data and then discuss the associated challenges.
What is omics data, and what does it include?
Omics data is the information generated by modern technology as it analyzes biological specimens. Omics gives us a detailed view of life at the molecular level. Such data is typically generated by disciplines ending with the suffix -omics, such as:
-
Genomics is the study of an organism’s entire genome
-
Transcriptomics focuses on RNA transcripts and reveals which genes are being actively expressed in different tissues or under specific conditions
-
Proteomics explores the peptides and proteins within an organism, helping researchers understand biological processes and signaling pathways
-
Metabolomics examines small molecules (metabolites) produced during metabolism to determine an organism’s metabolic state and responses
-
Epigenomics investigates DNA and histone modifications that control gene expression without affecting the underlying code
-
Microbiomics studies the community of microorganisms that live in and on the human body, including the gut microbiome
-
Lipidomics, as the name implies, concentrates on the study of lipids—fats and their derivatives—that play critical roles in energy storage, cell signaling, and membrane structure
-
Glycomics studies the intricate sugar chains that are attached to proteins and lipids and are essential for cell communication, immune response, and structural integrity
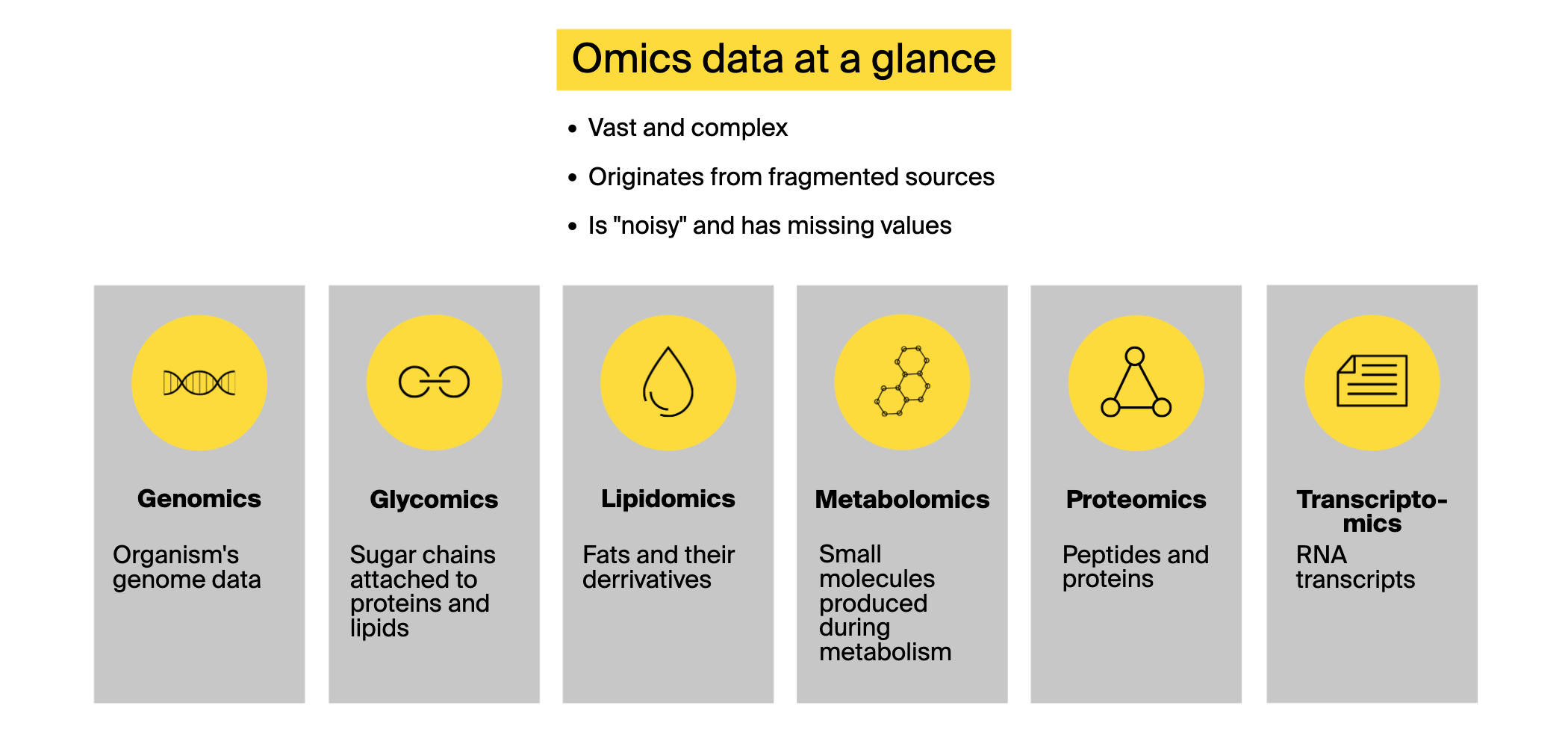
The importance and complexity of omics data analysis
Omics data is vast and complex, but it holds enormous potential. By analyzing omics data, researchers and clinicians can uncover disease biomarkers, predict patient responses to therapies, design personalized treatment plans, and more.
Omics data is specifically useful when taking the multi-omics approach, combining several data streams. Most prevalent diseases, such as Alzheimer and cancer, are multifactorial, and analyzing one type of omics data will have limited therapeutic or predictive effect. This makes multi-omics data management an essential capability for researchers, but it complicates the analysis.
Here is why it’s challenging to handle omics data with traditional analytical tools.
Challenges that omics data analysis software can face
There are several characteristics that prevent traditional analytics methods from effectively dealing with omics data, let alone multi-omics approaches:
-
Data complexity and volume. Omics datasets, such as those from genomics or proteomics, often contain millions of data points for a single sample. Traditional methods struggle to handle this vast feature space, leading to computational bottlenecks.
-
Fragmented data sources. Omics data comes from diverse platforms, experiments, and repositories. There are varying data formats, standards, and annotations used by different research groups or institutions. Integrating these data formats into a cohesive analysis framework can be daunting for traditional approaches.
-
Noise and missing data. Biological experiments generate inherently noisy data, which is exacerbated by technical errors and missing values. Traditional analytics tools lack robust mechanisms to deal with these imperfections, leading to biased or inaccurate results.
-
Complexity in biological interpretation. Traditional analytics often identify statistical correlations or patterns within omics datasets but fail to translate them into actionable biological insights. For example, to determine the role of a specific gene variant in a disease pathway, the tool must combine data with existing biological knowledge, such as gene expression profiles and protein interactions. Traditional omics data analysis tools typically lack the sophistication required to perform such analyses.
How AI could solve key omics data analytics challenges
Artificial intelligence and its subtypes have an immense influence on the pharma and bioinformatics fields. We prepared a list of insightful articles on the topic:
Let’s discover how the leading-edge technology can streamline omics data analysis.
Handling high dimensionality
Omics datasets frequently contain millions of features, which overwhelms traditional analytical methods and makes it difficult to determine which variables are relevant.
AI excels in managing such large datasets by automatically identifying the variables that matter most while ignoring irrelevant or redundant information by applying techniques like feature reduction. AI simplifies omics data analysis by focusing on the most significant patterns and connections, helping researchers uncover key insights without getting lost in the data’s complexity.
Integrating heterogeneous data
The diverse data generated by omics fields, such as genomics, proteomics, and metabolomics, are challenging to integrate cohesively.
AI models can standardize data that comes in different formats, like genomic sequences and clinical records, and normalize it to ensure consistency. The data is then processed by AI algorithms to reveal cross-dataset relationships, demonstrating how variations in one omics layer influence another.
For example, AI tools can combine genomic data, such as gene mutations, with proteomic data, such as protein expression levels, to better understand cancer. By linking these two data types, AI can help identify how genetic changes in tumor cells lead to alterations in protein behavior, explaining how cancer develops and suggesting new targets for treatment.
Addressing noise and missing information
Noisy data and missing values can skew traditional analysis methods.
To overcome these obstacles, AI uses advanced algorithms like imputation and noise reduction. AI-based omics data analytics software identifies patterns in complete datasets to estimate missing values with high accuracy. For instance, if a certain gene’s expression is unrecorded, AI might predict its value based on similar genes or patterns in the surrounding data. Techniques like generative adversarial networks (GANs) can synthesise realistic data points to fill the gaps. AI tools can also filter out irrelevant or noisy signals, such as outliers and random fluctuations.
To give an example, a Korean research team proposed a novel AI-powered tool that uses padding to work with incomplete omics datasets and correctly identify cancer types. This tool has two parts—a Gen AI model that can learn tumor genetic patterns and apply padding to substitute missing data points with virtual values and a classification model that analyzes omics data and predicts cancer type. The researchers tested this tool and reported that it effectively classifies cancer phenotypes, even when working with incomplete datasets.
Enhancing accuracy and efficiency
Traditional workflows heavily rely on people, which makes them error-prone, time-consuming, and inefficient for large-scale analyses.
AI transforms the process by automating critical tasks and improving accuracy. Instead of manually preprocessing, filtering, analyzing, and interpreting massive datasets, AI tools can do so automatically and with far greater precision. For example, AI can quickly scan thousands of genes, proteins, or metabolites to pinpoint the ones that are most relevant to a specific disease. It can also detect anomalies, such as unusual patterns and outliers, and flag these inconsistencies, preventing bias in analytics insights.
Clinical studies support the idea that artificial intelligence can be more accurate in detecting cancer than human doctors. A recent experiment shows that Unfold AI—clinical software built by Avenda Health and cleared by the FDA—could identify prostate cancer from various clinical datasets with the accuracy of 84%, while human doctors could only achieve 67% accuracy working on the same data.
There are even autonomous AI agents that take care of multi-omics data analysis with minimal human intervention. Automated Bioinformatics Analysis (AutoBA) is one such example. This AI agent uses large language models (LLMs) to plan and perform omics data analyses. The user’s input is limited to entering the data path, description, and the final goal of the computation. AutoBA then designs the process based on the datasets provided, generates code, runs it, and displays the results.
Improving interpretability and decision-making
Traditional data analysis techniques, as well as many AI models, often function as ‘black boxes,’ delivering results that are challenging to interpret or explain. Researchers see the recommendations or predictions but do not understand why the system made that decision.
AI can resolve this through explainable AI (XAI) techniques, which make complex results more transparent and easier to understand, demonstrating how the model arrives at its conclusions. For example, AI can highlight which genes, proteins, or other factors were most influential in predicting a disease or classifying samples. Visual tools, such as heatmaps, feature rankings, or network diagrams, can help researchers clearly see the relationships and reasoning behind the model’s output.
One example of an explainable AI omics data analysis tool is AutoXAI4Omics. This open-source software performs regression and classification tasks. It can preprocess data and select the optimal set of features and the best-suited machine learning model. AutoXAI4Omics explains its decisions by displaying connections between omics data features and the target under analysis.
Things to consider when implementing AI for omics data analysis
To successfully implement AI-powered omics data analysis, consider the following factors before beginning implementation.
Data quality
AI algorithms thrive on high-quality data, and in omics, insights are only as accurate as the datasets. After aggregating the data using either manual or automated data collection, preprocess the dataset so that it’s suitable for AI consumption.
For multi-omics data analysis, you will combine various data sources, such as genomics, proteomics, and metabolomics, which will necessitate resolving disparities in data formats and standards. If you haven’t done this yet, it’s time to invest in robust data governance practices.
At ITRex, we have experienced data consultants who will help you craft an effective enterprise data strategy and establish a solid data management framework to support your AI initiatives. We can also assist you with data storage and consult you on data warehouse options.
Ethics and regulatory compliance
Omics data often contains sensitive information that is protected by law as it can be used to uncover identities. For example, protein expression levels in blood plasma are enough to identify individuals in certain cases. When you add AI to this mix, privacy concerns escalate even further. Research demonstrates that during the model training phase it’s possible to infer patient identity. Even after the training is over, there is still potential for hackers to attack the model and extract private information.
To conform with ethical standards, obtain informed consent from study participants and ensure that AI algorithms don’t perpetuate biases or unfair practices.
If you partner with ITRex, we will ensure transparent data handling and clear process documentation to build trust with all the parties involved. We will help you deploy explainable AI so that researchers can understand how the algorithms came up with recommendations and verify their correctness. We will also check your AI system for security vulnerabilities. And of course, our team adheres to regulatory frameworks like the General Data Protection Regulation (GDPR), the Healthcare Insurance Portability and Accountability Act (HIPAA), and other relevant local regulations to safeguard data privacy and security.
Infrastructure and scalability
Processing omics data requires significant computational power and storage capacity, making infrastructure a key consideration. Cloud-based solutions offer scalability and flexibility, enabling teams to handle large datasets and run computationally intensive AI models. On-premises infrastructure gives you full control over your data and algorithms but demands a considerable upfront investment. A hybrid approach allows you to mix both options.
Cloud-based | On-premises | Hybrid | |
---|---|---|---|
Description |
Using remote servers hosted on platforms like AWS, Azure, or Google Cloud to store and process data |
Deploying and maintaining hardware and software within your organization’s facilities |
Combining on-premises infrastructure with cloud-based resources |
Characteristics |
+ Processing massive datasets and scaling computational resources up or down on demand – Security issues arise as you upload the data to third-party systems |
+ Giving you control over data and infrastructure – High upfront investment in hardware acquisition and maintenance |
+ Enhancing security as sensitive data remains on your premises – Integration complexities as you try to ensure a seamless workflow between on-premises and cloud-based tools |
Scalability also involves designing workflows that can adapt to increasing data volumes and evolving analytical requirements. One example is using containerization—packaging an application and all its dependencies into one container—and orchestration tools, like Docker and Kubernetes, to manage deployment and scaling of these containers.
If you decide to collaborate with ITRex, we will help you choose between the different deployment approaches, considering factors like data security requirements, latency, and long-term cost efficiency. Our team will also advise you on containerization and orchestration options.
Operational costs
Implementing an AI system for omics data analysis involves both upfront and ongoing costs. Organizations need to budget for the following expenses:
-
Acquiring high-quality data and pre-processing it
-
Providing data storage
-
Building or licensing AI models
-
Computational resources and power consumption
-
Maintaining the required infrastructure or paying usage fees to a cloud provider
-
Training your staff
Cloud services, while seeming like a cheaper option, may lead to unexpected costs if not managed carefully. The same applies to ready-made commercial AI algorithms. While developing an AI mode from the ground up requires a larger upfront investment, licensing fees for off-the-shelf tools can quickly accumulate and increase, particularly as your operations scale.
To give you a more detailed overview of the pricing options, our analysts compiled comprehensive guides on the costs associated with artificial intelligence, generative AI, machine learning, and data analytics solution implementation.
A reliable AI consulting company like ITRex can reduce costs by recommending cost-effective, open-source tools when possible to lower licensing expenses. Our expertise in compliance and data usage regulations will help you avoid penalties and reduce the complexity of meeting regulatory requirements. We can also provide cost-benefit analyses to align AI investments with measurable ROI. Overall, ITRex ensures that you implement cutting-edge solutions in a cost-efficient and sustainable manner.
Talent and expertise
Successfully deploying AI in omics data analysis requires a multidisciplinary team with expertise in bioinformatics, healthcare, and machine learning. You will need skilled professionals to design, build, train, and validate AI models. Research shows that talent shortage remains a significant barrier to AI adoption. A recent survey revealed that 63% of the responding managers can’t rely on their in-house staff for AI and ML tasks. Moreover, with the rapid pace of AI advancements, continuous training and upskilling are essential for keeping AI teams competent.
If you team up with ITRex, you will have access to a pool of skilled AI developers with experience in healthcare and other related fields. You can either outsource your AI projects to us or hire a dedicated team of experts to strengthen your internal staff.
To sum it up
In the rapidly evolving world of omics data analysis, harnessing the power of AI is a necessity for staying ahead in biotechnology and pharmaceutical research.
ITRex can be your trusted data science partner that will help you navigate this complex landscape, offering tailored AI solutions that simplify analysis, enhance accuracy, and ensure regulatory compliance. If you aren’t confident whether AI can effectively address your needs, we offer an AI proof-of-concept (PoC) service that allows you to experiment with the technology and test your hypothesis on a smaller scale without investing in a full-blown project. You can find more information on AI PoC on our blog.